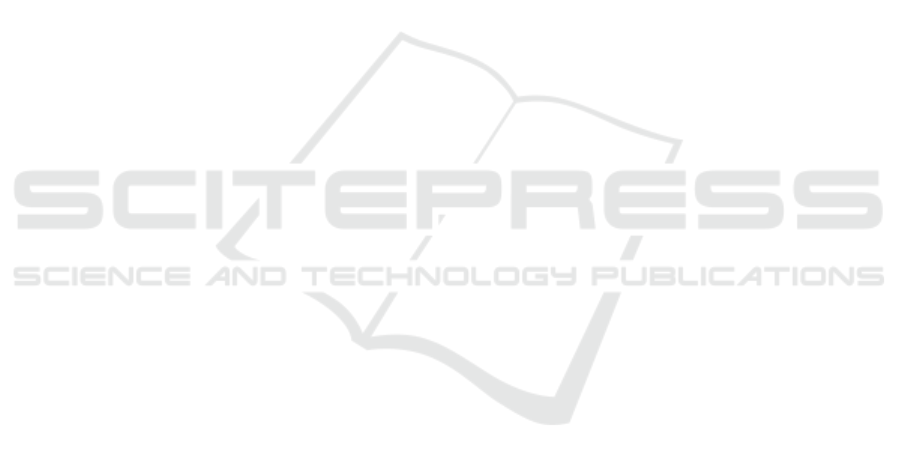
Arora, K., Asri, L. E., Bahuleyan, H., and Cheung, J. C. K.
(2022). Why exposure bias matters: An imitation
learning perspective of error accumulation in language
generation. arXiv preprint arXiv:2204.01171.
Choi, E., Bahadori, M. T., Schuetz, A., Stewart, W. F., and
Sun, J. (2016a). Doctor ai: Predicting clinical events
via recurrent neural networks. In Machine learning
for healthcare conference, pages 301–318.
Choi, E., Bahadori, M. T., Sun, J., Kulas, J., Schuetz, A.,
and Stewart, W. (2016b). Retain: An interpretable
predictive model for healthcare using reverse time at-
tention mechanism. Advances in neural information
processing systems, 29.
Devlin, J., Chang, M.-W., Lee, K., and Toutanova, K.
(2018). Bert: Pre-training of deep bidirectional trans-
formers for language understanding. arXiv preprint
arXiv:1810.04805.
Edin, J., Junge, A., Havtorn, J. D., Borgholt, L., Maistro,
M., Ruotsalo, T., and Maaløe, L. (2023). Automated
medical coding on mimic-iii and mimic-iv: A crit-
ical review and replicability study. In Proceedings
of the 46th International ACM SIGIR Conference on
Research and Development in Information Retrieval,
pages 2572–2582.
Egger, J., Gsaxner, C., Pepe, A., Pomykala, K. L., Jonske,
F., Kurz, M., Li, J., and Kleesiek, J. (2022). Medical
deep learning—a systematic meta-review. Computer
methods and programs in biomedicine, 221:106874.
Hosseini, M., Munia, M., and Khan, L. (2023). BERT
has more to offer: BERT layers combination yields
better sentence embeddings. In Bouamor, H., Pino,
J., and Bali, K., editors, Findings of the Association
for Computational Linguistics: EMNLP 2023, pages
15419–15431, Singapore. Association for Computa-
tional Linguistics.
Johnson, A., Bulgarelli, L., Pollard, T., Horng, S.,
Celi, L. A., and Mark, R. (2020). Mimic-iv.
PhysioNet. Available online at: https://physionet.
org/content/mimiciv/1.0/(accessed August 23, 2021),
pages 49–55.
Johnson, A. E., Pollard, T. J., Shen, L., Lehman, L.-w. H.,
Feng, M., Ghassemi, M., Moody, B., Szolovits, P.,
Anthony Celi, L., and Mark, R. G. (2016). Mimic-
iii, a freely accessible critical care database. Scientific
data, 3(1):1–9.
Lima, R. (2023). Hawkes processes modeling, inference,
and control: An overview. SIAM Review, 65(2):331–
374.
Longato, E., Morieri, M. L., Sparacino, G., Di Camillo, B.,
Cattelan, A., Menzo, S. L., Trevenzoli, M., Vianello,
A., Guarnieri, G., Lionello, F., et al. (2022). Time-
series analysis of multidimensional clinical-laboratory
data by dynamic bayesian networks reveals trajecto-
ries of covid-19 outcomes. Computer Methods and
Programs in Biomedicine, 221:106873.
Mall, P. K., Singh, P. K., Srivastav, S., Narayan, V., Pa-
przycki, M., Jaworska, T., and Ganzha, M. (2023).
A comprehensive review of deep neural networks
for medical image processing: Recent developments
and future opportunities. Healthcare Analytics, page
100216.
Miotto, R., Li, L., Kidd, B. A., and Dudley, J. T. (2016).
Deep patient: an unsupervised representation to pre-
dict the future of patients from the electronic health
records. Scientific reports, 6(1):1–10.
Pham, T., Tran, T., Phung, D., and Venkatesh, S.
(2017). Predicting healthcare trajectories from med-
ical records: A deep learning approach. Journal of
biomedical informatics, 69:218–229.
Portes, J., Trott, A., Havens, S., King, D., Venigalla, A.,
Nadeem, M., Sardana, N., Khudia, D., and Frankle,
J. (2024). Mosaicbert: A bidirectional encoder opti-
mized for fast pretraining. Advances in Neural Infor-
mation Processing Systems, 36.
Rodrigues-Jr, J. F., Gutierrez, M. A., Spadon, G., Brandoli,
B., and Amer-Yahia, S. (2021). Lig-doctor: Efficient
patient trajectory prediction using bidirectional mini-
mal gated-recurrent networks. Information Sciences,
545:813–827.
Romanov, A. and Shivade, C. (2018). Lessons from natural
language inference in the clinical domain. In Riloff,
E., Chiang, D., Hockenmaier, J., and Tsujii, J., edi-
tors, Proceedings of the 2018 Conference on Empiri-
cal Methods in Natural Language Processing, pages
1586–1596, Brussels, Belgium. Association for Com-
putational Linguistics.
Saad, M. M., O’Reilly, R., and Rehmani, M. H. (2024). A
survey on training challenges in generative adversar-
ial networks for biomedical image analysis. Artificial
Intelligence Review, 57(2):19.
Severson, K. A., Chahine, L. M., Smolensky, L., Ng, K.,
Hu, J., and Ghosh, S. (2020). Personalized input-
output hidden markov models for disease progression
modeling. In Machine learning for healthcare confer-
ence, pages 309–330. PMLR.
Shankar, V., Yousefi, E., Manashty, A., Blair, D., and Tee-
gapuram, D. (2023). Clinical-gan: Trajectory fore-
casting of clinical events using transformer and gen-
erative adversarial networks. Artificial Intelligence in
Medicine, 138:102507.
Shazeer, N. (2020). Glu variants improve transformer. arXiv
preprint arXiv:2002.05202.
Vaswani, A., Shazeer, N., Parmar, N., Uszkoreit, J., Jones,
L., Gomez, A. N., Kaiser, Ł., and Polosukhin, I.
(2017). Attention is all you need. Advances in neural
information processing systems, 30.
Wang, X., Salmani, M., Omidi, P., Ren, X., Reza-
gholizadeh, M., and Eshaghi, A. (2024). Beyond
the limits: A survey of techniques to extend the con-
text length in large language models. arXiv preprint
arXiv:2402.02244.
HEALTHINF 2025 - 18th International Conference on Health Informatics
586