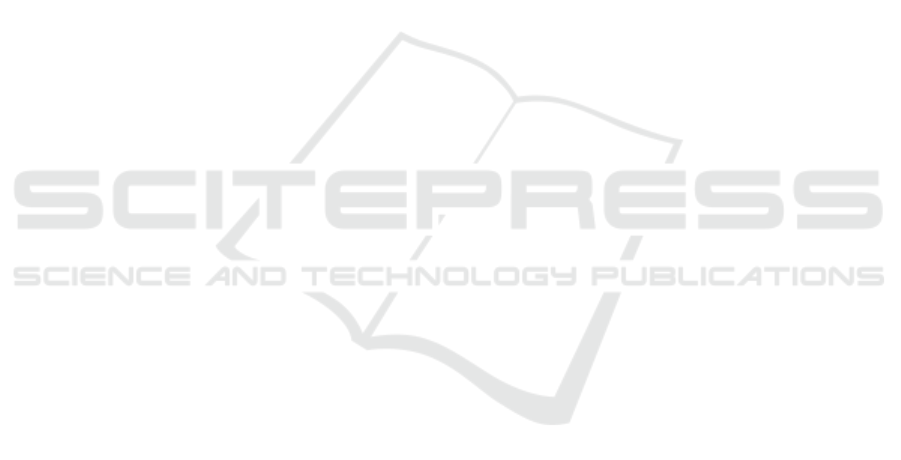
Our research has the potential to contribute to the
area of cyber security by building an automated agent
designed to extract meaningful data from dark web
marketplaces while adhering to ethical standards. By
supporting certified authorities in the collection and
monitoring of real-time data, trends in the sale of
products such as arms, biological weapons, or killing
contracts can be tracked. This allows authorities to
gather potential leads on terrorist attacks or harm-
ful activities, enhancing their ability to address such
threats preemptively. This tool can also help iden-
tify emerging patterns in malware trade, providing
insights into improving security software, mitigat-
ing vulnerabilities, and helping in the fight against
cybercrimes. Although our agent solves the anti-
phishing CAPTCHA of the Dark Matter Marketplace
efficiently, it may occasionally require multiple at-
tempts since OpenAI API may flag some CAPTCHA
images for ethical reasons. Additionally, we open-
source the data collected in the scraping process to
contribute to the research community.
Looking forward, we aim to enhance the agent’s
reliability, speed, and adaptability, particularly in han-
dling a broader range of CAPTCHAs across both the
dark and clear web. The ultimate vision is to fully
integrate MLLMs, minimizing the need for exten-
sive training while offering more flexible and efficient
CAPTCHA-solving capabilities.
REFERENCES
Bergman, J. and Popov, O. B. (2023). Exploring dark
web crawlers: A systematic literature review of dark
web crawlers and their implementation. IEEE Access,
11:35914–35933.
Burda, P., Boot, C., and Allodi, L. (2019). Characteriz-
ing the redundancy of darkweb. onion services. In
Proceedings of the 14th International Conference on
Availability, Reliability and Security, pages 1–10.
Cascavilla, G., Catolino, G., and Sangiovanni, M. (2023).
Illicit darkweb classification via natural-language pro-
cessing: Classifying illicit content of webpages
based on textual information. arXiv preprint
arXiv:2312.04944.
Connolly, K., Klempay, A., McCann, M., and Brenner, P.
(2023). Dark web marketplaces: Data for collabora-
tive threat intelligence. Digital Threats: Research and
Practice, 4(4):1–12.
Csuka, K., Gaastra, D., and de Bruijn, Y. (2018). Breaking
captchas on the dark web.
De Pascale, D., Cascavilla, G., Tamburri, D. A., and Van
Den Heuvel, W. J. (2024). Crator a crawler for tor:
Turning dark web pages into open source intelligence.
In European Symposium on Research in Computer Se-
curity, pages 144–161. Springer.
Deng, G., Ou, H., Liu, Y., Zhang, J., Zhang, T., and Liu, Y.
(2024). Oedipus: Llm-enchanced reasoning captcha
solver. arXiv preprint arXiv:2405.07496.
Dinh, N. and Ogiela, L. (2022). Human-artificial in-
telligence approaches for secure analysis in captcha
codes. EURASIP Journal on Information Security,
2022(1):8.
Hayes, D. R., Cappa, F., and Cardon, J. (2018). A frame-
work for more effective dark web marketplace inves-
tigations. Information, 9(8).
Horan, C. and Saiedian, H. (2021). Cyber crime inves-
tigation: Landscape, challenges, and future research
directions. Journal of Cybersecurity and Privacy,
1(4):580–596.
Kaur, S. and Randhawa, S. (2020). Dark web: A
web of crimes. Wireless Personal Communications,
112:2131–2158.
Khatavkar, V., Velankar, M., and Petkar, S. (2024).
Segmentation-free connectionist temporal classifica-
tion loss based ocr model for text captcha classifica-
tion. arXiv preprint arXiv:2402.05417.
Ma, Y., Zhong, G., Liu, W., Sun, J., and Huang, K. (2020).
Neural captcha networks. Applied Soft Computing,
97:106769.
Motoyama, M., Levchenko, K., Kanich, C., McCoy,
D., Voelker, G. M., and Savage, S. (2010).
Re:{CAPTCHAs—Understanding}{CAPTCHA-
Solving} services in an economic context. In 19th
USENIX Security Symposium (USENIX Security 10).
Platzer, F. and Lux, A. (2022). A synopsis of critical aspects
for darknet research. In Proceedings of the 17th in-
ternational conference on availability, reliability and
security, pages 1–8.
Radivojevic, K., Connolly, K., Klempay, A., and Brenner,
P. (2024). Dark web and internet freedom: navigating
the duality to facilitate digital democracy. Journal of
Cyber Policy, pages 1–16.
Ruiz R
´
odenas, J. M., Pastor-Galindo, J., and
G
´
omez M
´
armol, F. (2023). A general and mod-
ular framework for dark web analysis. Cluster
Computing, pages 1–17.
Saleem, J., Islam, R., and Kabir, M. A. (2022). The
anonymity of the dark web: A survey. IEEE Access,
10:33628–33660.
Sch
¨
afer, M., Fuchs, M., Strohmeier, M., Engel, M., Liechti,
M., and Lenders, V. (2019). Blackwidow: Monitoring
the dark web for cyber security information. In 2019
11th International Conference on Cyber Conflict (Cy-
Con), volume 900, pages 1–21.
Spagnoletti, P., Ceci, F., and Bygstad, B. (2022). Online
black-markets: An investigation of a digital infrastruc-
ture in the dark. Information Systems Frontiers, pages
1–16.
Yannikos, Y. and Heeger, J. (2024). Captchas on darknet
marketplaces: Overview and automated. Electronic
Imaging, 36:1–6.
Yannikos, Y., Heeger, J., and Steinebach, M. (2022). Data
acquisition on a large darknet marketplace. In Pro-
ceedings of the 17th International Conference on
Availability, Reliability and Security, pages 1–6.
Multimodal Web Agents for Automated (Dark) Web Navigation
443