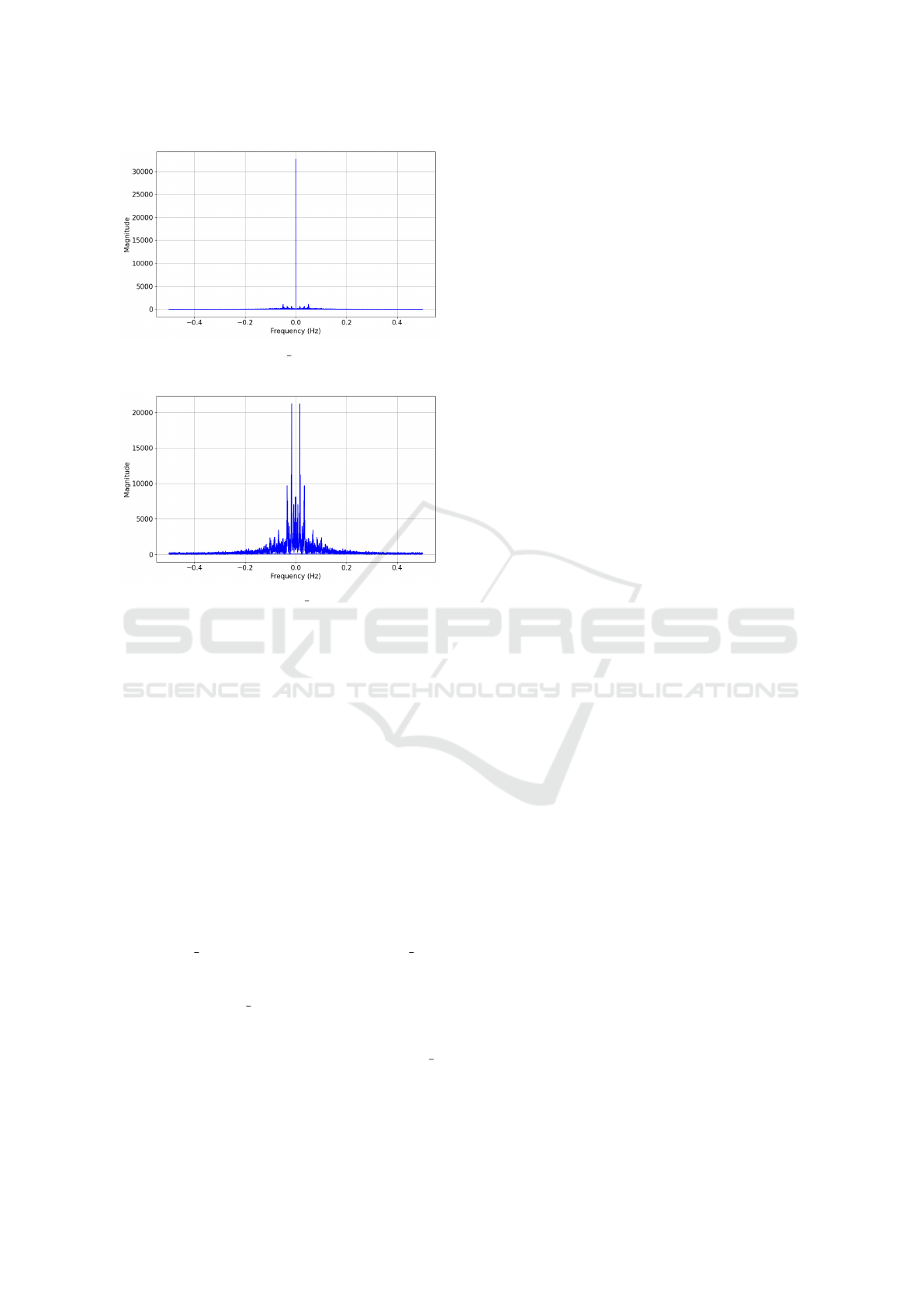
Figure 11: FFT analysis of acc x from wearable sensor and
smartphone data, showing the frequency spectrum.
Figure 12: FFT analysis of gyro y from wearable sensor
and smartphone data, showing dynamic movement across
frequencies.
5 CONCLUSION
Linear correlation provides an overview of relation-
ships between signals but lacks precision in evalu-
ating agreement. In contrast, Bland-Altman plots
and Lin’s Concordance Correlation Coefficient of-
fer a more robust assessment, essential for validating
methods in clinical and biomechanical studies. Error
metrics like RMSE and MAE quantify discrepancies,
while FFT confirms whether devices capture critical
motion frequencies similarly.
Our findings demonstrate that smartphones with
inertial sensors are highly promising for gait anal-
ysis. They showed strong correlations with the
G-Walk system for variables like absolute accel-
eration (acc abs) and gyroscope data (gyro abs).
Bland-Altman analysis revealed satisfactory agree-
ment overall, though notable discrepancies in the gy-
roscope Y-axis (gyro y) highlight limitations in cap-
turing specific rotational movements. Error metrics
confirmed these results, with minimal errors in abso-
lute acceleration and larger discrepancies in gyro y.
FFT analysis validated the smartphone’s ability to
represent essential gait cycle phases accurately.
This study establishes smartphones as accessible
alternatives for gait analysis, though improving Y-
axis gyroscope accuracy is critical for complete in-
terchangeability. Future work should focus on devel-
oping algorithms to analyze accelerometer and gyro-
scope data, identify gait phases, and generate detailed
reports for healthcare professionals.
REFERENCES
Arshad, M., Jamsrandorj, A., Kim, J., and Mun, K.-R.
(2022). Gait events prediction using hybrid cnn-rnn-
based deep learning models through a single waist-
worn wearable sensor. Sensors, 22(21):8226.
BTS Bioengineering (2024). G-walk: Wearable inertial sen-
sor for motion analysis.
Caro-Alvaro, S., Garcia-Lopez, E., et al. (2024). Gesture-
based interactions: Integrating accelerometer and gy-
roscope sensors in the use of mobile apps. Sensors,
24(3):1004.
Das, S., Meher, S., and Sahoo, U. K. (2022). A uni-
fied local–global feature extraction network for hu-
man gait recognition using smartphone sensors. Sen-
sors, 22(11):3968.
Hausdorff, J. and Alexander, N. (2005). Gait disorders eval-
uation and management. CRC Press.
Huang, H., Zhou, P., Li, Y., and Sun, F. (2021). A
lightweight attention-based cnn model for efficient
gait recognition with wearable imu sensors. Sensors,
21(8):2866.
Kim, Y., Bang, H., et al. (2018). Introduction to kalman
filter and its applications. Introduction and Implemen-
tations of the Kalman Filter, 1:1–16.
Kocuvan, P., Hrasti
ˇ
c, A., Kareska, A., and Gams, M.
(2023). Predicting a fall based on gait anomaly de-
tection: a comparative study of wrist-worn three-axis
and mobile phone-based accelerometer sensors. Sen-
sors, 23(19):8294.
Lopez-Nava, I. H., Valent
´
ın-Coronado, L. M., Garcia-
Constantino, M., and Favela, J. (2020). Gait ac-
tivity classification on unbalanced data from inertial
sensors using shallow and deep learning. Sensors,
20(17):4756.
Marimon, X., Mengual, I., et al. (2024). Kinematic analysis
of human gait in healthy young adults using imu sen-
sors: exploring relevant machine learning features for
clinical applications. Bioengineering, 11(2):105.
Ramli, A., Liu, X., , et al. (2024). Gait characterization in
duchenne muscular dystrophy (dmd) using a single-
sensor accelerometer: classical machine learning and
deep learning approaches. Sensors, 24(4):1123.
Shi, L.-F., Liu, Z.-Y., Zhou, K.-J., Shi, Y., and Jing, X.
(2023). Novel deep learning network for gait recog-
nition using multimodal inertial sensors. Sensors,
23(2):849.
Staacks, S., H
¨
utz, S., Heinke, H., and Stampfer, C. (2018).
Advanced tools for smartphone-based experiments:
phyphox. Physics education, 53(4):045009.
STMicroelectronics (2024). Lsm6dso. Acessado em: 20 de
outubro de 2024.
HEALTHINF 2025 - 18th International Conference on Health Informatics
602