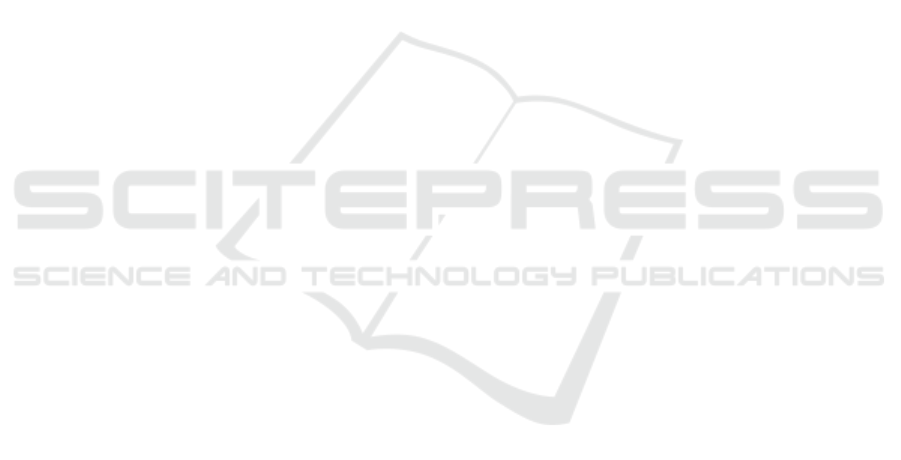
tivities. The automated timestamp extraction process,
while efficient, may introduce minor imprecisions in
event timing. Additionally, news coverage can vary
significantly across different neighbourhoods, poten-
tially affecting the spatial distribution analysis in our
findings.
The reliance on public news sources introduces
certain limitations in data completeness, as some in-
cidents may go unreported or receive limited cover-
age. These constraints particularly affect the analysis
of less newsworthy crimes or incidents in areas with
reduced media attention. Furthermore, the temporal
accuracy of reported events may vary based on the
delay between occurrence and reporting, potentially
affecting the precision of our temporal analysis.
5.5 Implications and Future Directions
This experimental setup demonstrates the potential
for developing comprehensive crime analysis systems
at the municipal level, particularly beneficial for cities
lacking robust governmental crime tracking infras-
tructure. The methodology presented here offers a
foundation for developing more sophisticated crime
analysis tools, especially in regions where official
crime mapping resources are limited or unavailable.
Future enhancements to this methodology could
include integration with official police records to vali-
date and supplement news-based patterns. The imple-
mentation of advanced machine learning algorithms
could improve pattern prediction and anomaly detec-
tion capabilities, whilst real-time updating systems
could provide immediate insights for law enforce-
ment and public safety officials. Cross-validation
with similar-sized municipalities could help identify
common patterns and unique local characteristics, en-
abling more targeted intervention strategies.
The analysis framework developed in this study
shows particular promise for medium-sized cities
seeking to implement data-driven crime prevention
strategies. By combining news source analysis with
geographical information systems, cities can develop
more effective approaches to resource allocation and
crime prevention, even in the absence of sophisticated
governmental tracking systems.
Our findings demonstrate both the potential and
limitations of leveraging news-based data for urban
crime analysis. While the methodology provides
valuable insights into crime patterns, the inherent bi-
ases in news reporting must be carefully considered
when interpreting results. Nevertheless, this approach
offers a promising foundation for cities seeking to
develop data-driven crime prevention strategies, par-
ticularly in regions where official crime mapping re-
sources are limited. The framework established here
can serve as a template for other municipalities look-
ing to enhance their understanding of local crime pat-
terns through systematic analysis of publicly available
information.
6 CONCLUSIONS AND FUTURE
WORK
This study has successfully developed a method to
generate an urban crime dataset for the city of Santa
Maria, addressing a significant gap in the availabil-
ity of structured crime data for analysis. The result-
ing dataset provides a valuable tool for crime analy-
sis in a city that previously lacked a formatted crime
database. Key achievements include the creation of
a structured crime dataset for Santa Maria, the de-
velopment of a methodology adaptable for generat-
ing new crime reports, and the provision of a tool for
validating existing crime data. While the method has
proven effective, several limitations and challenges
were identified. News repetition poses a significant
challenge, as the same crime event may be reported
multiple times, potentially skewing the dataset. Addi-
tionally, the accuracy of data presented in news con-
tent may not always perfectly align with reality. Tem-
poral consistency is another concern, as the coverage
and reporting of crimes in news outlets may vary over
time, affecting long-term trend analysis.
Despite these limitations, the dataset has already
yielded valuable insights. The heat map analysis in-
dicates that the city center experiences the highest
concentration of reported crimes, providing crucial
information for law enforcement resource allocation.
Analysis of crime occurrence by hour offers insights
into the most dangerous times in the city, which can
inform public safety strategies. With further refine-
ment, the dataset holds potential for more complex
correlations, such as examining the influence of tem-
perature on crime rates or identifying seasonal crime
patterns. To enhance the value and accuracy of this
dataset, several avenues for future work are proposed.
Collaboration with law enforcement is crucial; part-
nering with the local police department to validate and
refine the dataset will significantly improve its accu-
racy and comprehensiveness. Enhanced data clean-
ing techniques should be developed to identify and
remove duplicate reports while preserving unique in-
cidents. Integration with official records, combin-
ing this dataset with official police data, will create a
more complete and accurate picture of crime in Santa
Maria.
ICAART 2025 - 17th International Conference on Agents and Artificial Intelligence
748