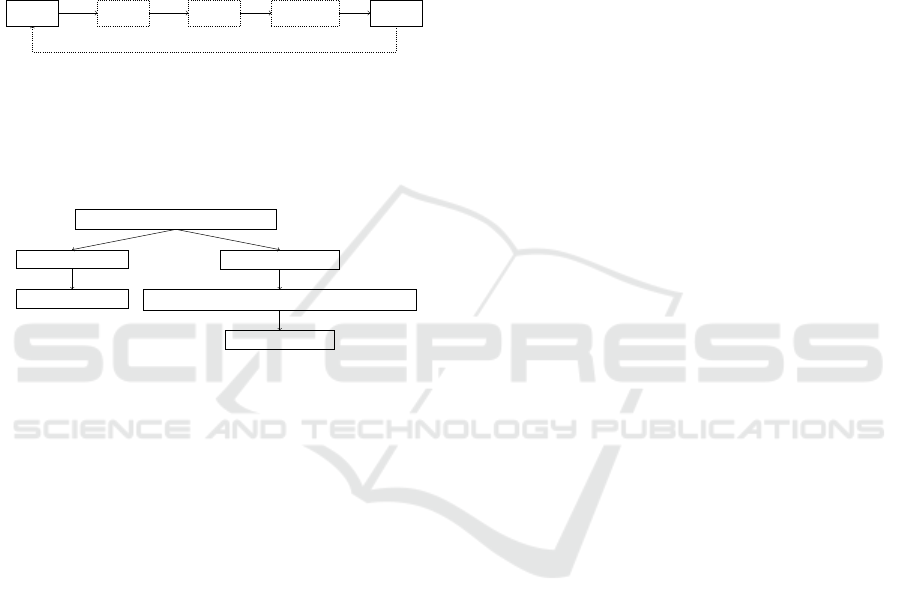
some steps involved in between, such as disengag-
ing (D), positioning (P), or applying pressure (AP).
While the last one doesn’t require a proper grasping
of the object, as it can be seen from Table 2, G5 mo-
tion describes the motion that allows touching an ob-
ject without properly grasping it. Additionally, Fig. 2
shows the corresponding arm motions that take place
between those MTM-1 basic hand motions. This way,
after an object is grasped by the hand, it is then moved
with the arm, released by the hand, and then the next
object is reached by the arm and grasped by the hand.
2.6 Transcription algorithm
relevant data is being analyzed. For instance, when a user initiates contact with an object, a hand motion
is performed. The decision tree for the hand motions can be triggered and will then only determine which
hand motion code was performed.
These decision trees all run simultaneous and individually listen for their trigger to be activated. The
hand and body motion decision tree work on their own, while the arm motions tree depends on data from
the hand motions tree and the leg motion tree relies on data from the body motion tree.
The upcoming Subsections elaborate on the decision trees and the corresponding triggers that initiate
them. These details will provide a better understanding on their implementation and especially on how
they are triggered.
2.6.1 Hand motions
Hand motions occur when objects are grasped or released. Therefore, the trigger for the hand motion
decision tree is the beginning or end of touching an object. At this point, it is certain that a reach or
grasp motion was performed. This approach has good synergy with the Sensoryx SDK, as it also needs
to know if an object is grasped or released for hand tracking. By utilizing this trigger, we can ensure that
the hand motions decision tree is only applied when it is relevant.
The 5 hand motions are grasp, release, position, apply pressure and disengage. These motions were
observed to commonly occur in the order illustrated in Figure 2.13.
Grasp Disengage
Position
Apply pressure
Release
Move
Reach
Figure 2.13: Hand motions cycle in MTM-1. The dashed motions are optional in a cycle.
The grasp and release motions are always present in a cycle. Their code and differentiation options are
displayed in Tables 2.1 and 2.2. The motions disengage, position and apply pressure are optional in a
cycle and are performed while holding an object, so between grasping and releasing. After pressure is
applied, the object is commonly released. While it is plausible to have multiple positioning or disengag-
ing motions in a cycle, it is not common for most tasks. The algorithm in this state is not able to detect
multiple disengage or position motions in one cycle. It is however able to detect the standard interaction
with an object that involves both of the motions in one cycle. That would be grasping it, disengaging it
from somewhere, position it somewhere else and then release it again.
25
Figure 2: Hand motions cycle in MTM-1. The dashed mo-
tions are optional in a cycle.
Fig. 3 represents the general idea of our decision
tree approach. We first check if either grasping the
object or releasing it was performed.
Decision trees Roman’s work
gorobets
June 2024
Hand motion type (grasp or release)
Grasp transcription
Reach transcription
Release transcription
Crank/position/disengage transcription (optional)
Move transcription
1
Figure 3: Interconnection between different motions.
3.3.1 Hand Motion Decision Tree
The hand decision tree (Fig. 4) is triggered when the
beginning or the end of touching a VO occurs. First,
we check whether we detected the beginning or end
of the virtual hand collision with the VO. If we detect
the end, we transcribe the release motion; otherwise -
a grasp motion. To distinguish between two different
types of release, the previous grasp is considered. If
it was G5, then RL2 is transcribed, otherwise RL1.
Distinguishing between different grasping types
requires additional knowledge about the grasped ob-
ject. In VR, it is possible to get information about
VO’s features. In our approach, we manually labeled
each VO if it was small, cylindrical, or in a group of
similar objects. It is easy to do as we are manually
setting the VE and all VOs in it. Once a grasping ac-
tion is detected, we check whether a regrasp of the
same object with the same hand was performed. For
this, we introduce a time limit of 1 second between the
time when an object was placed and grasped again. If
it is less than 1 second, we transcribe regrasp. Oth-
erwise, we check if the object was passed to another
hand without being put back on the surface. In this
case, we transcribe G3. Next, we consider whether a
VO has a label of being in a group (G4) or if it is la-
beled as small (G1B) or cylindrical (G1C). If neither
of those is true, we transcribe it as a normal grasp-
ing (G1A). Additionally, we check whether the hand
is properly closed or not (G5).
Fig. 4 shows all grasping motions (except regrasp-
ing), and triggers the arm decision tree that transcribes
a preceding arm motion with the consideration of the
recently transcribed hand motion. Hand motions po-
sition and apply pressure require knowledge about the
applied force. As this is not applicable in VR, our im-
plementation of apply pressure detection is based on
knowledge about the process. In our user study (Ta-
ble 4), we automatically transcribe AP code when the
screwing task is performed, as we know that this pro-
cess requires the application of the force in reality.
Similarly, our algorithm can not detect different
specifications of the position hand motion. However,
we combined them into one motion without specifica-
tions. We transcribe position when a VO reaches its
predefined location. For example, as soon as the ham-
merhead is properly positioned on the corresponding
red rectangle, we transcribe position. Disengage hand
motions appear when a previously inserted nail is ex-
tracted from a hole. We track two engaged VOs: if
they were engaged during the preceding grasping and
no longer touch each other after the release motion is
detected, we transcribe it as disengage.
3.3.2 Arm Motion Decision Tree
The arm decision tree (Fig. 5) is triggered by the out-
put of the hand decision tree. Once the MTM-1 code
for the hand motion is derived, we can also confirm
that the corresponding arm motion was performed.
It is important to note that the turn motion does
not intuitively fit into the arm motions category. Its
definition is the turning of the wrist during a reach or
move motion. Thus, it is always accompanied by arm
motions and is best suited to this category. In a man-
ual transcription, an expert would determine which
motion requires more TMUs and only transcribe the
higher one. So either the turn or the reach/move mo-
tion. However, since this algorithm focuses on mo-
tion detection, it transcribes the turn motion along-
side with reach/move motion. This decision tree is
triggered once the corresponding MTM-1 code for
the hand action is received. Based on this output we
check if it was a release motion or not. If the VO
was released, that means that it was either moved to a
new location or a cranking action was performed. If
not, that means that the object was grasped, and the
preceding corresponding arm motion was reach.
To distinguish between cranking and moving, we
check the label of the VO. If the released VO has the
Automatic Transcription and Detection of the MTM-1 Hand Motions Performed in Virtual Reality
599