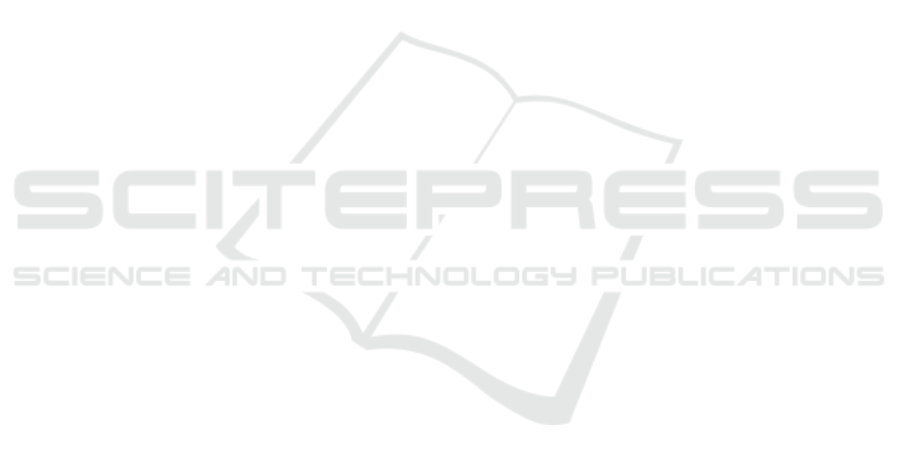
ACKNOWLEDGEMENTS
We would like to thank our business partner for
the fruitful cooperation and all the support. We
thank Christian Zeyen for CBR-related discussions
and valuable feedback. We also thank Mahta
Bakhshizadeh, Desiree Heim, Christian Jilek, Heiko
Maus, and Markus Schr
¨
oder for fruitful discussions
and inspiration. Finally, we would like to thank
anonymous reviewers for their valuable feedback.
REFERENCES
Antoniou, G. and van Harmelen, F. (2004). Web ontology
language: OWL. In Handbook on Ontologies, Interna-
tional Handbooks on Information Systems, pages 67–
92. Springer.
Bardelli, C., Rondinelli, A., Vecchio, R., and Figini, S.
(2020). Automatic electronic invoice classification us-
ing machine learning models. Mach. Learn. Knowl.
Extr., 2(4):617–629.
Belskis, Z., Zirne, M., and Pinnis, M. (2020). Features
and methods for automatic posting account classifi-
cation. In Databases and Information Systems - 14th
Intern. Baltic Conf., volume 1243 of Communications
in Computer and Information Science, pages 68–81.
Springer.
Belskis, Z., Zirne, M., Slaidins, V., and Pinnis, M. (2021).
Natural language based posting account classification.
Balt. J. Mod. Comput., 9(2).
Bergmann, R., Grumbach, L., Malburg, L., and Zeyen, C.
(2019). Procake: A process-oriented case-based rea-
soning framework. In Workshops Proc. for (ICCBR
2019), Otzenhausen, Germany, September 8-12, 2019,
volume 2567 of CEUR Workshop Proceedings, pages
156–161. CEUR-WS.org.
Bergmeir, C. and Ben
´
ıtez, J. M. (2012). On the use of
cross-validation for time series predictor evaluation.
Inf. Sci., 191:192–213.
Ehrlinger, L. and W
¨
oß, W. (2016). Towards a definition of
knowledge graphs. In Joint Proc. of the Posters and
Demos Track of SEMANTiCS2016, Leipzig, Germany,
September 12-15, 2016, volume 1695 of CEUR Work-
shop Proceedings. CEUR-WS.org.
Gr
¨
uninger, M. and Fox, M. S. (1995). The role of compe-
tency questions in enterprise engineering. pages 22–
31. Springer US, Boston, MA.
Hart, P. E., Nilsson, N. J., and Raphael, B. (1968). A formal
basis for the heuristic determination of minimum cost
paths. IEEE Trans. Syst. Sci. Cybern., 4(2):100–107.
Jain, K. and Woodcock, E. (2017). A road map for digitiz-
ing source-to-pay. McKinsey.
Koch, B. (2019). The e-invoicing journey 2019-2025.
billentis GmbH.
Kolodner, J. L. (2014). Case-Based Reasoning. Morgan
Kaufmann.
Lazar, J., Feng, J., and Hochheiser, H. (2017). Research
Methods in Human-Computer Interaction, 2nd Edi-
tion. Morgan Kaufmann.
Levenshtein, V. I. (1966). Binary codes capable of cor-
recting deletions, insertions, and reversals. In Soviet
physics doklady, volume 10, pages 707–710.
Liu, Y., Ott, M., Goyal, N., Du, J., Joshi, M., Chen, D.,
Levy, O., Lewis, M., Zettlemoyer, L., and Stoyanov,
V. (2019). Roberta: A robustly optimized BERT pre-
training approach. CoRR, abs/1907.11692.
Meilicke, C., Chekol, M. W., Betz, P., Fink, M., and
Stuckenschmidt, H. (2024). Anytime bottom-up rule
learning for large-scale knowledge graph completion.
VLDB J., 33(1):131–161.
Meilicke, C., Chekol, M. W., Ruffinelli, D., and Stucken-
schmidt, H. (2019). Anytime bottom-up rule learn-
ing for knowledge graph completion. In Proceedings
of IJCAI 2019, Macao, China, August 10-16, 2019,
pages 3137–3143. ijcai.org.
Mikolov, T., Sutskever, I., Chen, K., Corrado, G. S., and
Dean, J. (2013). Distributed representations of words
and phrases and their compositionality. In Proc. of
27th Annual Conference on Neural Information Pro-
cessing Systems 2013. Lake Tahoe, Nevada, United
States, pages 3111–3119.
Panichi, B. and Lazzeri, A. (2023). Semi-supervised classi-
fication with a*: A case study on electronic invoicing.
Big Data Cogn. Comput., 7(3):155.
Poveda-Villal
´
on, M., G
´
omez-P
´
erez, A., and Su
´
arez-
Figueroa, M. C. (2014). Oops! (ontology pitfall scan-
ner!): An on-line tool for ontology evaluation. Int. J.
Semantic Web Inf. Syst., 10(2):7–34.
Rastrigin, L. A. (1963). The convergence of the ran-
dom search method in the extremal control of a many
parameter system. Automaton & Remote Control,
24:1337–1342.
Schulze, M., Pelzer, M., Schr
¨
oder, M., Jilek, C., Maus, H.,
and Dengel, A. (2022). Towards knowledge graph
based services in accounting use cases. In Proceed-
ings of Poster and Demo Track of SEMANTiCS 2022,
Vienna, Austria, September 13th to 15th, 2022, vol-
ume 3235 of CEUR. CEUR-WS.org.
Schulze, M., Schr
¨
oder, M., Jilek, C., Albers, T., Maus,
H., and Dengel, A. (2021). P2P-O: A purchase-to-
pay ontology for enabling semantic invoices. In The
Semantic Web, ESWC 2021, Virt. Event, June 6-10,
2021, Proc., volume 12731 of LNCS, pages 647–663.
Springer.
Su
´
arez-Figueroa, M. C., G
´
omez-P
´
erez, A., and Fern
´
andez-
L
´
opez, M. (2015). The neon methodology framework:
A scenario-based methodology for ontology develop-
ment. Appl. Ontology, 10(2):107–145.
Valle, E. D. and Ceri, S. (2011). Querying the semantic
web: SPARQL. In Handbook of Semantic Web Tech-
nologies, pages 299–363. Springer.
World Wide Web Consortium (2014a). The organization
ontology, http://www.w3.org/tr/vocab-org/.
World Wide Web Consortium (2014b). Rdf 1.1 primer,
http://www.w3.org/tr/2014/note-rdf11-primer-
20140624/.
ICAART 2025 - 17th International Conference on Agents and Artificial Intelligence
452