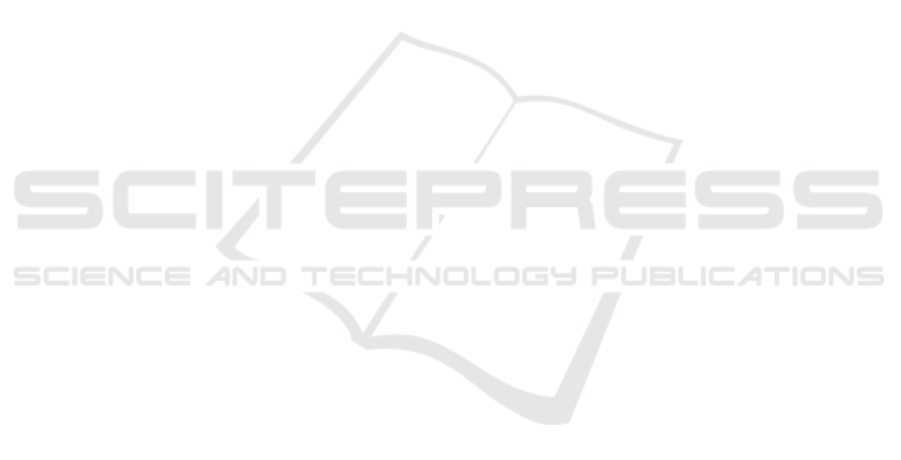
Unlike humans, who continuously process large
volumes of data and face a variety of challenges over
time, deep learning models typically learn from a
more limited dataset tailored to a specific task. This
study focused on exploring the impact of an optimized
data pipeline on the performance of a deep learn-
ing model, highlighting the significant improvements
that can be achieved through a data-driven approach.
Our findings suggest that combining robust data engi-
neering with a relatively simple convolutional neural
network architecture, such as the Siamese network,
holds great potential for advancing clinical applica-
tions. Specifically, the model can be leveraged to
predict responses to anti-VEGF treatment in patients
with diabetic macular edema (DME), offering a valu-
able tool for personalized treatment strategies.
The use of the Siamese network architecture in our
study, designed for scenarios involving small datasets,
was particularly beneficial given the limited size of
our private OCT image dataset. However, several av-
enues remain for enhancing clinical outcomes. Fu-
ture research could focus on expanding the dataset by
including diverse patient populations to improve the
generalizability of the model. Additionally, integrat-
ing multimodal data, such as clinical histories or ge-
netic information, could enhance predictive accuracy.
Exploring transfer learning or semi-supervised learn-
ing techniques could also help overcome the limita-
tions of small datasets and expand the applicability
of this approach to other retinal pathologies and dis-
eases beyond DME. These steps could strengthen the
model’s potential in clinical settings, ultimately lead-
ing to more accurate and timely treatment predictions
for patients.
REFERENCES
Bhagat, N., Grigorian, R. A., Tutela, A., and Zarbin, M. A.
(2009). Diabetic macular edema: pathogenesis and
treatment. Survey of Ophthalmology, 54(1):1–32.
Brown, D. M., Nguyen, Q. D., Marcus, D. M., Boyer, D. S.,
Patel, S., Feiner, L., and Ehrlich, J. S. (2013). Long-
term outcomes of ranibizumab therapy for diabetic
macular edema: the 36-month results from two phase
iii trials. Ophthalmology, 120(10):2013–2022.
Browning, D. J., Glassman, A. R., Aiello, L. P., Beck,
R. W., Brown, D. M., Fong, D. S., and Ferris, F. L.
(2007). Relationship between optical coherence to-
mography–measured central retinal thickness and vi-
sual acuity in diabetic macular edema. Ophthalmol-
ogy, 114(3):525–536.
Cao, J., You, K., Jin, K., Lou, L., Wang, Y., Chen, M., and
Ye, J. (2021). Prediction of response to anti-vascular
endothelial growth factor treatment in diabetic mac-
ular oedema using an optical coherence tomography-
based machine learning method. Acta Ophthalmolog-
ica, 99(1):e19–e27.
Ding, F. and Zhu, F. (2022). Hliferl: A hierarchical life-
long reinforcement learning framework. Journal of
King Saud University - Computer and Information
Sciences, 34(7):4312–4321.
Fauw, J. D., Ledsam, J. R., Romera-Paredes, B., Nikolov,
S., Tomasev, N., Blackwell, S., and Ronneberger, O.
(2018). Clinically applicable deep learning for diag-
nosis and referral in retinal disease. Nature Medicine,
24(9):1342–1350.
Ferrara, N., Hillan, K. J., Gerber, H. P., and Novotny, W.
(2004). Discovery and development of bevacizumab,
an anti-vegf antibody for treating cancer. Nature Re-
views Drug Discovery, 3(5):391–400.
Grandini, E. B. and Visani, G. (2020). Metrics for multi-
class classification: an overview. arXiv preprint,
2008.05756.
Jin, Y., Yong, S., Ke, S., Zhang, C., Liu, Y., Wang, J., and
Zhang, J. (2024). Deep learning assisted fluid vol-
ume calculation for assessing anti-vascular endothe-
lial growth factor effect in diabetic macular edema.
Heliyon, 10(8).
Kermany, D. S., Goldbaum, M., Cai, W., Valentim, C. C.,
Liang, H., Baxter, S. L., and Zhang, K. (2018). Iden-
tifying medical diagnoses and treatable diseases by
image-based deep learning. Cell, 172(5):1122–1131.
Ko, Y., Peng, C., Ho, H., Chiu, S., Chen, S., and Lee, C.
(2022). Deep learning assisted prediction of long-term
visual outcome after 3 monthly anti-vascular endothe-
lial growth factor injections in patients with central-
involved diabetic macular edema. Investigative Oph-
thalmology & Visual Science, 63(7):3778–F0199.
Koch, G., Zemel, R., and Salakhutdinov, R. (2015).
Siamese neural networks for one-shot image recogni-
tion. In ICML Deep Learning Workshop, volume 2,
Lille.
Liu, S., Hu, W., Xu, F., Chen, W., Liu, J., Yu, X., and
Li, J. (2023). Prediction of oct images of short-
term response to anti-vegf treatment for diabetic mac-
ular edema using different generative adversarial net-
works. Photodiagnosis and Photodynamic Therapy,
41:103272.
Meng, Z., Chen, Y., Li, H., Zhang, Y., Yao, X., Meng,
Y., and Luo, J. (2024). Machine learning and optical
coherence tomography-derived radiomics analysis to
predict persistent diabetic macular edema in patients
undergoing anti-vegf intravitreal therapy. Journal of
Translational Medicine, 22(1):358.
Schlegl, T., Waldstein, S. M., Bogunovic, H., Endstraßer, F.,
Sadeghipour, A., Philip, A. M., and Schmidt-Erfurth,
U. (2018). Fully automated detection and quantifica-
tion of macular fluid in oct using deep learning. Oph-
thalmology, 125(4):549–558.
Taigman, Y., Yang, M., Ranzato, M., and Wolf, L. (2014).
Deepface: Closing the gap to human-level perfor-
mance in face verification. In Proceedings of the IEEE
Conference on Computer Vision and Pattern Recogni-
tion, pages 1701–1708.
A Deep Learning Approach for Predicting the Response to Anti-VEGF Treatment in Diabetic Macular Edema Patients Using Optical
Coherence Tomography Images
461