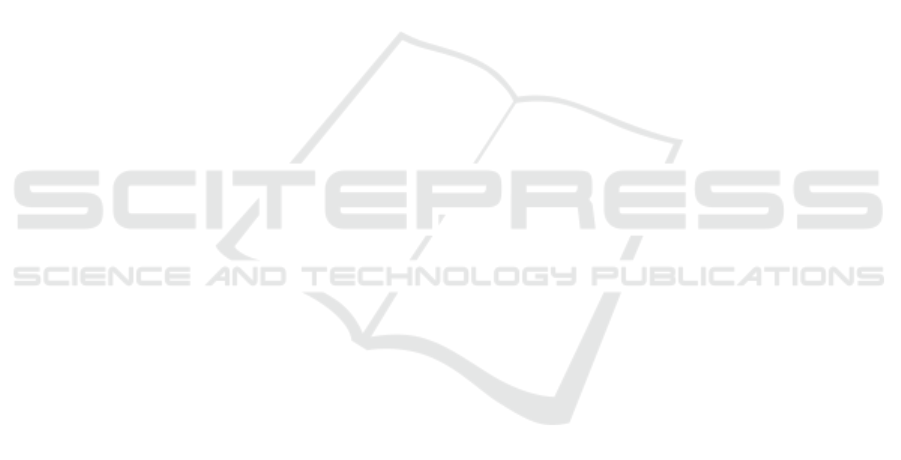
outperform traditional data management methods in
environments with limited network connections, as is
often the case in rural areas. This opens the way for
wider adoption of this technology, not only in agri-
culture but also in other sectors where data privacy
and limited access to infrastructure are major con-
cerns (McMahan et al., 2017) (Li et al., 2020).
5.4 Limitations of the Approach and
Prospects for Improvement
Although the results of this study are promising, some
limitations remain. One of the main limitations is the
latency of communications between local devices and
the central server, which can affect the speed of fed-
erated model training. This is particularly problem-
atic in agricultural areas that are poorly connected. In
addition, the heterogeneity of IoT devices and data
formats can lead to inconsistencies in local model up-
dates (Smith et al., 2017).
In the future, more research is needed to improve
the model’s resilience to these challenges. One pos-
sible way would be to explore approaches for com-
pression of data before transmission, as well as ad-
vanced optimization techniques to reduce the band-
width required when exchanging between local de-
vices and the central server. In addition, the integra-
tion of reinforcement algorithms could allow for a dy-
namic adaptation of the model to specific local condi-
tions, thus further improving the accuracy of predic-
tions (Park and Sim, 2020) (Kone
ˇ
cn
´
y et al., 2016).
6 CONCLUSIONS AND FUTURE
WORK
Federated learning is an innovative and promising so-
lution for managing agricultural data in the area of
smart agriculture. This approach offers an effective
alternative to centralized methods, allowing machine
learning models to be trained without the need for
massive data transfer, thus ensuring the confidential-
ity and security of local information. In this study,
we demonstrated that FL applied to soil classification
provides high-precision results while adapting to the
geographic and technological constraints of agricul-
tural operations.
In addition, the integration of our solution within a
microservices architecture via an MLaaS service has
proven its effectiveness in terms of scalability and
flexibility. This approach allows for seamless interac-
tion between different users of the system while pro-
viding continuous update capability and rapid deploy-
ment. Applying this technology to smart farming can
revolutionize agricultural practices, making it easier
to make decisions and optimizing crop management
through personalized recommendations based on soil
data.
However, some limitations such as communica-
tion latency and the heterogeneity of IoT devices re-
quire further research. Improvements can be made,
including through the optimization of federated drive
processes and network resource management in envi-
ronments with limited connectivity. In the future, the
integration of reinforcement learning techniques and
data compression methods could further enhance the
effectiveness of this approach.
In conclusion, federated learning combined with a
distributed service architecture is a breakthrough for
smart agriculture. It offers not only high performance
but also better data protection, a crucial factor in the
development of more sustainable and innovative agri-
culture.
REFERENCES
Assem, H., Xu, L., Buda, T. S., and O’Sullivan, D. (2016).
Machine learning as a service for enabling internet of
things and people. Personal and Ubiquitous Comput-
ing.
Bacciu, D., Chessa, S., Gallicchio, C., and Micheli, A.
(2017). On the need of machine learning as a service
for the internet of things. In Proceedings of the 1st In-
ternational Conference on Internet of Things and Ma-
chine Learning.
Bonawitz, K., Eichner, H., Grieskamp, W., Huba, D., Inger-
man, A., Ivanov, V., Kiddon, C., Kone
ˇ
cn
´
y, J., Maz-
zocchi, S., McMahan, H., Overveldt, T. V., Petrou, D.,
Ramage, D., and Roselander, J. (2019). Towards fed-
erated learning at scale: System design. In Proceed-
ings of the 2nd SysML Conference.
Garc
´
ıa, L., Parra, L., Jim
´
enez, J., Lloret, J., and Lorenz,
P. (2020). Iot-based smart irrigation systems: An
overview on the recent trends on sensors and iot sys-
tems for irrigation in precision agriculture. Sensors,
20(4):1042.
Kairouz, P., McMahan, H., et al. (2019). Advances and
open problems in federated learning. Foundations and
Trends® in Machine Learning, 11(3-4):185–383.
Kone
ˇ
cn
´
y, J., McMahan, H., Yu, F., et al. (2016). Feder-
ated learning: Strategies for improving communica-
tion efficiency. In Proceedings of the 19th Interna-
tional Conference on Neural Information Processing
Systems.
Li, T., Sahu, A., Talwalkar, A., and Smith, V. (2020). Fed-
erated learning: Challenges, methods, and future di-
rections. volume 37, pages 50–60.
McMahan, H., Moore, E., Ramage, D., and Arcas, B.
(2017). Communication-efficient learning of deep net-
works from decentralized data. In Proceedings of
Federated Machine Learning Framework for Soil Classification in Smart Agriculture
787