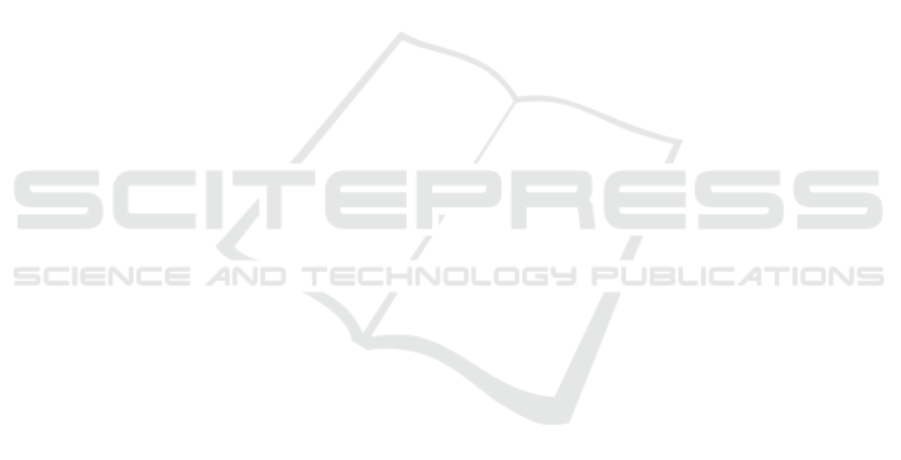
5 CONCLUSION
In this work, we described a design tool for a model-
and simulation-based systems engineering framework
for capturing design decisions and evaluating static
and dynamic properties for ITS design. In addition to
capturing different design decisions, users can guide
design decisions by systematic comparison and eval-
uation of system configuration and control strategies.
Application results demonstrate the feasibility of the
design tool for verification of ITS design decisions
with respect to static and dynamic system properties.
REFERENCES
Ascher, D. and Hackenberg, G. (2015). Integrated trans-
portation and power system modeling. In 2015 Inter-
national Conference on Connected Vehicles and Expo
(ICCVE), pages 379–384.
Ascher, D. and Hackenberg, G. (2016). The transp-0 frame-
work for integrated transportation and power system
design. In 2016 IEEE 19th International Conference
on Intelligent Transportation Systems (ITSC), pages
945–952.
Ascher, D. and Hackenberg, G. (2017). The passenger ex-
tension of the transp-0 system design framework. In
2017 5th IEEE International Conference on Models
and Technologies for Intelligent Transportation Sys-
tems (MT-ITS), pages 256–261.
Ascher, D. and Hackenberg, G. (2023). A discrete event for-
malism for fast simulation of on-demand transporta-
tion systems. In International Conference on Intel-
ligent Systems Design and Applications, pages 185–
197. Springer.
Ascher, D., Hackenberg, G., and Albayrak, S. (2023).
Model-based design of integrated transportation sys-
tems using approximate dynamic programming. In
2023 IEEE 26th International Conference on Intel-
ligent Transportation Systems (ITSC), pages 4443–
4450.
Barcel
´
o, J. and Casas, J. (2005). Dynamic network simula-
tion with aimsun. In Simulation approaches in trans-
portation analysis, pages 57–98. Springer.
Bellman, R. (1957). Dynamic Programming. Princeton
University Press, New Jersey.
Brake, J., Nelson, J. D., and Wright, S. (2004). Demand re-
sponsive transport: towards the emergence of a new
market segment. Journal of Transport Geography,
12(4):323–337.
Ericsson, E., Larsson, H., and Brundell-Freij, K. (2006).
Optimizing route choice for lowest fuel consumption–
potential effects of a new driver support tool. Trans-
portation Research Part C: Emerging Technologies,
14(6):369–383.
Fagnant, D. J. and Kockelman, K. M. (2014). The travel
and environmental implications of shared autonomous
vehicles, using agent-based model scenarios. Trans-
portation Research Part C: Emerging Technologies,
40:1–13.
Fellendorf, M. (1994). VISSIM: A microscopic simulation
tool to evaluate actuated signal control including bus
priority. In 64th Institute of Transportation Engineers
Annual Meeting, volume 32. Springer.
Furuhata, M., Dessouky, M., Ord
´
o
˜
nez, F., Brunet, M.-E.,
Wang, X., and Koenig, S. (2013). Ridesharing: The
state-of-the-art and future directions. Transportation
Research Part B: Methodological, 57:28–46.
Gianni, D., D’Ambrogio, A., and Tolk, A. (2014). Modeling
and simulation-based systems engineering handbook.
CRC Press.
Harris, I., Wang, Y., and Wang, H. (2015). Ict in multimodal
transport and technological trends: Unleashing poten-
tial for the future. International Journal of Production
Economics, 159:88 – 103.
Hu, J., Morais, H., Sousa, T., and Lind, M. (2016). Electric
vehicle fleet management in smart grids: A review of
services, optimization and control aspects. Renewable
and Sustainable Energy Reviews, 56:1207–1226.
Huang, Y., Ng, E. C., Zhou, J. L., Surawski, N. C., Chan,
E. F., and Hong, G. (2018). Eco-driving technology
for sustainable road transport: A review. Renewable
and sustainable energy reviews, 93:596–609.
Jia, D., Lu, K., Wang, J., Zhang, X., and Shen, X. (2015).
A survey on platoon-based vehicular cyber-physical
systems. IEEE communications surveys & tutorials,
18(1):263–284.
Kopelias, P., Demiridi, E., Vogiatzis, K., Skabardonis,
A., and Zafiropoulou, V. (2020). Connected & au-
tonomous vehicles–environmental impacts–a review.
Science of the total environment, 712:135237.
Lopez, P. A., Behrisch, M., Bieker-Walz, L., Erdmann, J.,
Fl
¨
otter
¨
od, Y.-P., Hilbrich, R., L
¨
ucken, L., Rummel, J.,
Wagner, P., and Wießner, E. (2018). Microscopic traf-
fic simulation using SUMO. In 2018 21st Interna-
tional Conference on Intelligent Transportation Sys-
tems (ITSC), pages 2575–2582. IEEE.
Namoun, A., Tufail, A., Mehandjiev, N., Alrehaili, A.,
Akhlaghinia, J., and Peytchev, E. (2021). An eco-
friendly multimodal route guidance system for urban
areas using multi-agent technology. Applied Sciences,
11(5):2057.
Powell, W. B. (2007). Approximate Dynamic Program-
ming: Solving the Curses of Dimensionality. John
Wiley & Sons.
W Axhausen, K., Horni, A., and Nagel, K. (2016). The
multi-agent transport simulation MATSim. Ubiquity
Press.
Wang, B., Han, Y., Wang, S., Tian, D., Cai, M., Liu, M., and
Wang, L. (2022). A review of intelligent connected
vehicle cooperative driving development. Mathemat-
ics, 10(19):3635.
Zhang, R. and Fujimori, S. (2020). The role of trans-
port electrification in global climate change miti-
gation scenarios. Environmental Research Letters,
15(3):034019.
Next-Generation Design Tools for Intelligent Transportation Systems
241