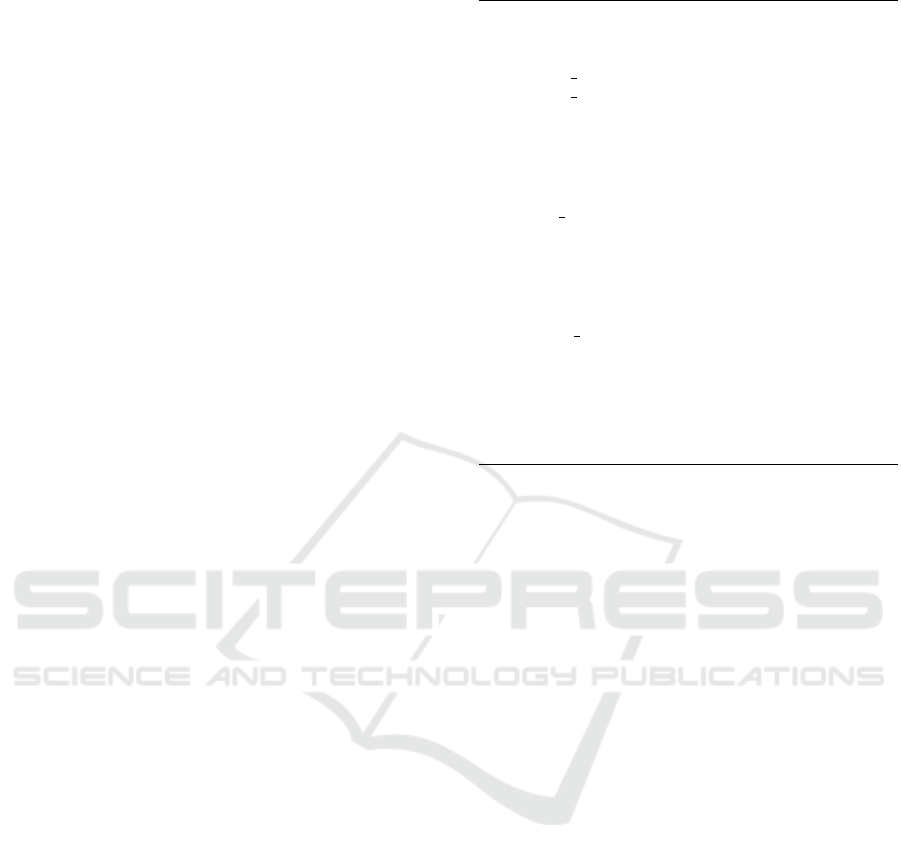
which objects to interact with becomes more chal-
lenging.
5.2.2 Placement Task
These tasks involve placing objects in specific loca-
tions within the environment, such as “Put all apples
in the fridge.” The targeted objects for these tasks in-
clude those that the robot can grasp and those that can
receive other objects (receptacles).
Similar to state change tasks, placement tasks are
divided into two difficulty levels: simple tasks, which
involve placing a single object, like “Put an apple in
the fridge,” and more complex tasks, which involve
placing multiple objects like “Put all apples in the
fridge.”
Here too, we focused on designing the more com-
plex tasks. Some receptacles, like fridges, may have
doors that can be either “OPEN” or “CLOSED.” the
dataset includes two initial state patterns for the re-
ceptacles (“OPEN” or “CLOSED”). Before placing
an object, the agent must infer whether the recepta-
cle’s door needs to be opened using home environ-
ment knowledge. This design increases the difficulty,
as the task cannot be completed successfully without
such inference.
5.2.3 Dataset for VirtualHome
As described in Section 4.7, this study utilizes VH as
the simulator. Therefore, the dataset was designed to
be compatible with VH.
VH includes seven different scenes (houses), each
containing various rooms and different objects within
those spaces. Tasks were configured according to the
unique characteristics of each scene.
In VH, there are four types of object states: “ON,”
“OFF,” “Open,” and “CLOSED.” Considering object
variations in VH, we constructed a dataset for the state
change task by targeting seven types of objects with
a power supply (e.g., lightswitch, tablelamp). Addi-
tionally, for the placement task, we limited the place-
ment to movable objects classified as food or drink
items (e.g., banana, milk), and the placement loca-
tions were selected based on their suitability for plac-
ing such items (e.g., kitchentable, fridge). The dataset
was designed with twelve types of food and drink
items and eight designated placement locations.
Examples of the dataset are shown in Listing 2 and
3. The dataset consists the task description, the scene
in which the task takes place, the agent’s initial po-
sition, the initial states of the objects, the goal states
of the objects, and the action script necessary for the
agent to complete the task.
Listing 2: Example of State Change Task for VH.
{
”task”: ”Turn on all lightswitches”,
”scene”: 1,
”initial room”: ”kitchen”,
”initial states”: [
{”id”: 71, ”states”: [”ON”]},
{”id”: 173, ”states”: [”OFF”]},
{”id”: 261, ”states”: [”ON”]},
{”id”: 427, ”states”: [”OFF”]}
],
”goal states”: [
{”id”: 71, ”states”: [”ON”]},
{”id”: 173, ”states”: [”ON”]},
{”id”: 261, ”states”: [”ON”]},
{”id”: 427, ”states”: [”ON”]}
],
”action scripts”: [
”[WALK] <lightswitch> (173)”,
”[SWITCHON] <lightswitch> (173)”,
”[WALK] <lightswitch> (427)”,
”[SWITCHON] <lightswitch> (427)”
]
}
5.3 Data Split
The state change task dataset contains 464 examples,
while the placement task dataset has 154 examples.
We split each dataset roughly in a 2:1 ratio between
testing and sample. The sample data is used to gener-
ate action steps as examples.
For state change tasks, 312 examples were allo-
cated for testing, and 152 for training. For placement
tasks, 103 examples were used for testing, and 51 for
training.
Each dataset treats tasks as distinct, even when
they share the same task description, due to variations
in the initial states of objects and VH scenes. Al-
though the data split was random, we ensured that ex-
amples with the same task description were not shared
between the sample and test sets. As a result, the split
may slightly vary from the exact 2:1 ratio.
5.4 Evaluation Methods
To begin, the VH home environment is configured us-
ing the dataset’s scene and initial state. The proposed
method then generates action plans based on the task
description provided in the dataset.
We experiment with two approaches for prompt-
ing the LLM to generate action steps. The first
method, “Single Prompt,” provides multiple compo-
nents of the prompts described in Figure 3 to the LLM
at once. The second method, “Multi Prompts,” gives
the contents step-by-step. Furthermore, an ablation
study is conducted to evaluate the effectiveness of the
Household Task Planning with Multi-Objects State and Relationship Using Large Language Models Based Preconditions Verification
479