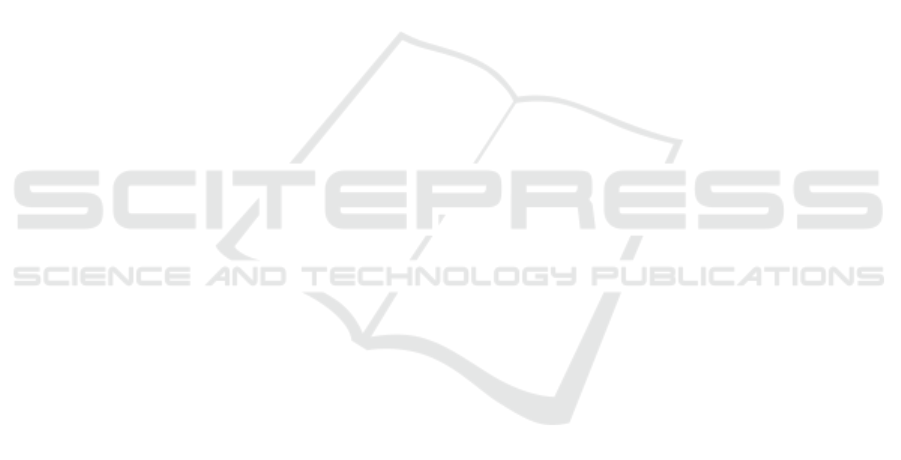
Chan, S., Li, J., Yao, B., Mahmood, A., Huang, C.-M., Jimi-
son, H., Mynatt, E. D., and Wang, D. (2023). ”mango
mango, how to let the lettuce dry without a spinner?”:
Exploring user perceptions of using an llm-based con-
versational assistant toward cooking partner.
Chen, D. (2023). Learning task preferences from real-world
data. Master’s thesis, Carnegie Mellon University,
Pittsburgh, PA.
de Haan, P., Jayaraman, D., and Levine, S. (2019). Causal
confusion in imitation learning.
Deshpande, M. and Karypis, G. (2004). Item-based top-n
recommendation algorithms. ACM Trans. Inf. Syst.,
22(1):143–177.
Funk, M., Mayer, S., and Schmidt, A. (2015). Using in-situ
projection to support cognitively impaired workers at
the workplace. In Proceedings of the 17th Interna-
tional ACM SIGACCESS Conference on Computers &
Accessibility, ASSETS ’15, page 185–192, New York,
NY, USA. Association for Computing Machinery.
Hamada, R., Okabe, J., Ide, I., Satoh, S., Sakai, S., and
Tanaka, H. (2005). Cooking navi: assistant for daily
cooking in kitchen. In Proceedings of the 13th Annual
ACM International Conference on Multimedia, MUL-
TIMEDIA ’05, page 371–374, New York, NY, USA.
Association for Computing Machinery.
Hu, Z., Lucchetti, F., Schlesinger, C., Saxena, Y., Freeman,
A., Modak, S., Guha, A., and Biswas, J. (2024). De-
ploying and evaluating llms to program service mo-
bile robots. IEEE Robotics and Automation Letters,
9(3):2853–2860.
Johansson, M. M., Marcusson, J., and Wressle, E. (2015).
Cognitive impairment and its consequences in every-
day life: experiences of people with mild cognitive
impairment or mild dementia and their relatives. In-
ternational Psychogeriatrics, 27(6):949–958.
Kojima, T., Gu, S. S., Reid, M., Matsuo, Y., and Iwasawa,
Y. (2023). Large language models are zero-shot rea-
soners.
Kosch, T., Wennrich, K., Topp, D., Muntzinger, M., and
Schmidt, A. (2019). The digital cooking coach: using
visual and auditory in-situ instructions to assist cog-
nitively impaired during cooking. In Proceedings of
the 12th ACM International Conference on PErvasive
Technologies Related to Assistive Environments, PE-
TRA ’19, page 156–163, New York, NY, USA. Asso-
ciation for Computing Machinery.
Le, D. M., Guo, R., Xu, W., and Ritter, A. (2023). Improved
instruction ordering in recipe-grounded conversation.
Liang, J., Huang, W., Xia, F., Xu, P., Hausman, K., Ichter,
B., Florence, P., and Zeng, A. (2023). Code as poli-
cies: Language model programs for embodied control.
Lin, K., Agia, C., Migimatsu, T., Pavone, M., and Bohg,
J. (2023). Text2motion: from natural language in-
structions to feasible plans. Autonomous Robots,
47(8):1345–1365.
McGrattan, A. M., McEvoy, C. T., McGuinness, B.,
McKinley, M. C., and Woodside, J. V. (2018). Ef-
fect of dietary interventions in mild cognitive impair-
ment: a systematic review. British Journal of Nutri-
tion, 120(12):1388–1405.
Nemlekar, H., Modi, J., Gupta, S. K., and Nikolaidis, S.
(2021). Two-stage clustering of human preferences
for action prediction in assembly tasks. In 2021 IEEE
International Conference on Robotics and Automation
(ICRA), pages 3487–3494.
Nikolaidis, S., Ramakrishnan, R., Gu, K., and Shah, J.
(2015). Efficient model learning from joint-action
demonstrations for human-robot collaborative tasks.
In Proceedings of the Tenth Annual ACM/IEEE In-
ternational Conference on Human-Robot Interaction,
HRI ’15, page 189–196, New York, NY, USA. Asso-
ciation for Computing Machinery.
OpenAI (2024). Gpt-4 technical report.
Padmanabha, A., Yuan, J., Gupta, J., Karachiwalla, Z.,
Majidi, C., Admoni, H., and Erickson, Z. (2024).
Voicepilot: Harnessing llms as speech interfaces
for physically assistive robots. arXiv preprint
arXiv:2404.04066.
Randers, I. and Mattiasson, A.-C. (2004). Autonomy
and integrity: upholding older adult patients’ dignity.
Journal of Advanced Nursing, 45(1):63–71.
Sanders, J. and Martin-Hammond, A. (2019). Exploring
autonomy in the design of an intelligent health assis-
tant for older adults. In Companion Proceedings of the
24th International Conference on Intelligent User In-
terfaces, IUI ’19 Companion, page 95–96, New York,
NY, USA. Association for Computing Machinery.
Sato, A., Watanabe, K., and Rekimoto, J. (2014). Mimi-
cook: a cooking assistant system with situated guid-
ance. TEI ’14, page 121–124, New York, NY, USA.
Association for Computing Machinery.
Singh, I., Blukis, V., Mousavian, A., Goyal, A., Xu, D.,
Tremblay, J., Fox, D., Thomason, J., and Garg, A.
(2022). Progprompt: Generating situated robot task
plans using large language models.
Tang, J. and Wang, K. (2018). Personalized top-n sequen-
tial recommendation via convolutional sequence em-
bedding. In Proceedings of the Eleventh ACM Inter-
national Conference on Web Search and Data Mining,
WSDM ’18, page 565–573, New York, NY, USA. As-
sociation for Computing Machinery.
Tuokko, H., Morris, C., and Ebert, P. (2005). Mild cognitive
impairment and everyday functioning in older adults.
Neurocase, 11(1):40–47. PMID: 15804923.
Wang, H., Gonzalez-Pumariega, G., Sharma, Y., and
Choudhury, S. (2023). Demo2code: From summariz-
ing demonstrations to synthesizing code via extended
chain-of-thought.
Wei, J., Wang, X., Schuurmans, D., Bosma, M., Ichter, B.,
Xia, F., Chi, E., Le, Q., and Zhou, D. (2023). Chain-
of-thought prompting elicits reasoning in large lan-
guage models.
Wherton, J. P. and Monk, A. F. (2010). Problems people
with dementia have with kitchen tasks: The challenge
for pervasive computing. Interacting with Computers,
22(4):253–266. Supportive Interaction: Computer In-
terventions for Mental Health.
Wu, J., Antonova, R., Kan, A., Lepert, M., Zeng, A., Song,
S., Bohg, J., Rusinkiewicz, S., and Funkhouser, T.
(2023). Tidybot: Personalized robot assistance with
large language models. Autonomous Robots.
ICAART 2025 - 17th International Conference on Agents and Artificial Intelligence
530