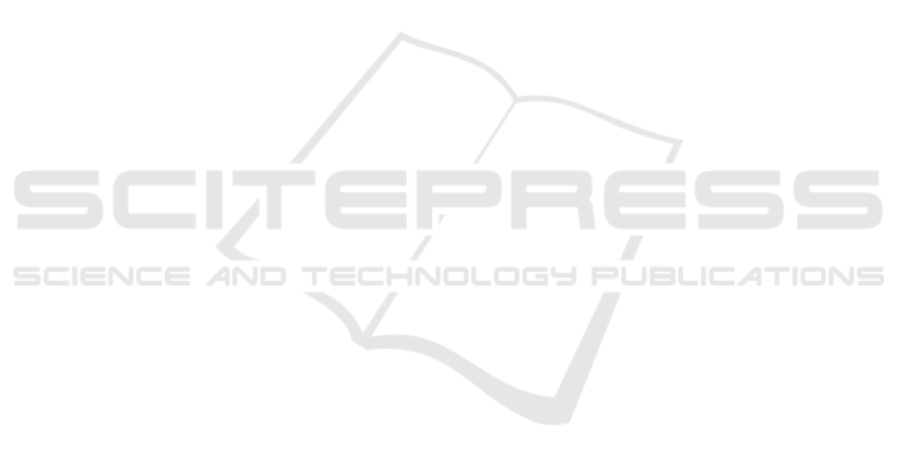
ited: The State of the Field in 2023. IEEE Computer
Graphics and Applications, pages 1–14.
Chernoff, H. (1973). The use of faces to represent points in
k-dimensional space graphically. Journal of the Amer-
ican statistical Association, 68(342):361–368.
Cunha, J. M., Polisciuc, E., Martins, P., and Machado, P.
(2018). The many-faced plot: strategy for automatic
glyph generation. In 2018 22nd International Con-
ference Information Visualisation (IV), pages 71–77.
IEEE.
Dragicevic, P. and Jansen, Y. (2012). List of physical vi-
sualizations. www.dataphys.org/list. Last accessed
2024-04-22.
Dumas, M., McGuffin, M. J., and Lemieux, V. L. (2014).
Financevis. net-a visual survey of financial data visu-
alizations. In Poster Abstracts of IEEE conference on
visualization, volume 2, page 8.
Figueiras, A. (2013). A Typology for Data Visualization on
the Web. In 2013 17th International Conference on
Information Visualisation, pages 351–358, London,
United Kingdom. IEEE.
Isaacs, K. E., Gim
´
enez, A., Jusufi, I., Gamblin, T., Bhatele,
A., Schulz, M., Hamann, B., and Bremer, P.-T. (2014).
State of the Art of Performance Visualization. EuroVis
- STARs, page 20 pages.
Jena, A., Engelke, U., Dwyer, T., Raiamanickam, V., and
Paris, C. (2020). Uncertainty Visualisation: An Inter-
active Visual Survey. In 2020 IEEE Pacific Visualiza-
tion Symposium (PacificVis), pages 201–205.
J.H., S., E.J., F., R.M., G., and H.P., F. (1972). The surgi-
cal implications of physiologic patterns in myocardial
infarction shock. pages 126–141.
Kehrer, J. and Hauser, H. (2013). Visualization and Vi-
sual Analysis of Multifaceted Scientific Data: A Sur-
vey. IEEE Transactions on Visualization and Com-
puter Graphics, 19(3):495–513.
Kerren, A., Kucher, K., Li, Y.-F., and Schreiber, F. (2017).
BioVis Explorer: A visual guide for biological data vi-
sualization techniques. PLOS ONE, 12(11):e0187341.
Kraus, M., Fuchs, J., Sommer, B., Klein, K., Engelke, U.,
Keim, D., and Schreiber, F. (2022). Immersive An-
alytics with Abstract 3D Visualizations: A Survey.
Computer Graphics Forum, 41(1):201–229.
Kucher, K. and Kerren, A. (2015). Text visualization tech-
niques: Taxonomy, visual survey, and community in-
sights. In 2015 IEEE Pacific Visualization Symposium
(PacificVis), pages 117–121.
Kucher, K., Paradis, C., and Kerren, A. (2018). The State of
the Art in Sentiment Visualization. Computer Graph-
ics Forum, 37(1):71–96.
Liu, S., Maljovec, D., Wang, B., Bremer, P.-T., and Pas-
cucci, V. (2017). Visualizing High-Dimensional Data:
Advances in the Past Decade. IEEE Transactions on
Visualization and Computer Graphics, 23(3):1249–
1268.
Lu, Y., Garcia, R., Hansen, B., Gleicher, M., and Ma-
ciejewski, R. (2017). The State-of-the-Art in Pre-
dictive Visual Analytics. Computer Graphics Forum,
36(3):539–562.
Page, M. J., McKenzie, J. E., Bossuyt, P. M., Boutron,
I., Hoffmann, T. C., Mulrow, C. D., Shamseer, L.,
Tetzlaff, J. M., Akl, E. A., Brennan, S. E., Chou,
R., Glanville, J., Grimshaw, J. M., Hr
´
objartsson, A.,
Lalu, M. M., Li, T., Loder, E. W., Mayo-Wilson, E.,
McDonald, S., McGuinness, L. A., Stewart, L. A.,
Thomas, J., Tricco, A. C., Welch, V. A., Whiting, P.,
and Moher, D. (2021). The prisma 2020 statement:
an updated guideline for reporting systematic reviews.
BMJ, 372.
Ramos, B. N., Mac¸
˜
as, C., Lourenc¸o, N., and Polisciuc, E.
(2023). Towards contextual glyph design: Visualizing
hearing screenings. In 2023 27th International Con-
ference Information Visualisation (IV), pages 96–102.
IEEE.
Schulz, H.-J. (2011). Treevis.net: A Tree Visualization Ref-
erence. IEEE Computer Graphics and Applications,
31(6):11–15.
Sch
¨
ottler, S., Yang, Y., Pfister, H., and Bach, B. (2021). Vi-
sualizing and Interacting with Geospatial Networks:
A Survey and Design Space. Computer Graphics Fo-
rum, 40(6):5–33.
Sperrle, F., El-Assady, M., Guo, G., Borgo, R., Chau, D. H.,
Endert, A., and Keim, D. (2021). A Survey of Human-
Centered Evaluations in Human-Centered Machine
Learning. Computer Graphics Forum, 40(3):543–568.
Tominski, C. and Aigner, W. (2023). The TimeViz Browser
– A Visual Survey of Visualization Techniques for
Time-Oriented Data. https://browser.timeviz.net. Ver-
sion 2.0.
Tsirikoglou, A., Eilertsen, G., and Unger, J. (2020). A Sur-
vey of Image Synthesis Methods for Visual Machine
Learning. Computer Graphics Forum, 39(6):426–451.
Wang, Q., Chen, Z., Wang, Y., and Qu, H. (2021). A sur-
vey on ml4vis: Applying machine learning advances
to data visualization. IEEE transactions on visualiza-
tion and computer graphics, 28(12):5134–5153.
Wang, Q. and Laramee, R. (2022). Ehr star: The state-
of-the-art in interactive ehr visualization. Computer
Graphics Forum, 41(1):69–105.
Ward, M. O. (2002). A taxonomy of glyph placement strate-
gies for multidimensional data visualization. Informa-
tion Visualization, 1(3-4):194–210.
Ward, M. O. (2008). Multivariate data glyphs: Principles
and practice. In Handbook of data visualization, pages
179–198. Springer.
Xu, K., Ottley, A., Walchshofer, C., Streit, M., Chang, R.,
and Wenskovitch, J. (2020). Survey on the Analysis of
User Interactions and Visualization Provenance. Com-
puter Graphics Forum, 39(3):757–783.
Yi, J. S., Kang, Y., Stasko, J., and Jacko, J. (2007). Toward
a Deeper Understanding of the Role of Interaction in
Information Visualization. IEEE Transactions on Vi-
sualization and Computer Graphics, 13:1224–1231.
Interactive Platform for Surveys in Information Visualisation
729