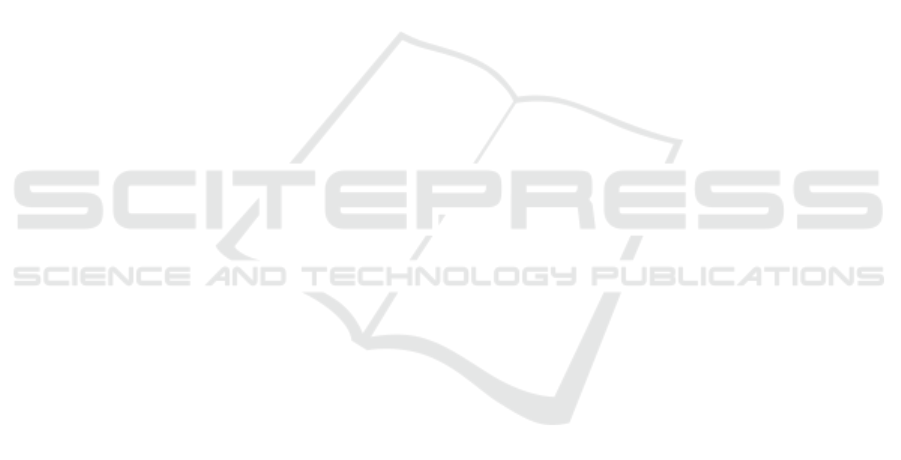
relies on the user’s abilities to review the visualiza-
tions. In future work, an automatic detection and
highlighting of these rhymes could be investigated.
Furthermore, our approach includes various pa-
rameters and our default configuration cannot be ideal
for every song. Users might be willing to try out dif-
ferent configurations through our setting panels, but
this can be time-consuming. Moreover, as shown in
Table 1, the system may incorrectly classify words
even when users have the expertise to understand and
configure the different options. Since there is not one
default best setting suitable for every song, future re-
search could try to optimize the default parameters by
determining them dynamically based on the charac-
teristics of the given song (e.g., through learning from
manually optimized examples).
While the detection quality and application exam-
ples presented in this paper demonstrate potential, a
user evaluation is still necessary to assess the usabil-
ity and usefulness of the visualization. However, a
traditional task-based study might be too limited, as
it would be difficult to specify meaningful, represen-
tative low-level tasks—how users would want to use
and interact with an explorative music visualization is
too open and likely individual. In contrast, a more
qualitative study methodology focusing on insights
and personally perceived value could provide more
relevant results.
8 CONCLUSIONS
With the goal to provide an automated visualization
approach to reflect on rhymes in rap music, we pre-
sented RapViz. It builds on a processing pipeline that
takes the song and its lyrics as input and provides dis-
tinct rhyme groups and text–audio synchronization as
output. To display the data, the approach connects
two main visualizations, one focusing on the lyrics,
the other one presenting a temporal perspective. In-
teractive playback with congruent animation provides
a basis for understanding rhyme patterns while listen-
ing. Further interactions and visual encodings also
support a retrospective analysis. The studied songs
give examples of findings and insights that lay listen-
ers can realistically discover using the approach, show
added potentials when optimizing the expert settings,
and reveal current limitations. While the approach
overall already provides results of good quality, we
see that future improvements can be made through an
AI-based audio analysis and parameter optimization,
as well as conducting in-depth user research.
REFERENCES
Abdul-Rahman, A., Lein, J., Coles, K., Maguire, E., Meyer,
M., Wynne, M., Johnson, C. R., Trefethen, A., and
Chen, M. (2013). Rule-based visual mappings – with a
case study on poetry visualization. Computer Graph-
ics Forum, 32(3pt4):381–390.
Benito-Santos, A., Mu
˜
noz, S., Ther
´
on S
´
anchez, R., and
Garc
´
ıa Pe
˜
nalvo, F. J. (2023). Characterizing the vi-
sualization design space of distant and close reading
of poetic rhythm. Frontiers in Big Data, 6:1167708.
Bergstrom, T., Karahalios, K., and Hart, J. (2007). Iso-
chords: visualizing structure in music. In Proceedings
of Graphics Interface 2007, pages 297–304. ACM.
Ciuha, P., Klemenc, B., and Solina, F. (2010). Visualization
of concurrent tones in music with colours. In Pro-
ceedings of the 18th ACM International Conference
on Multimedia, pages 1677–1680. ACM.
Clement, T., Tcheng, D., Auvil, L., Capitanu, B., and Mon-
roe, M. (2013). Sounding for meaning: Using theories
of knowledge representation to analyze aural patterns
in texts. Digital Humanities Quarterly, 7(1).
Dannenberg, R. and Raphael, C. (2006). Music score align-
ment and computer accompaniment. Communications
of the ACM, 49(8):38–43.
Delmonte, R. (2015). Visualizing poetry with SPARSAR
– visual maps from poetic content. In Proceedings
of the Fourth Workshop on Computational Linguistics
for Literature, pages 68–78. ACL.
Eastwood, J. and Hinton, E. (2016a). How does ‘Hamilton,’
the non stop, hip-hop Broadway sensation tap rap’s
master rhymes to blur musical lines? http://graphics.
wsj.com/hamilton/. Accessed: 2024-09-22.
Eastwood, J. and Hinton, E. (2016b). How WSJ used an
algorithm to analyze ‘Hamilton’ the musical. http://
graphics.wsj.com/hamilton-methodology. Accessed:
2024-09-22.
Edwards, P. (2009). How to rap. Chicago Review Press.
Encyclopaedia Britannica (2024). Assonance. https://www.
britannica.com/art/assonance. Accessed: 2024-09-22.
Goss, C. and Carson, J. (2016). A visual represen-
tation of Wagner’s music. https://wp.stolaf.edu/
dh/a-visual-representation-of-wagners-music/. Ac-
cessed: 2024-09-24.
Khulusi, R., Kusnick, J., Meinecke, C., Gillmann, C.,
Focht, J., and J
¨
anicke, S. (2020). A Survey on Visual-
izations for Musical Data. Computer Graphics Forum,
39(6):82–110.
Lein, J. G., Mccurdy, N., and Hurtado, A. (2018). Deep
in poetry: Visualizing texts’ sonic depths in 3D.
Leonardo, 51(1):80–82.
McCurdy, N., Lein, J., Coles, K., and Meyer, M. (2016).
Poemage: Visualizing the sonic topology of a poem.
IEEE Transactions on Visualization and Computer
Graphics, 22(1):439–448.
Meinecke, C., Hakimi, A. D., and J
¨
anicke, S. (2022a). Ex-
plorative visual analysis of rap music. Information,
13(1):10.
IVAPP 2025 - 16th International Conference on Information Visualization Theory and Applications
738