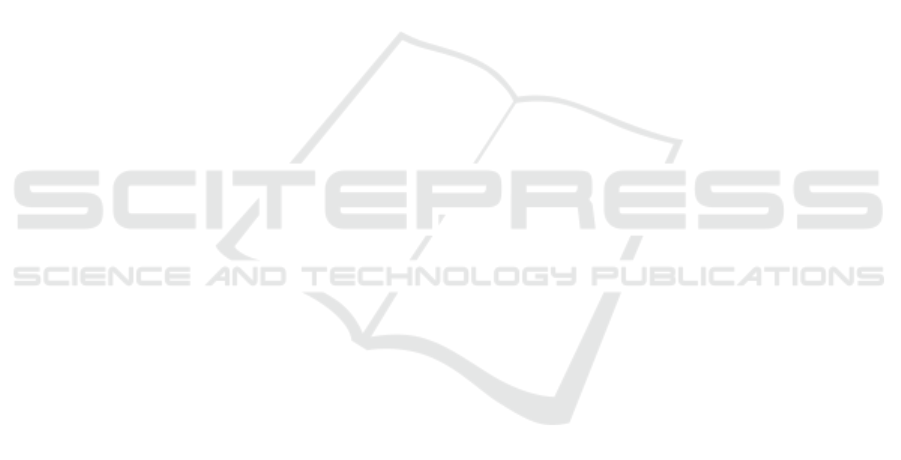
our approach involves less computationally inten-
sive operations while still maintaining a high level
of security. Overall, our obfuscation approach of-
fers a balanced solution, prioritizing security without
compromising on performance or resource efficiency.
This makes it a viable alternative for various deploy-
ment scenarios, including resource-constrained envi-
ronments where minimizing computational and mem-
ory overhead is crucial.
4 RELATED WORKS
Several mechanisms for privacy-preserving data be-
fore cloud deployment are proposed in the literature,
often focusing on data encryption. However, encryp-
tion, including searchable and homomorphic encryp-
tion, can hinder cloud processing due to its perfor-
mance limitations and high computational cost. These
encryption techniques also require careful key man-
agement, which can introduce further complexity.
Alternatively, hybrid methods, such as the com-
bination of AES and FHE (Kumar and Badal, 2019),
offer data security while maintaining operational ef-
ficiency. Muralidhar et al. (Muralidhar and Sarathy,
2006) introduced data shuffling to mask confidential
numerical data, enhancing utility while minimizing
disclosure risks. Similarly, V Ciriani et al. (Ciriani
et al., 2010) proposed a method that combines data
fragmentation and encryption to protect sensitive in-
formation.
Other approaches focus on data partitioning and
distribution across multiple cloud providers. Jensen
et al. (Jensen et al., 2011) suggested using multiple
clouds to reduce the risk of data manipulation and
tampering. Zhang et al. (Zhang et al., 2013) and
Celesti et al. (Celesti et al., 2016) explored split-
ting data into parts and distributing them across dif-
ferent clouds, improving security by minimizing ex-
posure. However, these approaches often overlook the
potential for data reconstruction through conspiracies
among cloud providers.
5 CONCLUSION
To ensure the protection of business strategies and
sensitive data before cloud deployment, we intro-
duced an innovative approach that combines process
fragmentation with dynamic obfuscation. This sec-
ond level of obfuscation involves randomly selecting
techniques at each execution, making it harder for at-
tackers to reverse engineer the data.
Our evaluation demonstrates the effectiveness of
this approach in obscuring sensitive information,
as indicated by low similarity indices between ob-
fuscated and deobfuscated data. Additionally, our
method outperforms traditional cryptographic algo-
rithms in terms of execution time, CPU usage, and
memory usage, highlighting its efficiency.
Future work will focus on enhancing the adapt-
ability and resilience of our approach to emerging se-
curity threats and refining evaluation metrics to assess
obfuscation techniques more comprehensively.
DECLARATIONS
The authors have no competing interests to declare
that are relevant to the content of this article.
REFERENCES
Alliance, C. (2014). Csa security, trust & assurance registry
(star). visited on, pages 08–18.
Aron, R., Clemons, E. K., and Reddi, S. (2005). Just right
outsourcing: Understanding and managing risk. Jour-
nal of management information systems, 22(2):37–55.
Breiman, L. (2017). Classification and regression trees.
Routledge.
Celesti, A., Fazio, M., Villari, M., and Puliafito, A. (2016).
Adding long-term availability, obfuscation, and en-
cryption to multi-cloud storage systems. Journal of
Network and Computer Applications, 59:208–218.
Ciriani, V., Vimercati, S. D. C. D., Foresti, S., Jajodia,
S., Paraboschi, S., and Samarati, P. (2010). Combin-
ing fragmentation and encryption to protect privacy in
data storage. ACM Transactions on Information and
System Security (TISSEC), 13(3):1–33.
Devlin, J., Chang, M.-W., Lee, K., and Toutanova, K.
(2018). Bert: Pre-training of deep bidirectional trans-
formers for language understanding. arXiv preprint
arXiv:1810.04805.
ENISA, C. C. (2009). Benefits, risks and recommendations
for information security. European Network and In-
formation Security, 23.
Goettelmann, E., Ahmed-Nacer, A., Youcef, S., and Godart,
C. (2015). Paving the way towards semi-automatic
design-time business process model obfuscation. In
2015 IEEE International Conference on Web Services,
pages 559–566. IEEE.
Hastie, T., Tibshirani, R., Friedman, J. H., and Friedman,
J. H. (2009). The elements of statistical learning: data
mining, inference, and prediction, volume 2. Springer.
Jamil, T. (2004). The rijndael algorithm. IEEE potentials,
23(2):36–38.
Jensen, M., Schwenk, J., Bohli, J.-M., Gruschka, N., and
Iacono, L. L. (2011). Security prospects through
cloud computing by adopting multiple clouds. In 2011
Dynamic Obfuscation for Secure and Efficient Multi-Cloud Business Processes
181