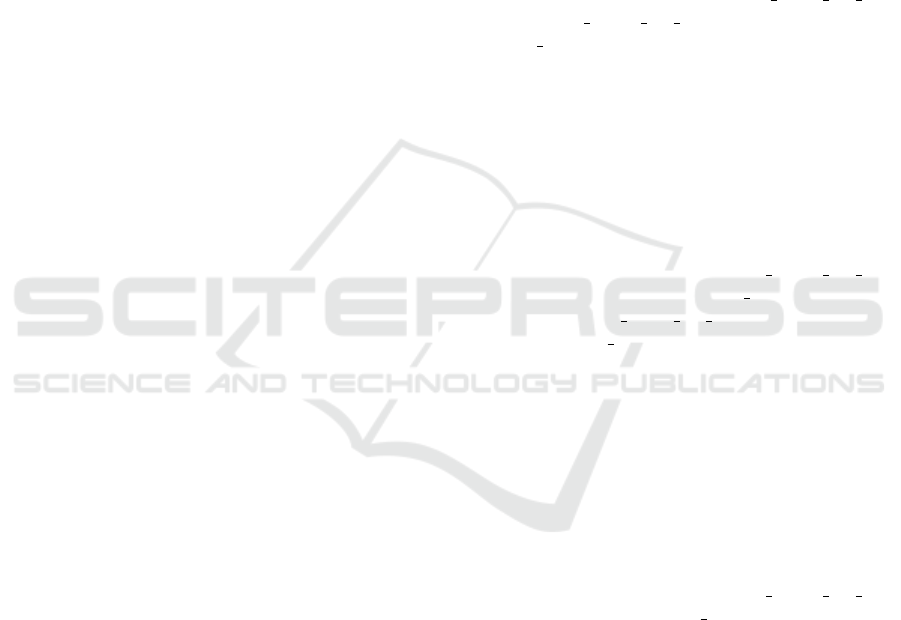
Examples are provided across different scenarios,
classes, and datasets. Consider the query with input
parameters [Sun: 1, Wind: 1, Sore Knee: 0], or, for
a more detailed representation [Sun: 1, No Sun: 0,
Wind: 1, No Wind: 0, Sore Knee: 0, Good Knee:
1]. For this query, all seven algorithms unanimously
predicted class 1, representing the Surf activity.
Given the size of the database, only two extensions
were identified as preferred extensions. Among
these, one extension had a uniform score of 1 for
its arguments, whereas the other achieved a score
of 10 for all its arguments. The standout argument
was Argument 16, which is arg16, Premise: ’Wind’,
’Good Knee’, Conclusion: ’Surf’, achieving a score
of 3. This score was attributed to its perfect alignment
with the query (+1), the presence of two elements
in the premise (+1), and its conclusion matching
the predicted class (Surf). This argument logically
correlates with the database, indicating that windy
conditions and the absence of knee soreness, rather
than sunny weather, influenced the ML algorithm’s
prediction favoring Surf as the activity. Had the ML
classification model predicted Fishing for the same
query, a completely different set of arguments and
extensions would have emerged, such as Argument
1, with a premise of ’Fishing’ leading to a conclusion
of ’Sun’, thereby attributing the sunny condition as
a decisive factor in predicting Fishing, according to
XARF’s explanation. Examining a query that elicited
split predictions from the ML classifiers [Sun: 0,
Wind: 1, Sore Knee: 1], or more succinctly: [Sun:
0, No Sun: 1, Wind: 1, No Wind: 0, Sore Knee:
1, Good Knee: 0]. The majority of classifiers (5
out of 7) favored class 0 (Fishing), while Random
Forest and Naive Bayes opted for class 1 (Surf).
For models predicting Fishing, the most robust
extension featured a score of 5, with the higher score
argument, arg9, Premise: ’Sore Knee’, Conclusion:
’Fishing’ with a score 2, indicating that a sore knee
is a deterrence from surfing. Additional arguments
in this extension included correlations between the
absence of sun and wind, and a sore knee, further
supporting the Fishing prediction. Conversely, for
models predicting Surfing, the leading extension
scored 13, highlighted by arg12, Premise: ’No Sun’,
’Wind’, Conclusion: ’Surf’. This implies that the
lack of sunshine combined with windy conditions
were considered significant by the classifiers for a
Surf prediction. These explanations, coherent with
both the database content and the predicted classes,
underscore the capability of XARF to generate
plausible explanations, even when classifiers diverge
in their predictions for the same query. Relating
to the experiments in the Iris dataset, upon the
implementation of the Apriori algorithm and the
formulation of attack relations, the argumentation
framework (AF) for the Iris dataset was meticulously
constructed. Analogously to the Boolean dataset, we
herein exhibit examples of explanations generated
by XARF across different scenarios. Consider a
query with the following characteristics: Sepal length
(cm): 5.4, Sepal width (cm): 3.7, Petal length (cm):
1.5, Petal width (cm): 0.2. For this dataset, all
seven ML predictors accurately classified the query
as class 0, corresponding to the Setosa species.
Among the extensions evaluated, one with a score
of 12 was selected, prominently featuring Argument
5 which is arg5, Premise: ’petal width bin (0.1,
0.5]’, ’petal length bin (1.0, 2.0]’, Conclusion:
’species setosa’. This argument, which aligns with
two premises and the conclusion being the predicted
class, received a score of 3. It underscores the
importance of both petal length and width in deter-
mining the Setosa classification. Another query is
examined: Sepal length (cm): 7.0, Sepal width (cm):
3.2, Petal length (cm): 4.7, Petal width (cm): 1.4.
Here, all algorithms concurred on class 1, Versicolor.
The chosen extension scored 4, highlighting two
arguments, Argument 2 and Argument 3, as equally
significant: arg2, Premise: ’petal length bin (4.0,
5.0]’, Conclusion: ’species versicolor’ arg3,
Premise: ’petal width bin (1.0, 1.5]’, Conclu-
sion: ’species versicolor’ Both arguments are
consistent with the query and show that petal sizes
were the most important attributes for the decision
of ML to assign class Versicolor. A contentious
example involves the query: Sepal length (cm): 5.9,
Sepal width (cm): 3.2, Petal length (cm): 4.8, Petal
width (cm): 1.8. Here, a split in predictions occurred:
Naive Bayes, Logistic Regression, KNN, and Neural
Networks opted for class 2, while Decision Tree,
Random Forest, and SVM selected class 1. For
predictions of class 1, XARF highlighted Argument
2, which is arg2, Premise: ’petal length bin (4.0,
5.0]’, Conclusion: ’species versicolor’, as the sole
explanatory factor with a score of 2. Conversely,
for class 2 predictions, the framework found no
supporting arguments, resulting in an extension score
of zero. Although no explanation was discovered in
this particular instance, the outcome aligns with the
dataset and the predicted class. This underscores the
integrity of the framework, as it avoids creating ex-
planations in the absence of sufficient evidence. The
challenge presented by the query, which divided the
”opinion” of the ML algorithms, highlights its com-
plexity and the difficulty in generating explanations
for such cases. Nevertheless, there is a requirement
for a broader spectrum of arguments and, conse-
ICEIS 2025 - 27th International Conference on Enterprise Information Systems
688