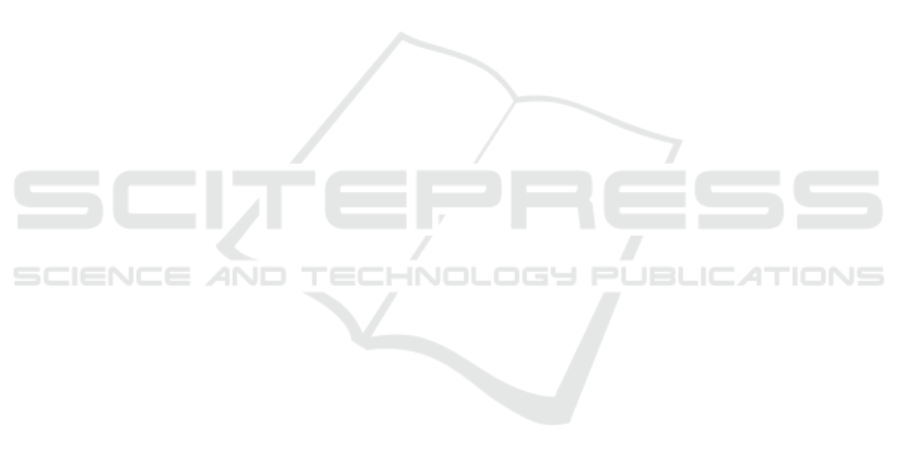
Our primary contributions are as follows:
• We compiled a comprehensive dataset containing
over 139,000 traffic snapshots collected from all
divisions of Bangladesh over six months (January
2023 to June 2023) using Google Maps and image
processing techniques.
• A data modification method was proposed to en-
hance clustering accuracy by eliminating random
noise from the traffic intensity data, thereby im-
proving the quality of the clustering process.
• We employed a modified hierarchical clustering
algorithm, using DTW as the distance metric in-
stead of traditional Euclidean distance. This ap-
proach significantly improved the alignment of
traffic patterns over time, capturing similarities
between traffic patterns.
This research offers insights into regional traffic
patterns and provides a framework for more effective
traffic management strategies. It enables urban plan-
ners to design tailored congestion mitigation policies
for different areas of Bangladesh. This study inte-
grates advanced clustering techniques with spatiotem-
poral analysis, offering a nuanced understanding of
traffic congestion in rapidly urbanizing contexts like
Bangladesh.
2 RELATED WORK
Urban traffic congestion has been extensively stud-
ied due to its significant impact on transportation ef-
ficiency, economic costs, and quality of life. Re-
searchers have employed various data-driven and
machine-learning methodologies to analyze and man-
age traffic congestion patterns, aiming to develop
effective strategies for urban traffic management.
Xiong introduced an innovative method using Dy-
namic Time Warping (DTW) to detect spatiotempo-
ral propagation patterns of traffic congestion (Xiong
et al., 2023). Analyzing fine-grained vehicle tra-
jectory data reveals how localized congestion events
can propagate across road networks, providing new
insights for managing urban traffic systems. Simi-
larly, Chen employed taxi trajectory data to model
the spread of traffic congestion across neighboring
road segments, offering a method for anticipating and
mitigating congestion through effective traffic control
measures (Chen et al., 2018). Zang applied a self-
organizing map (SOM) to cluster traffic congestion
patterns based on the Traffic Performance Index (TPI)
in Beijing (Zang et al., 2023). The study identified
specific congestion patterns for weekdays, weekends,
and holidays, providing a temporal perspective on
traffic management and policy planning. Kanchana-
mala explored Hadoop-based hierarchical clustering
for large-scale traffic data analysis, demonstrating
how big data analytics can improve the scalability and
efficiency of traffic monitoring and management in
megacities (Kanchanamala et al., 2016). Amb
¨
uhl fur-
ther contributed by analyzing macroscopic fundamen-
tal diagrams (MFDs) to track urban traffic rhythms
over time, providing insights into long-term traffic
management strategies (Amb
¨
uhl et al., 2021). Wang
proposed a Spatio-Temporal Non-Negative Matrix
Factorization (ST-NMF) approach to address the chal-
lenges of analyzing noisy, high-dimensional data in
large-scale urban networks (Wang et al., 2021). ST-
NMF enhances traffic data reconstruction and pre-
dicts future traffic states by decomposing traffic states
into spatial and temporal patterns. This approach pro-
vides a robust framework for managing intelligent
transportation systems through a clearer understand-
ing of spatio-temporal traffic dynamics. Akbar con-
ducted a comprehensive analysis of traffic speeds in
1,200 cities across 152 countries, revealing that cities
in more affluent countries tend to have faster travel
speeds due to their larger urban areas and more ex-
tensive road infrastructure (Akbar et al., 2023b). The
study found that uncongested speed, rather than con-
gestion reduction, is the primary driver of faster travel
speeds in wealthier countries. This finding under-
scores the importance of infrastructure investment in
improving urban mobility. Li employed a weighted
K-means clustering method to analyze traffic conges-
tion patterns in Beijing, focusing on the effects of ur-
ban policies such as vehicle license plate restrictions
(Li et al., 2023). Their study illustrates the poten-
tial of big data analytics for identifying spatial and
temporal congestion patterns across different city dis-
tricts, contributing valuable insights for traffic man-
agement strategies. Akbar investigated traffic conges-
tion in Indian cities using simulated trip data, finding
that uncongested speed plays a more significant role
than congestion in determining travel speed differ-
ences across cities (Akbar et al., 2023a) . This chal-
lenges conventional beliefs that traffic management
efforts should focus primarily on reducing conges-
tion instead of emphasizing the need for infrastruc-
ture development. In the context of Bangladesh, our
study builds upon these methodologies by employing
a hierarchical clustering approach combined with Dy-
namic Time Warping (DTW) to analyze urban traf-
fic patterns. This research collected traffic intensity
data using Google Maps data and image processing
techniques across all divisions of Bangladesh, identi-
fying four distinct traffic clusters. By enhancing the
clustering accuracy with a noise reduction technique,
VEHITS 2025 - 11th International Conference on Vehicle Technology and Intelligent Transport Systems
320