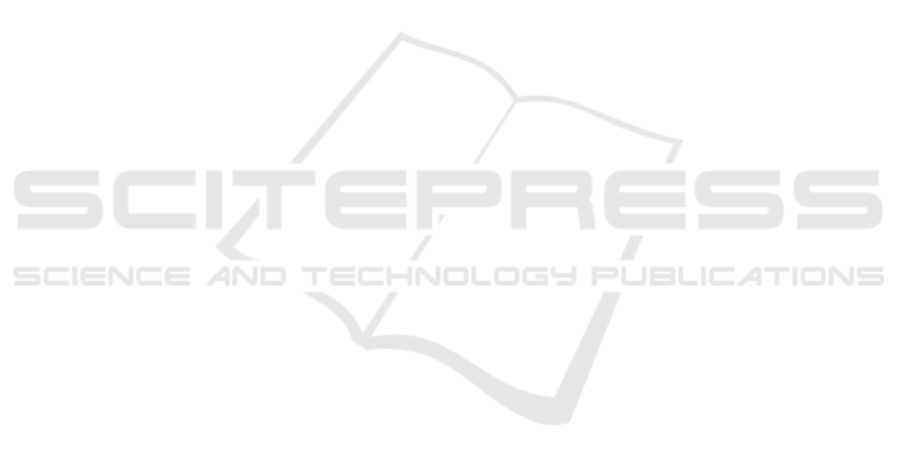
• Virtual Banking as a Fully Decentralized
Ecosystem: Transform into a fully decen-
tralized virtual banking ecosystem where cus-
tomers experience seamless, data-driven ser-
vices without reliance on centralized infrastruc-
ture.
3.3 Implement Difficulties
In the migration framework, the organization would
be in a state that is close to the opposite of the native
framework. Its key obstacles would be highly con-
trolled legacy legal contracts and conservative con-
trol processes, which highly introduce time and re-
sources to the data-sharing process. Also, the data
model might not be ready for data sharing. The key
strong advantage of this would also be a large amount
of data, in terms of the number of customers and rich-
ness of customer behavior to the organization. The
key focus on driving open data for migration organi-
zations should be on bringing high-impact use cases,
especially on data sharing with other big players from
other industries. This would drive the usage and mi-
gration to be faster, especially from business impact.
In the transformation approach, which focuses on
the transformation of the organization in parallel with
the revamping data model and stack, the key advan-
tage would be that the early adopters in organizations
are graving for the new business impact, which also
includes an open data use case. The key principles
that would help drive this would be focusing on No-
bel solutions and use cases by drawing the advantage
of a huge legacy number of customers. The concerns
that organizations should be aware of the matrix pro-
cess in evaluating cases
4 CONCLUSION
We have highlighted the critical role of data strategy
in the successful implementation and operation of vir-
tual banking. As virtual banks operate entirely on-
line, they require a robust data infrastructure capable
of handling real-time transactions, customer data, and
service requests around the clock. A well-structured
data strategy focusing on scalability, real-time analyt-
ics, and customer-centricity is essential to meet the
growing demand for instant and personalized finan-
cial services. By leveraging cutting-edge technolo-
gies like AI and big data analytics, virtual banks can
offer tailored financial products, enhance fraud detec-
tion, and improve operational efficiency compared to
their traditional banking counterparts.
REFERENCES
Analytica, O. (2020). Singapore will lead in south-east
asian fintech. Emerald Expert Briefings, (oxan-db).
Boshkov, T. (2019). Virtual banking and financial inclusion.
Chaimaa, B., Najib, E., and Rachid, H. (2021). E-banking
overview: concepts, challenges and solutions. Wire-
less Personal Communications, 117:1059–1078.
Curtis, H., Hogeveen, B., Kang, J., Le Thu, H., Ra-
jagopalan, R. P., and Ray, T. (2022). Digital southeast
asia. Australian Strategic Policy Institute.
Dehghani, Z. (2019). How to move beyond a monolithic
data lake to a distributed data mesh. Martin Fowler
Website.
Dolhopolov, A., Castelltort, A., and Laurent, A. (2024). Im-
plementing federated governance in data mesh archi-
tecture. Future Internet, 16(4):115.
Hadi, A. and Hmood, S. (2020). Analysis of the role of
digital transformation strategies in achieving the edge
of financial competition. 10:19–40.
Kraiwanit, T., Shaengchart, Y., Limna, P., Thetlek, R., and
Moolngearn, P. (2024). The strategy of virtual bank-
ing adoption in the digital economy. Thetlek, R., Krai-
wanit, T., Limna, P., Shaengchart, Y., & Moolngearn,
P.(2024). The strategy of virtual banking adoption
in the digital economy [Special issue]. Corporate &
Business Strategy Review, 5(1):264–272.
Lehmann, M. (2020). Global rules for a global mar-
ket place?-regulation and supervision of fintech
providers. BU Int’l LJ, 38:118.
Machado, I. A., Costa, C., and Santos, M. Y. (2022). Ad-
vancing data architectures with data mesh implemen-
tations. In Lecture Notes in Business Information Pro-
cessing, pages 34–49. Springer, Cham.
Mori, M. (2021). Ai-powered virtual assistants in the realms
of banking and financial services. Virtual assistant,
1:65–93.
Nguyen, T. V. and McCahery, J. (2020). Virtual bank
by fintech firms–global trending, challenges, solutions
and experience of regulating virtual banks in vietnam.
Technical report, Working Paper. Tilburg University,
the Netherlands.
Nian, L. P. and Chuen, D. L. K. (2024). A light touch of reg-
ulation for virtual currencies. In Handbook of Digital
Currency, pages 291–308. Elsevier.
Oruganti, S. C. (2020). Virtual bank assistance: An ai based
voice bot for better banking. International Journal of
Research, 9(1):177–183.
Schatt, D. (2014). Virtual banking: a guide to innovation
and partnering. John Wiley & Sons.
Wewege, L., Lee, J., and Thomsett, M. C. (2020). Disrup-
tions and digital banking trends. Journal of Applied
Finance and Banking, 10(6):15–56.
Windasari, N. A., Kusumawati, N., Larasati, N., and
Amelia, R. P. (2022). Digital-only banking experi-
ence: Insights from gen y and gen z. Journal of Inno-
vation & Knowledge, 7(2):100170.
CLOSER 2025 - 15th International Conference on Cloud Computing and Services Science
118