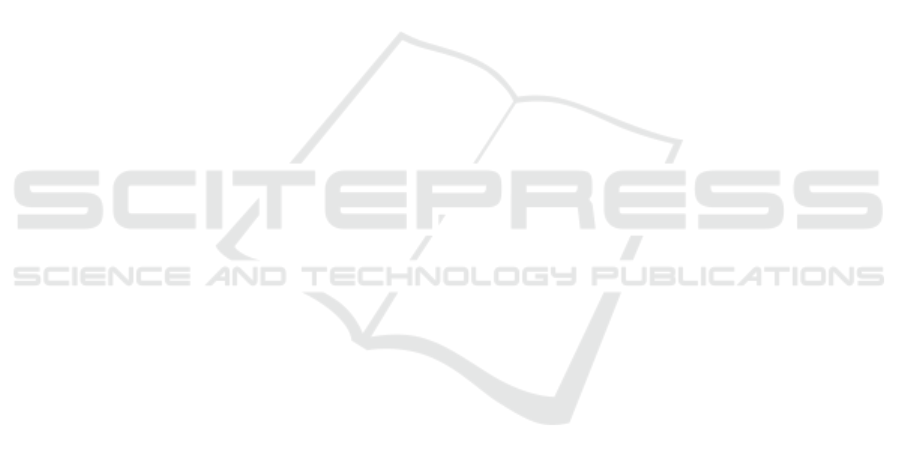
some of the decision trees that control the affected
processes—thus modifying the application.
Except for these rare exceptions, most ADAclas-
sifier’s results are reliable. However, verification by
an expert will always be recommended to ensure that
situations like the one just described do not go unno-
ticed.
3 CONCLUSION
Despite being available since 2018 as an integral part
of the ADAtools, the original version of ADAclassi-
fier has not been regularly used to attempt to deter-
mine the causes of ground movement due to this tool’s
performance issues but, primarily, because of the low
reliability of the results offered, which were obtained
by means of overly simplistic algorithms.
Recently, and within the framework of the RAS-
TOOL project, a great effort has been made to identify
the reasons why the five analyzed ground movement
processes occur. Ultimately, this effort was aimed
at improving the understanding of these processes,
which has resulted in a set of detection algorithms
closer to reality, materialized in the aforementioned
decision trees.
ADAclassifier is not yet a mature tool, but today
it is much closer to being able to be exploited regu-
larly in expert environments not necessarily involved
in research. It is true that notable defects have still
been detected, such as the already mentioned land-
slide/uplift dichotomy, but this is one more step to be
solved like the many others that have been overcome
to reach the current state of ADAclassifier. The avail-
ability of a large volume of EGMS data making possi-
ble the processing of ADAs at a national level opened
the doors to an extensive validation task, thanks to
which the authors are reasonably satisfied with the re-
liability of the results produced by tool.
Perhaps it is adventurous to advance a date on
which ADAclassifier can be considered a production
tool (in expert environments), but the authors consider
that, despite the aforementioned defects, that moment
is not far off. Probably this will happen by the end of
2024 or the beginning of 2025.
ADAclassifier is part of the ADAtools, which can
be downloaded for free at https://adatools.cttc.es.
ACKNOWLEDGMENTS
This work is part of the Spanish grant
SARAI, PID2020-116540RB-C21, funded by
MCIN/AEI/10.13039/501100011033.
REFERENCES
Barra, A., Solari, L., B
´
ejar-Pizarro, M., Monserrat, O.,
Bianchini, S., Herrera, G., Crosetto, M., Sarro, R.,
Gonz
´
alez-Alonso, E., Mateos, R. M., Lig
¨
uerzana, S.,
L
´
opez, C., and Moretti, S. (2017). A methodology to
detect and update active deformation areas based on
Sentinel-1 SAR images. Remote Sens., 9(10).
EGMS (2017). European Ground Motion Service (EU-
GMS) - A proposed Copernicus service element.
White paper, European Ground Motion Service.
Gasc-Barbier, M., Barra, A., Bux
´
o, P., Trapero, L.,
Crosetto, M., Colell, X., Fabregat, I., Echeverria,
A., and Marturia, J. (2021). Monitoring deforma-
tions related to geological risks with InSaR data – the
MOMPA project. IOP Conference Series: Earth and
Environmental Science, 833(1):012142.
Montserrat, O., Barra, A., B
´
ejar-Pizarro, M., Rivera, J.,
Galve, J. P., Guardiola, C., Cuevas-Gonz
´
alez, M., Ma-
teos, R. M., Ezquerro, P., Aza
˜
n
´
on, J. M., Shahbazi, S.,
Navarro, J., Crosetto, M., and Luzi, G. (2024). ADA-
tools: herramientas gratuitas y f
´
aciles de usar para
extraer y analizar semiautom
´
aticamente mapas de de-
splazamiento basados en interferometr
´
ıa multitempo-
ral. aplicaciones al European Ground Motion Service
(EGMS). In Proceedings of XX Congreso de la Aso-
ciaci
´
on Espa
˜
nola de Teledetecci
´
on.
Navarro, J., Garc
´
ıa, D., and Crosetto, M. (2023). New
challenges in the implementation and exploitation of
a low-cost web map of the Active Deformation Ar-
eas across Europe. In Proceedings of the 9th Interna-
tional Conference on Geographical Information Sys-
tems Theory, Applications and Management - Volume
1: GISTAM,, pages 192–199.
Navarro, J. A. (2024a). ADAclassifier’s user guide. In-
cluded in the ADAtools software package. Available
at: https://adatools.cttc.es.
Navarro, J. A. (2024b). ADAfinder’s user guide. In-
cluded in the ADAtools software package. Available
at: https://adatools.cttc.es.
Navarro, J. A., Garc
´
ıa, D., and Crosetto, M. (2022). An
in-house, self-developed low-cost WebGIS relying on
open-source and self-made tools to show the Active
Deformation Areas across Europe. In Proceedings of
the 8th International Conference on Geographical In-
formation Systems Theory, Applications and Manage-
ment.
Navarro, J. A., Tom
´
as, R., Barra, A., Pag
´
an, J. I., Reyes-
Carmona, C., Solari, L., Vinielles, J. L., Falco, S., and
Crosetto, M. (2020). ADAtools: Automatic detection
and classification of Active Deformation Areas from
PSI displacement maps. ISPRS Int. J. Geo-Inf., 9:584.
RASTOOL project (2024). RASTOOL.
https://rastool.cttc.es. Accessed on October 25,
2024.
Solari, L., Bianchini, S., Franceschini, R., Barra, A., Mon-
serrat, O., Thuegaz, P., Bertolo, D., Crosetto, M., and
Catani, F. (2020). Satellite interferometric data for
landslide intensity evaluation in mountainous regions.
Int. J. Appl. Earth Obs. Geoinf., 87.
GISTAM 2025 - 11th International Conference on Geographical Information Systems Theory, Applications and Management
134