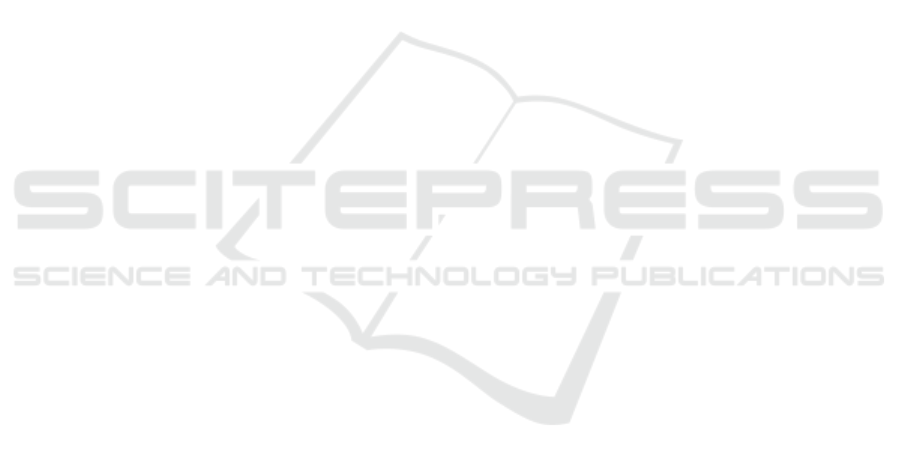
ACKNOWLEDGEMENTS
This work was supported in part by the Macao
Polytechnic University – Research on Representation
Learning in Decision Support for Medical Diagnosis
(RP/FCA-11/2022).The submission of this research
is controlled by the Macau Polytechnic University
(fca.65b5.d61c.2).
REFERENCES
Bai, L., Li, Q., Wang, J., Lavigne, E., Gasparrini, A., Copes,
R., Yagouti, A., Burnett, R. T., Goldberg, M. S., Cak-
mak, S., et al. (2018). Increased coronary heart dis-
ease and stroke hospitalisations from ambient temper-
atures in ontario. Heart, 104(8):673–679.
Bollepalli, S. C., Sahani, A. K., Aslam, N., Mohan, B.,
Kulkarni, K., Goyal, A., Singh, B., Singh, G., Mit-
tal, A., Tandon, R., et al. (2022). An optimized ma-
chine learning model accurately predicts in-hospital
outcomes at admission to a cardiac unit. Diagnostics,
12(2):241.
Chen, T., Madanian, S., Airehrour, D., and Cherrington, M.
(2022). Machine learning methods for hospital read-
mission prediction: systematic analysis of literature.
Journal of Reliable Intelligent Environments, 8(1):49–
66.
Cox, D. R. (1972). Regression models and life-tables. Jour-
nal of the Royal Statistical Society: Series B (Method-
ological), 34(2):187–202.
Davis, S., Mietlowski, W., Rohwedder, J. J., Griffin, J. P.,
and Neshat, A. A. (1982). Levamisole as an adju-
vant to chemotherapy in extensive bronchogenic car-
cinoma. a veterans administration lung cancer group
study. Cancer, 50(4):646–651.
Gensheimer, M. F. and Narasimhan, B. (2019). A scal-
able discrete-time survival model for neural networks.
PeerJ, 7:e6257.
Goodfellow, I., Warde-Farley, D., Mirza, M., Courville, A.,
and Bengio, Y. (2013). Maxout networks. In in ICML,
pages 1319–1327. PMLR.
Harrell, F. E., Califf, R. M., Pryor, D. B., Lee, K. L., and
Rosati, R. A. (1982). Evaluating the yield of medical
tests. Jama, 247(18):2543–2546.
Heidenreich, P. A., Bozkurt, B., Aguilar, D., Allen, L. A.,
Byun, J. J., Colvin, M. M., Deswal, A., Drazner,
M. H., Dunlay, S. M., Evers, L. R., et al. (2022).
2022 aha/acc/hfsa guideline for the management of
heart failure: a report of the american college of car-
diology/american heart association joint committee on
clinical practice guidelines. Journal of the American
College of Cardiology, 79(17):e263–e421.
Ishikawa, S. A., Zhukova, A., Iwasaki, W., and Gascuel, O.
(2019). A fast likelihood method to reconstruct and
visualize ancestral scenarios. Molecular biology and
evolution, 36(9):2069–2085.
Ishwaran, H., Kogalur, U. B., Blackstone, E. H., and Lauer,
M. S. (2008). Random survival forests.
Joglar, J. A., Chung, M. K., Armbruster, A. L., Benjamin,
E. J., Chyou, J. Y., Cronin, E. M., Deswal, A., Eck-
hardt, L. L., Goldberger, Z. D., Gopinathannair, R.,
et al. (2024). 2023 acc/aha/accp/hrs guideline for the
diagnosis and management of atrial fibrillation: a re-
port of the american college of cardiology/american
heart association joint committee on clinical practice
guidelines. Circulation, 149(1):e1–e156.
Kaplan, E. L. and Meier, P. (1958). Nonparametric esti-
mation from incomplete observations. Journal of the
American statistical association, 53(282):457–481.
Katzman, J. L., Shaham, U., Cloninger, A., Bates, J., Jiang,
T., and Kluger, Y. (2018). Deepsurv: personalized
treatment recommender system using a cox propor-
tional hazards deep neural network. BMC medical re-
search methodology, 18:1–12.
Lee, C., Zame, W., Yoon, J., and Van Der Schaar, M. (2018).
Deephit: A deep learning approach to survival analy-
sis with competing risks. In in AAAI.
Mi
ˇ
si
´
c, V. V., Gabel, E., Hofer, I., Rajaram, K., and Maha-
jan, A. (2020). Machine learning prediction of postop-
erative emergency department hospital readmission.
Anesthesiology, 132(5):968–980.
Model, C. P. H. (2017). Multi-task cox proportional hazard
model for predicting risk of unplanned hospital read-
mission. 2017 Systems and Information Engineering
Design Symposium (SIEDS), pages 265–270.
Pan, W.-H., Li, L.-A., and Tsai, M.-J. (1995). Temperature
extremes and mortality from coronary heart disease
and cerebral infarction in elderly chinese. The Lancet,
345(8946):353–355.
Pepe, M. S. and Fleming, T. R. (1989). Weighted kaplan-
meier statistics: a class of distance tests for censored
survival data. Biometrics, pages 497–507.
Saigo, H., Nowozin, S., Kadowaki, T., Kudo, T., and Tsuda,
K. (2009). gboost: a mathematical programming ap-
proach to graph classification and regression. Machine
Learning, 75:69–89.
Schumacher, M., Bastert, G., Bojar, H., H
¨
ubner, K.,
Olschewski, M., Sauerbrei, W., Schmoor, C., Beyerle,
C., Neumann, R., and Rauschecker, H. (1994). for
the german breast cancer study group (gbsg). random-
ized 2x2 trial evaluating hormonal treatment and the
duration of chemotherapy in node-positive breast can-
cer patients. Journal of Clinical Oncology, 12:2086–
2093.
Schwartz, J., Samet, J. M., and Patz, J. A. (2004). Hospital
admissions for heart disease: the effects of tempera-
ture and humidity. Epidemiology, 15(6):755–761.
Van Belle, V., Pelckmans, K., Van Huffel, S., and Suykens,
J. A. (2011). Support vector methods for survival
analysis: a comparison between ranking and regres-
sion approaches. Artificial intelligence in medicine,
53(2):107–118.
Weibin, W. (2022). Deep learning-based prediction mod-
els of early recurrence and recurrence-free survival
in hepatocellular carcinoma with multi-phase ct. Rit-
sumeikan University.
Zhai, Y.-j., Zhang, Y., Liu, H.-z., and Zhang, Z.-r. (2023).
Multi-angle support vector survival analysis with neu-
ral tangent kernel study. Arabian Journal for Science
and Engineering, 48(8):10267–10284.
IoTBDS 2025 - 10th International Conference on Internet of Things, Big Data and Security
190