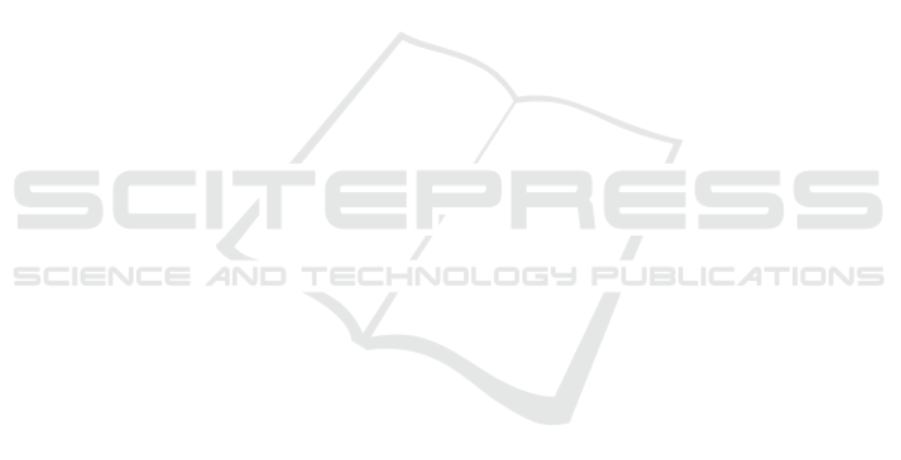
Carlini, N. and Wagner, D. (2018). Audio adversarial ex-
amples: Targeted attacks on speech-to-text.
Chen, Z., Zhang, J. M., Hort, M., Harman, M., and Sarro, F.
(2024). Fairness testing: A comprehensive survey and
analysis of trends. ACM Trans. Softw. Eng. Methodol.
Just Accepted.
Chu, Z., Wang, Z., and Zhang, W. (2024). Fairness in large
language models: A taxonomic survey.
D’Amour, A., Heller, K., Moldovan, D., Adlam, B., Ali-
panahi, B., Beutel, A., Chen, C., Deaton, J., Eisen-
stein, J., Hoffman, M. D., Hormozdiari, F., Houlsby,
N., Hou, S., Jerfel, G., Karthikesalingam, A., Lucic,
M., Ma, Y., McLean, C., Mincu, D., Mitani, A., Mon-
tanari, A., Nado, Z., Natarajan, V., Nielson, C., Os-
borne, T. F., Raman, R., Ramasamy, K., Sayres, R.,
Schrouff, J., Seneviratne, M., Sequeira, S., Suresh,
H., Veitch, V., Vladymyrov, M., Wang, X., Webster,
K., Yadlowsky, S., Yun, T., Zhai, X., and Sculley,
D. (2020). Underspecification presents challenges for
credibility in modern machine learning.
Falcone, Y., Havelund, K., and Reger, G. (2013). A tuto-
rial on runtime verification. Engineering dependable
software systems, pages 141–175.
Falcone, Y., Krsti
´
c, S., Reger, G., and Traytel, D. (2021).
A taxonomy for classifying runtime verification tools.
International Journal on Software Tools for Technol-
ogy Transfer, 23(2).
Francalanza, A., Pérez, J. A., and Sánchez, C. (2018). Run-
time Verification for Decentralised and Distributed
Systems, pages 176–210. Springer International Pub-
lishing, Cham.
Geirhos, R., Jacobsen, J.-H., Michaelis, C., Zemel, R.,
Brendel, W., Bethge, M., and Wichmann, F. A. (2020).
Shortcut learning in deep neural networks. Nature
Machine Intelligence, 2(11):665–673.
Ghazarian, A., Zheng, J., Struppa, D., and Rakovski, C.
(2022). Assessing the reidentification risks posed by
deep learning algorithms applied to ecg data. IEEE
Access, 10.
Goodfellow, I., Bengio, Y., and Courville, A. (2016). Deep
Learning. MIT Press. http://www.deeplearningbook.
org.
Harder, P., Pfreundt, F.-J., Keuper, M., and Keuper, J.
(2021). Spectraldefense: Detecting adversarial attacks
on cnns in the fourier domain. In 2021 International
Joint Conference on Neural Networks (IJCNN).
Hendrycks, D. and Dietterich, T. (2019). Benchmarking
neural network robustness to common corruptions and
perturbations.
Heo, J., Joo, S., and Moon, T. (2019). Fooling neural net-
work interpretations via adversarial model manipula-
tion. In Wallach, H., Larochelle, H., Beygelzimer,
A., d'Alché-Buc, F., Fox, E., and Garnett, R., editors,
NeurIPS, volume 32. Curran Associates, Inc.
Holstein, K., Wortman Vaughan, J., Daumé, H., Dudik, M.,
and Wallach, H. (2019). Improving fairness in ma-
chine learning systems: What do industry practition-
ers need? In Proceedings of the 2019 CHI Confer-
ence on Human Factors in Computing Systems, CHI
’19, page 1–16, New York, NY, USA. Association for
Computing Machinery.
Hurlin, C., Pérignon, C., and Saurin, S. (2024). The fairness
of credit scoring models.
Hynes, N., Sculley, D., and Terry, M. (2017). The data
linter: Lightweight automated sanity checking for ml
data sets.
Inan, H., Upasani, K., Chi, J., Rungta, R., Iyer, K., Mao, Y.,
Tontchev, M., Hu, Q., Fuller, B., Testuggine, D., and
Khabsa, M. (2023). Llama guard: Llm-based input-
output safeguard for human-ai conversations.
Kauffmann, J., Ruff, L., Montavon, G., and Müller, K.-R.
(2020). The clever hans effect in anomaly detection.
Lahat, D., Adali, T., and Jutten, C. (2015). Multimodal
data fusion: An overview of methods, challenges, and
prospects. Proceedings of the IEEE, 103(9).
Li, C., Wong, C., Zhang, S., Usuyama, N., Liu, H., Yang,
J., Naumann, T., Poon, H., and Gao, J. (2023). Llava-
med: Training a large language-and-vision assistant
for biomedicine in one day.
Li, S., Guo, J., Lou, J.-G., Fan, M., Liu, T., and Zhang, D.
(2022). Testing machine learning systems in industry:
an empirical study. In Proceedings of the 44th Inter-
national Conference on Software Engineering: Soft-
ware Engineering in Practice, ICSE-SEIP ’22, page
263–272, New York, NY, USA. Association for Com-
puting Machinery.
Li, T., Pang, G., Bai, X., Miao, W., and Zheng, J. (2024a).
Learning transferable negative prompts for out-of-
distribution detection.
Li, Y., Guo, H., Zhou, K., Zhao, W. X., and Wen, J.-R.
(2024b). Images are achilles’ heel of alignment: Ex-
ploiting visual vulnerabilities for jailbreaking multi-
modal large language models.
Lindemann, L., Qin, X., Deshmukh, J. V., and Pappas, G. J.
(2023). Conformal prediction for stl runtime verifica-
tion. In ICCPS ’23, page 142–153, New York, NY,
USA. Association for Computing Machinery.
Liu, X., Xie, L., Wang, Y., Zou, J., Xiong, J., Ying, Z., and
Vasilakos, A. V. (2021). Privacy and security issues in
deep learning: A survey. IEEE Access, 9.
Liu, Y., Tantithamthavorn, C., Liu, Y., and Li, L. (2024). On
the reliability and explainability of language models
for program generation. ACM Transactions on Soft-
ware Engineering and Methodology.
Lwakatare, L. E., Raj, A., Crnkovic, I., Bosch, J., and Ols-
son, H. H. (2020). Large-scale machine learning sys-
tems in real-world industrial settings: A review of
challenges and solutions. Information and Software
Technology, 127:106368.
Ma, P., Petridis, S., and Pantic, M. (2021). Detecting adver-
sarial attacks on audiovisual speech recognition.
Marathe, S., Nambi, A., Swaminathan, M., and Sutaria, R.
(2021). Currentsense: A novel approach for fault and
drift detection in environmental iot sensors. In IoTDI,
IoTDI ’21, page 93–105, New York, NY, USA. Asso-
ciation for Computing Machinery.
Metzen, J. H., Genewein, T., Fischer, V., and Bischoff, B.
(2017). On detecting adversarial perturbations.
Mireshghallah, F., Taram, M., Vepakomma, P., Singh, A.,
Raskar, R., and Esmaeilzadeh, H. (2020). Privacy in
deep learning: A survey.
ENASE 2025 - 20th International Conference on Evaluation of Novel Approaches to Software Engineering
376