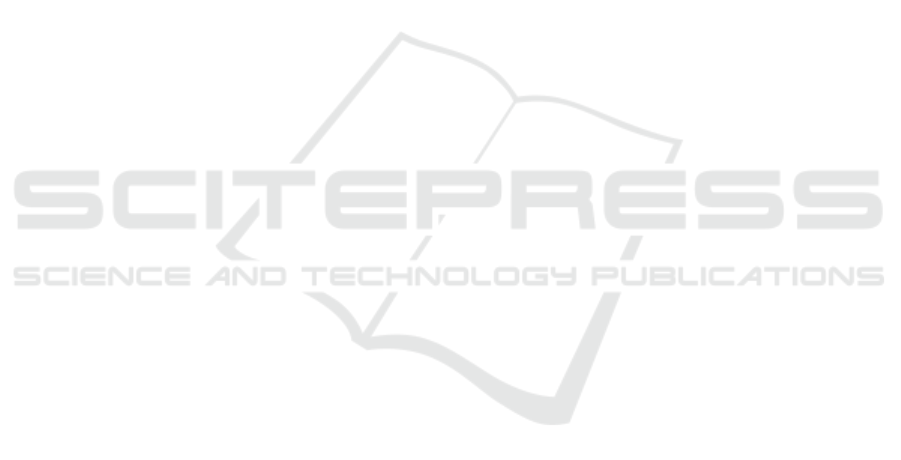
demic performance. The study concluded that high-
resilience students tend to have greater life satisfac-
tion and are more likely to excel academically. Addi-
tionally, a supportive environment enhances students’
ability to study effectively and perform well at uni-
versity. Academic programs can implement various
initiatives to improve students’ perceived experiences
and strengthen their resilience.
The findings can inform curriculum design and the
learning environment in academic programs. Follow-
up studies should be conducted to evaluate their im-
pact. This could involve implementing initiatives as
academic interventions and measuring students’ re-
silience and academic performance before and after
the interventions. Further research on student expe-
riences and changes in resilience during and after the
COVID period would also be valuable.
REFERENCES
Abolghasemi, A. and Varaniyab, S. T. (2010). Resilience
and perceived stress: predictors of life satisfaction in
the students of success and failure. Procedia-Social
and Behavioral Sciences, 5:748–752.
Abraham, J. and Barker, K. (2015). Exploring gender
difference in motivation, engagement and enrolment
behaviour of senior secondary physics students in
new south wales. Research in Science Education,
45(1):59–73.
Allan, J. F., McKenna, J., and Dominey, S. (2014). Degrees
of resilience: profiling psychological resilience and
prospective academic achievement in university in-
ductees. British Journal of Guidance & Counselling,
42(1):9–25.
Asif, R., Merceron, A., and et al., S. A. A. (2017). An-
alyzing undergraduate students’ performance using
educational data mining. Computers & Education,
113:177–194.
Ayala, J. C. and Manzano, G. (2018). Academic perfor-
mance of first-year university students: The influence
of resilience and engagement. Higher Education Re-
search & Development, 37(7):1321–1335.
Barker, L., Hovey, C. L., and Thompson, L. D. (2014). Re-
sults of a large-scale, multi-institutional study of un-
dergraduate retention in computing. In Proceedings
of the 2014 IEEE Frontiers in Education Conference
(FIE), pages 1–8.
Cassidy, S. (2016). The academic resilience scale (ars-30):
A new multidimensional construct measure. Frontiers
in Psychology, 7(2016):1787.
Clarke, J. and Nicholson, J. (2010). Resilience: bounce
back from whatever life throws at you. Crimson, Ha-
chette, UK.
Crawford-Garrett, K. (2018). Lacking resilience or mount-
ing resistance? interpreting the actions of indige-
nous and immigrant youth within teachfirst new
zealand. American Educational Research Journal,
55(5):1051–1075.
Eisenberg, D., Lipson, S. K., and Posselt, J. (2016). Pro-
moting resilience, retention, and mental health. New
Directions for Student Services, 156:87–95.
Kwek, A., Bui, H. T., and et al., J. R. (2013). The impacts of
self-esteem and resilience on academic performance:
An investigation of domestic and international hospi-
tality and tourism undergraduate students. Journal of
Hospitality & Tourism Education, 25(3):110–122.
MacLeod, S., Musich, S., and et al., K. H. (2016). The im-
pact of resilience among older adults. Geriatric Nurs-
ing, 37(4):266–272.
NCWIT (2021). Survey-in-a-box: Student experience of
the major.
Okubo, F., Yamashita, T., and et al., A. S. (2017). Stu-
dents’ performance prediction using data of multiple
courses by recurrent neural network. In Proceedings
of the 25th International Conference on Computers in
Education (ICCE 2017), pages 439–444.
Pathak, R. and Lata, S. (2018). Optimism in relation to re-
silience and perceived stress. Journal of Psychosocial
Research, 13(2):359–367.
Prickett, T., Walters, J., and et al., L. Y. (2020). Resilience
and effective learning in first-year undergraduate com-
puter science. In Proceedings of the 2020 ACM Con-
ference on Innovation and Technology in Computer
Science Education, pages 19–25.
Rodr
´
ıguez-Fern
´
andez, A., Ramos-D
´
ıaz, E., and Axpe, I.
(2018). The role of resilience and psychological well-
being in school engagement and perceived academic
performance: An exploratory model to improve aca-
demic achievement. Health and Academic Achieve-
ment, 10(1):159–176.
Stephen, H., Lucy, T., and Paul, A. (2013). Measuring sub-
jective well-being and its potential role in policy: per-
spectives from the UK office for national statistics. So-
cial Indicators Research, 114(1):73–86.
VanderWeele, T. J., Trudel-Fitzgerald, C., and et al., P. A.
(2020). Current recommendations on the selection
of measures for well-being. Preventive Medicine,
133:106004.
Widjaja, A. T., Wang, L., Truong, N. T., Gunawan, A., and
Lim, E.-P. (2020). Next-term grade prediction: A ma-
chine learning approach. In Proceedings of the 13th
International Conference on Educational Data Min-
ing (EDM 2020).
Wu, G., Feder, A., Cohen, H., Kim, J. J., Calderon, S., and
et al., D. S. C. (2013). Understanding resilience. Fron-
tiers in behavioral neuroscience, 7:10–10.
York, T. T., Gibson, C., and Rankin, S. (2015). Defining and
measuring academic success. Practical Assessment,
Research, and Evaluation, 20(1):5–5.
On Unraveling Student Resilience and Academic Performance in Higher Education
215