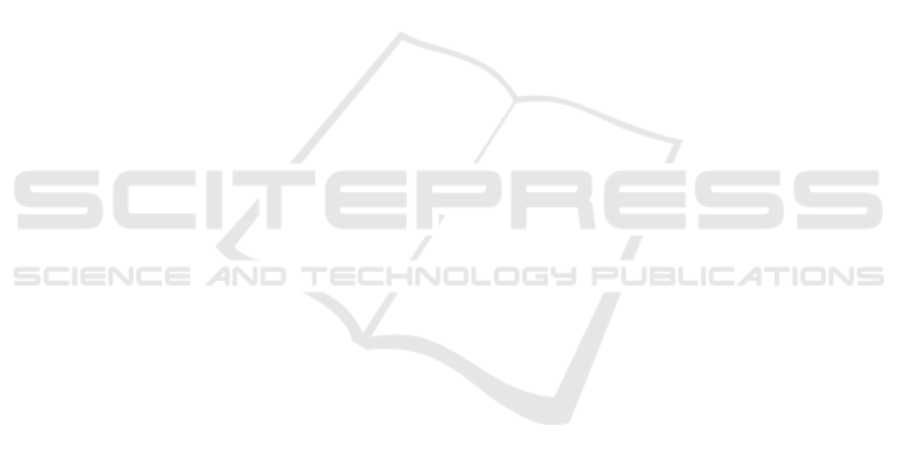
(Lee, 2020) present a review of IoT cybersecurity risk
management frameworks that cover both qualitative
and quantitative approaches, and in their review, they
also propose a four-layered IoT cyber risk manage-
ment framework consisting of (1) IoT cyber ecosys-
tem layer, (2) IoT cyber infrastructure layer, (3) IoT
cyber risk assessment layer, and (4) IoT cyber perfor-
mance layer.
Khosravi-Farmad et al. (Khosravi-Farmad and
Ghaemi-Bafghi, 2020) proposed a quantitative risk
management approach that is based on Bayesian deci-
sion networks. The framework also expands Bayesian
attack graphs by integrating risk mitigation phases to
address countermeasures for risk reduction. The pro-
posed model is made up of three phases: (1) risk as-
sessment, (2) risk mitigation, and (3) risk validation
and monitoring. One possible improvement of their
proposed approach is the need to improve the accu-
racy of the risk assessment process.
A quantitative risk assessment model that is es-
pecially related to privacy in smart homes IoT-based
systems is presented in (Werner et al., 2022). A ques-
tionnaire covering 15 major concerns with respect to
privacy were designed to reveal several features of the
connected home IoT devices for the purpose of as-
sessing the risks that are targeting them. Similarly,
(Wang et al., 2023) proposed a privacy risk assess-
ment method called STPA–FMEA to uncover 35 pri-
vacy risk scenarios related to smart home systems.
While there are many risk assessment frameworks
in the literature, none of them, to the best of our
knowledge, fully integrates qualitative and quantita-
tive approaches to provide an enhanced method for
the analysis and management of risk in IoT ecosys-
tems. In addition, these studies typically also pay less
attention to risk assessment methodology that takes
into consideration aspects of uncertainty in their risk
measurement approaches. In this paper, we aim to fill
these gaps by proposing a model that explicitly inte-
grates the opinions of IoT experts, researchers, prac-
titioners, and risk analysts during the risk assessment
process, while also incorporating fuzzy AHP to that
aspects of uncertainty and imprecision that are asso-
ciated with risk assessment. Our proposed method,
RAM-IoT, thus integrates both qualitative and quan-
titative approaches, while also taking uncertainty into
account, specifically for risk assessment in the IoT.
3 PROPOSED APPROACH
This section details the components of RAM-IoT and
provides formal guidance on its requirements. RAM-
IoT is an asset-based risk assessment model designed
for IoT ecosystems, considering the various layers
involved in the typical case of IoT service delivery.
Each layer introduces specific attack surfaces that
may be exposed through interactions between com-
ponents across different layers. The model acknowl-
edges the inherently subjective nature of risk assess-
ments conducted by IoT analysts and other stakehold-
ers. To address this, RAM-IoT employs both qualita-
tive and quantitative risk assessment methods, offer-
ing a comprehensive and robust approach to risk as-
sessment and mitigation within IoT ecosystems.
As shown in Figure 1, the proposed risk assess-
ment model consists of different modules. RAM-IoT
considers fuzzy AHP as a multi-criteria decision mak-
ing (MCDM) approach for risk assessment to define
empirical risk measures relating to the vulnerabilities
and threats targeting the specific asset. Therefore, the
model can be viewed as providing a set of formal
structural guidelines to IoT risk analysts and their cor-
responding stakeholders on how to identify and quan-
tify risks that are associated with specific assets in the
IoT.
RAM-IoT can be formally conceptualized as a
tuple IE = (U, S, A,V, T, R, F) where IE: the IoT
ecosystem, U: users, A: assets related to S, S: IoT
services, V : vulnerabilities related to the assets which
can be exploited by threat sources, T : threats target-
ing the assets A, R: risks estimation function that ad-
dresses both qualitative and quantitative risk assess-
ment methodologies. F: a mapping function that
maps the risk score to risk severity. The detail of each
component is provided as follows.
3.1 The IoT Ecosystem (ie)
Figure 2 presents a generic four-layer architecture of
IoT highlighting security and privacy as major con-
cerns. The security and privacy issues cut across
the different layers of the IoT ecosystem including
the perception where the ”things” (devices, actua-
tors, etc.) in the IoT are accommodated. The net-
work layer that concerns with communication tech-
nologies, such as Zigbee, WiFi, Bluetooth, Celullar
(e.g. 5G), LoRaWAN, and MQTT (Adewole and
Jacobsson, 2024a; Adewole and Jacobsson, 2024b).
The management layer that is responsible for storage,
computing and device management, as well as for the
application and service layer that accommodates dif-
ferent software applications that render services to the
users. Services may include support for building au-
tomation, health and wellness, energy consumption
monitoring, safety and security surveillance, and so
on. Users interact with various components in the IoT
ecosystem, hence, their security and privacy are of
RAM-IoT: Risk Assessment Model for IoT-Based Critical Assets
193