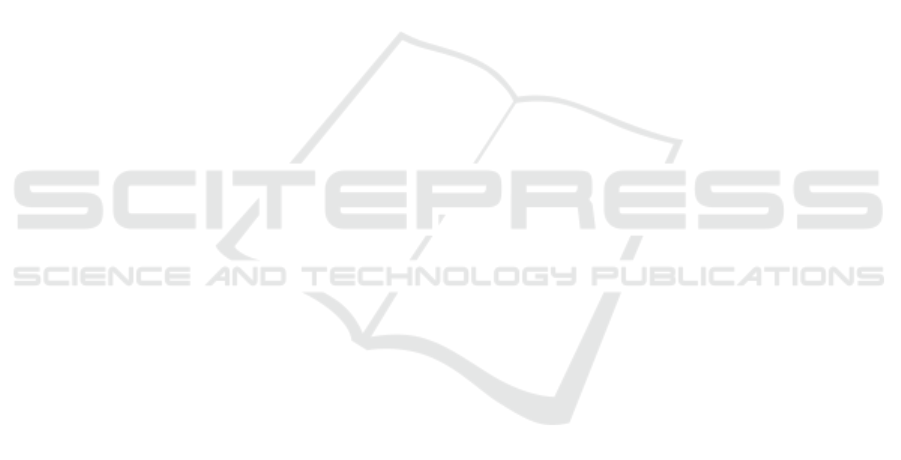
contrastive self-supervised texture learning. Medical
Physics, 49(6):3638–3653.
Chen, T., He, T., Benesty, M., Khotilovich, V., Tang, Y.,
Cho, H., Chen, K., Mitchell, R., Cano, I., Zhou, T.,
et al. (2015). Xgboost: extreme gradient boosting. R
package version 0.4-2, 1(4):1–4.
Corlan, A.-S., Diogen, B., Flavia, C., and Darian, O. (2023).
Prediction and classification models for hashimoto. In
Endocrine Abstracts, volume 90. Bioscientifica.
Corlan, A.-S., Diogen, B., Flavia, C., Vlad, M., Balas, M.,
Golu, I., Amzar, D.-G., and Darian, O. (2024). An ar-
tificial intelligence system for estimating the improve-
ment of clinical and paraclinical parameters after ther-
apy in pituitary tumors. In Endocrine Abstracts, vol-
ume 99. Bioscientifica.
Hearst, M. A., Dumais, S. T., Osuna, E., Platt, J., and
Scholkopf, B. (1998). Support vector machines. IEEE
Intelligent Systems and their applications, 13(4):18–
28.
Hussain, E., Mahanta, L. B., Das, C. R., and Talukdar, R. K.
(2020). A comprehensive study on the multi-class
cervical cancer diagnostic prediction on pap smear
images using a fusion-based decision from ensemble
deep convolutional neural network. Tissue and Cell,
65:101347.
Kanavati, F., Hirose, N., Ishii, T., Fukuda, A., Ichihara,
S., and Tsuneki, M. (2022). A deep learning model
for cervical cancer screening on liquid-based cytol-
ogy specimens in whole slide images. Cancers,
14(5):1159.
Khamparia, A., Gupta, D., Rodrigues, J. J., and de Al-
buquerque, V. H. C. (2021). Dcavn: Cervical can-
cer prediction and classification using deep convolu-
tional and variational autoencoder network. Multime-
dia Tools and Applications, 80:30399–30415.
Kudva, V., Prasad, K., and Guruvare, S. (2020). Hybrid
transfer learning for classification of uterine cervix
images for cervical cancer screening. Journal of digi-
tal imaging, 33:619–631.
Lehman, C. D., Wellman, R. D., Buist, D. S., Kerlikowske,
K., Tosteson, A. N., Miglioretti, D. L., Consor-
tium, B. C. S., et al. (2015). Diagnostic accuracy
of digital screening mammography with and without
computer-aided detection. JAMA internal medicine,
175(11):1828–1837.
Li, P., Liang, J., and Zhang, M. (2021). A degradation
model for simultaneous brightness and sharpness en-
hancement of low-light image. Signal Processing,
189:108298.
Nandy, A., Sathish, R., and Sheet, D. (2020). Identifica-
tion of cervical pathology using adversarial neural net-
works. arXiv preprint arXiv:2004.13406.
Pal, A., Xue, Z., Befano, B., Rodriguez, A. C., Long, L. R.,
Schiffman, M., and Antani, S. (2021). Deep metric
learning for cervical image classification. IEEE Ac-
cess, 9:53266–53275.
Park, Y. R., Kim, Y. J., Ju, W., Nam, K., Kim, S., and
Kim, K. G. (2021). Comparison of machine and
deep learning for the classification of cervical cancer
based on cervicography images. Scientific Reports,
11(1):16143.
Rahaman, M. M., Li, C., Yao, Y., Kulwa, F., Wu, X., Li, X.,
and Wang, Q. (2021). Deepcervix: A deep learning-
based framework for the classification of cervical cells
using hybrid deep feature fusion techniques. Comput-
ers in Biology and Medicine, 136:104649.
Ribeiro, E., Uhl, A., Wimmer, G., H
¨
afner, M., et al.
(2016). Exploring deep learning and transfer learning
for colonic polyp classification. Computational and
mathematical methods in medicine, 2016.
Sabottke, C. F. and Spieler, B. M. (2020). The effect of
image resolution on deep learning in radiography. Ra-
diology: Artificial Intelligence, 2(1):e190015.
Sellamuthu Palanisamy, V., Athiappan, R. K., and Na-
galingam, T. (2022). Pap smear based cervical cancer
detection using residual neural networks deep learning
architecture. Concurrency and Computation: Practice
and Experience, 34(4):e6608.
Sompawong, N., Mopan, J., Pooprasert, P., Himakhun, W.,
Suwannarurk, K., Ngamvirojcharoen, J., Vachiramon,
T., and Tantibundhit, C. (2019). Automated pap smear
cervical cancer screening using deep learning. In 2019
41st Annual International Conference of the IEEE En-
gineering in Medicine and Biology Society (EMBC),
pages 7044–7048. IEEE.
Tekchandani, H., Verma, S., Londhe, N. D., Jain, R. R.,
and Tiwari, A. (2022). Computer aided diagnosis sys-
tem for cervical lymph nodes in ct images using deep
learning. Biomedical Signal Processing and Control,
71:103158.
Tsikouras, P., Zervoudis, S., Manav, B., Tomara, E., Ia-
trakis, G., Romanidis, C., Bothou, A., and Galazios,
G. (2016). Cervical cancer: screening, diagnosis and
staging. J buon, 21(2):320–325.
Wang, Z., Chen, J., and Hoi, S. C. (2020). Deep learning
for image super-resolution: A survey. IEEE trans-
actions on pattern analysis and machine intelligence,
43(10):3365–3387.
William, W., Ware, A., Basaza-Ejiri, A. H., and Obun-
goloch, J. (2018). A review of image analysis and
machine learning techniques for automated cervical
cancer screening from pap-smear images. Computer
methods and programs in biomedicine, 164:15–22.
Xue, Z., Guo, P., Desai, K. T., Pal, A., Ajenifuja, K. O.,
Adepiti, C. A., Long, L. R., Schiffman, M., and
Antani, S. (2021). A deep clustering method for
analyzing uterine cervix images across imaging de-
vices. In 2021 IEEE 34th International Symposium
on Computer-Based Medical Systems (CBMS), pages
527–532. IEEE.
Zhang, L., Wang, X., Yang, D., Sanford, T., Harmon, S.,
Turkbey, B., Wood, B. J., Roth, H., Myronenko, A.,
Xu, D., et al. (2020). Generalizing deep learning for
medical image segmentation to unseen domains via
deep stacked transformation. IEEE transactions on
medical imaging, 39(7):2531–2540.
ICT4AWE 2025 - 11th International Conference on Information and Communication Technologies for Ageing Well and e-Health
180