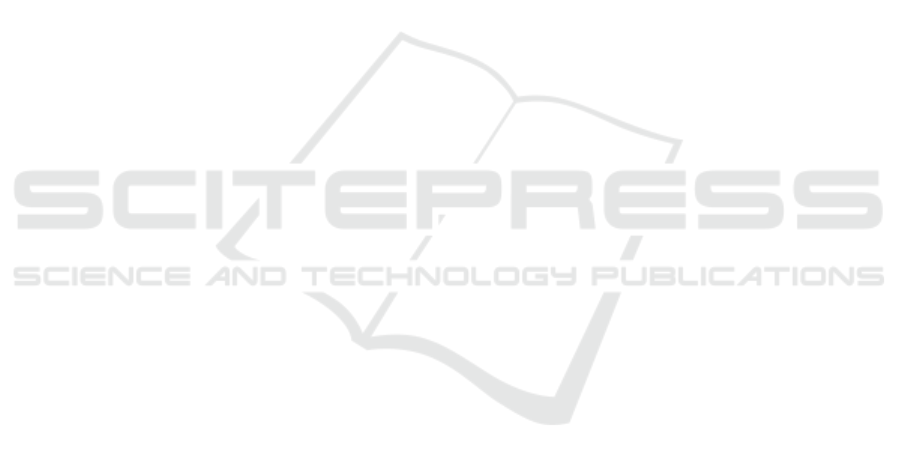
ulator. In Conference on robot learning, pages 1–16.
PMLR.
Fabbri, M., Bras
´
o, G., Maugeri, G., Cetintas, O., Gasparini,
R., O
ˇ
sep, A., Calderara, S., Leal-Taix
´
e, L., and Cuc-
chiara, R. (2021). Motsynth: How can synthetic data
help pedestrian detection and tracking? In Proceed-
ings of the IEEE/CVF International Conference on
Computer Vision, pages 10849–10859.
Geiger, A., Lenz, P., Stiller, C., and Urtasun, R. (2013).
Vision meets robotics: The kitti dataset. The Inter-
national Journal of Robotics Research, 32(11):1231–
1237.
Geyer, J., Kassahun, Y., Mahmudi, M., Ricou, X., Durgesh,
R., Chung, A. S., Hauswald, L., Pham, V. H.,
M
¨
uhlegg, M., Dorn, S., et al. (2020). A2d2:
Audi autonomous driving dataset. arXiv preprint
arXiv:2004.06320.
Jocher, G., Stoken, A., Borovec, J., Changyu, L., Hogan, A.,
Diaconu, L., Ingham, F., Poznanski, J., Fang, J., Yu,
L., et al. (2020). ultralytics/yolov5: v3. 1-bug fixes
and performance improvements. Zenodo.
Kuznetsova, A., Rom, H., Alldrin, N., Uijlings, J., Krasin,
I., Pont-Tuset, J., Kamali, S., Popov, S., Malloci, M.,
Kolesnikov, A., et al. (2020). The open images dataset
v4: Unified image classification, object detection, and
visual relationship detection at scale. International
journal of computer vision, 128(7):1956–1981.
Lin, T.-Y., Maire, M., Belongie, S., Hays, J., Perona, P.,
Ramanan, D., Doll
´
ar, P., and Zitnick, C. L. (2014).
Microsoft coco: Common objects in context. In Com-
puter Vision–ECCV 2014: 13th European Confer-
ence, Zurich, Switzerland, September 6-12, 2014, Pro-
ceedings, Part V 13, pages 740–755. Springer.
Linder, T., Wehner, S., and Arras, K. O. (2015). Real-time
full-body human gender recognition in (rgb)-d data.
2015 IEEE International Conference on Robotics and
Automation (ICRA), pages 3039–3045.
Luna-Romero, S. F., Stempniak, C. R., de Souza, M. A.,
and Reynoso-Meza, G. (2024). Urban digital twins
for synthetic data of individuals with mobility aids in
curitiba, brazil, to drive highly accurate ai models for
inclusivity. In Salgado-Guerrero, J. P., Vega-Carrillo,
H. R., Garc
´
ıa-Fern
´
andez, G., and Robles-Bykbaev, V.,
editors, Systems, Smart Technologies and Innovation
for Society, pages 116–125, Cham. Springer Nature
Switzerland.
Maddern, W., Pascoe, G., Linegar, C., and Newman, P.
(2017). 1 year, 1000 km: The oxford robotcar
dataset. The International Journal of Robotics Re-
search, 36(1):3–15.
Mohr, L., Kirillova, N., Possegger, H., and Bischof, H.
(2023). A comprehensive crossroad camera dataset
of mobility aid users. In 34th British Machine Vision
Conference: BMVC 2023. The British Machine Vi-
sion Association.
Nieto, M., Senderos, O., and Otaegui, O. (2021). Boosting
ai applications: Labeling format for complex datasets.
SoftwareX, 13:100653.
Padilla, R., Netto, S. L., and Da Silva, E. A. (2020). A sur-
vey on performance metrics for object-detection algo-
rithms. In 2020 international conference on systems,
signals and image processing (IWSSIP), pages 237–
242. IEEE.
Rashed, H., Mohamed, E., Sistu, G., Kumar, V. R., Eis-
ing, C., El-Sallab, A., and Yogamani, S. (2021). Gen-
eralized object detection on fisheye cameras for au-
tonomous driving: Dataset, representations and base-
line. In Proceedings of the IEEE/CVF Winter Con-
ference on Applications of Computer Vision, pages
2272–2280.
Scheuerman, M. K., Spiel, K., Haimson, O. L., Hamidi, F.,
and Branham, S. M. (2020). Hci guidelines for gender
equity and inclusivity.
Schumann, C., Ricco, S., Prabhu, U., Ferrari, V., and Panto-
faru, C. (2021). A step toward more inclusive peo-
ple annotations for fairness. In Proceedings of the
2021 AAAI/ACM Conference on AI, Ethics, and So-
ciety, pages 916–925.
Schwartz, R., Schwartz, R., Vassilev, A., Greene, K., Per-
ine, L., Burt, A., and Hall, P. (2022). Towards a stan-
dard for identifying and managing bias in artificial in-
telligence, volume 3. US Department of Commerce,
National Institute of Standards and Technology.
Shah, S., Dey, D., Lovett, C., and Kapoor, A. (2018). Air-
sim: High-fidelity visual and physical simulation for
autonomous vehicles. In Field and Service Robotics:
Results of the 11th International Conference, pages
621–635. Springer.
Shahbazi, N., Lin, Y., Asudeh, A., and Jagadish, H. (2023).
Representation bias in data: A survey on identification
and resolution techniques. ACM Computing Surveys,
55(13s):1–39.
Sharma, G. and Jurie, F. (2011). Learning discrimina-
tive spatial representation for image classification.
In BMVC 2011-British Machine Vision Conference,
pages 1–11. BMVA Press.
Sun, P., Kretzschmar, H., Dotiwalla, X., Chouard, A., Pat-
naik, V., Tsui, P., Guo, J., Zhou, Y., Chai, Y., Caine,
B., et al. (2020). Scalability in perception for au-
tonomous driving: Waymo open dataset. In Proceed-
ings of the IEEE/CVF conference on computer vision
and pattern recognition, pages 2446–2454.
Unreal Engine Marketplace (2024a). Old Man
Animset. https://www.fab.com/listings/
8fcce9be-d727-44f1-9261-56cfa8ef41e4. Accessed:
2024-06-01.
Unreal Engine Marketplace (2024b). Run and
Walk. https://www.fab.com/es-es/listings/
6f5351b5-b6c9-4e00-a248-8158e6a7c067. Ac-
cessed: 2025-01-07.
Vasquez, A., Kollmitz, M., Eitel, A., and Burgard, W.
(2017). Deep detection of people and their mobil-
ity aids for a hospital robot. In Proc. of the IEEE
Eur. Conf. on Mobile Robotics (ECMR).
Wilson, B., Qi, W., Agarwal, T., Lambert, J., Singh, J.,
Khandelwal, S., Pan, B., Kumar, R., Hartnett, A.,
Pontes, J. K., Ramanan, D., Carr, P., and Hays,
J. (2021). Argoverse 2: Next generation datasets
for self-driving perception and forecasting. In Pro-
ceedings of the Neural Information Processing Sys-
tems Track on Datasets and Benchmarks (NeurIPS
Datasets and Benchmarks 2021).
DiverSim: A Customizable Simulation Tool to Generate Diverse Vulnerable Road User Datasets
23