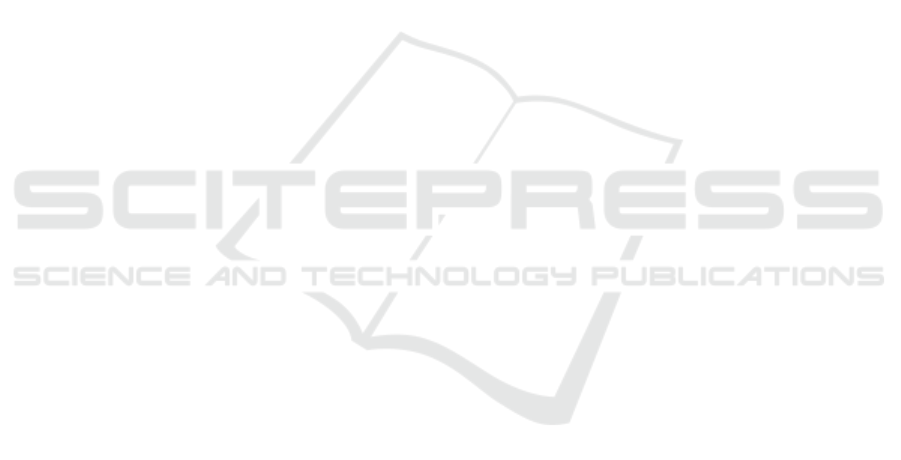
quality to include urban discovery, social integration,
and language acquisition. Adaptive learning algo-
rithms to further enhance personalization should also
be explored. Finally, examining long-term effects on
social integration and well-being is paramount.
This study underscores the significant role of rec-
ommender systems in helping international students
document, reflect on, and engage with their experi-
ences in host cities. By addressing their specific chal-
lenges, the system fosters self-reflection, enriches lo-
cal engagement, and supports integration through tai-
lored suggestions. These systems not only enhance
students’ ability to capture their experiences but also
contribute to their personal growth and sense of be-
longing. Ultimately, this research demonstrates the
potential of adaptive, personalized technologies to
create more inclusive and engaging urban experiences
for diverse global communities.
ACKNOWLEDGEMENTS
This work is supported by the MOBILES project
funded by the Agence Nationale de la Recherche
(ANR), France (grant ANR-20-CE38-0009).
REFERENCES
De Stefani, E. and De Marco, D. (2019). Language, gesture,
and emotional communication: An embodied view of
social interaction. Frontiers in Psychology, 10:2063.
Deardorff, D. K. (2006). Identification and assessment of
intercultural competence as a student outcome of in-
ternationalization. Journal of studies in international
education, 10(3):241–266.
Gumbheer, C. P., Khedo, K. K., and Bungaleea, A.
(2022). Personalized and adaptive context-aware
mobile learning: review, challenges and future di-
rections. Education and Information Technologies,
27(6):7491–7517.
Guo, D., McTigue, E. M., Matthews, S. D., and Zimmer,
W. (2020). The impact of visual displays on learning
across the disciplines: A systematic review. Educa-
tional Psychology Review, 32(3):627–656.
Gutema, D. M., Pant, S., and Nikou, S. (2024). Exploring
key themes and trends in international student mobil-
ity research—a systematic literature review. Journal
of Applied Research in Higher Education, 16(3):843–
861.
Huynh, T. and Tran, L. T. (2023). Digitalisation of the
teaching and learning for international students in
higher education: A systematic review. Journal
of Comparative and International Higher Education,
15(3):63–80.
Kukulska-Hulme, A. (2020). Mobile and personal learning
for newcomers to a city. Electronic Journal of Foreign
Language Teaching, 17(1):93–103.
Lefevre, M., Benedetto, R., Sadallah, M., Chevalier, D.,
Cunty, C., Grassin, J.-F., Joliveau, T., Mathian, H.,
and Scheffler, C. (2024). MOBILES: A Mobile Ap-
plication for Documenting the Urban and Social Ex-
periences of International Students. Software, Version
1.2.41.
Loewen, S., Isbell, D. R., and Sporn, Z. (2020). The effec-
tiveness of app-based language instruction for devel-
oping receptive linguistic knowledge and oral commu-
nicative ability. Foreign Language Annals, 53(2):209–
233.
Martirosyan, N. M., Bustamante, R., and Saxon, D. P.
(2019). Academic and social support services for in-
ternational students: Current practices. Journal of In-
ternational Students, 9(1):172–191.
Mueller, C. M. and Richardson, P. (2022). Talking about
oneself: Effects of personalized prompts on l2 learn-
ers’ speech. Journal of Second Language Studies,
5(2):344–363.
Patel, N., Calhoun, D. W., and Tolman, S. (2024). Under-
standing the role of cultural competence in peer men-
torship programs for international students: A student
development theory perspective. Georgia Journal of
College Student Affairs, 40(1):62–80.
Sadallah, M. and Lefevre, M. (2024). MOBILES RecSys:
A Personalized Recommendation System for Enhanc-
ing the Urban and Social Experiences of International
Students. Software, Version 1.0.
Santos, R. (2020). The visual storytelling as a way to create
knowledge and empathy between generations in aca-
demic institutions. In The Barcelona Conference on
Arts Media & Culture 2020, Official Conference Pro-
ceedings IAFOR Barcelona, pages 1–14.
Sassi, I. B., Mellouli, S., and Yahia, S. B. (2017). Context-
aware recommender systems in mobile environment:
On the road of future research. Information Systems,
72:27–61.
Settouti, L. S., Pri
´
e, Y., Cram, D., Champin, P.-A., and
Mille, A. (2009). A trace-based framework for sup-
porting digital object memories. In Workshops Pro-
ceedings of the 5th International Conference on Intel-
ligent Environments, pages 39–44. IOS Press.
Silva, F. L., Slodkowski, B. K., Ara
´
ujo da Silva, K. K.,
and Cazella, S. C. (2022). A systematic literature re-
view on educational recommender systems for teach-
ing and learning: research trends, limitations and op-
portunities. Education and Information Technologies,
28:3289–3328.
Sun, L. (2023). Social media usage and students’ so-
cial anxiety, loneliness and well-being: does digi-
tal mindfulness-based intervention effectively work?
BMC psychology, 11(1):362.
Urdaneta-Ponte, M. C., Mendez-Zorrilla, A., and
Oleagordia-Ruiz, I. (2021). Recommendation
systems for education: Systematic review. Electron-
ics, 10(14):1611.
Zhou, Y., Jindal-Snape, D., Topping, K., and Todman, J.
(2008). Theoretical models of culture shock and adap-
tation in international students in higher education.
Studies in Higher Education, 33(1):63–75.
CSEDU 2025 - 17th International Conference on Computer Supported Education
930