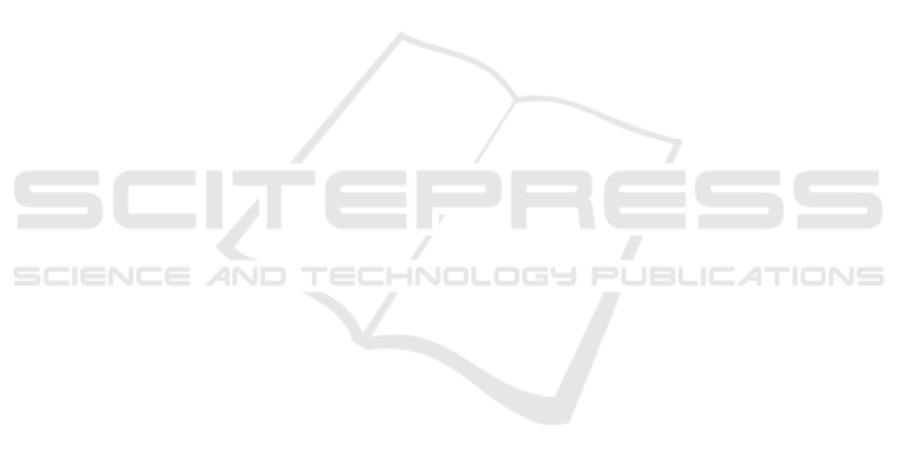
ducing training and prediction times, making it ideal
for large-scale data. Supported by the Delta BDA,
it ensures efficient deployment in MOOC environ-
ments. This research enhances online education and
big data analytics by addressing dropout issues and
improving the quality of MOOCs.
ACKNOWLEDGEMENTS
This research is funded by University of Informa-
tion Technology-Vietnam National University Ho Chi
Minh City under grant number D1-2024-69.
REFERENCES
Anand, G., Kumari, S., and Pulle, R. (2023). Fractional-
iterative bilstm classifier: A novel approach to pre-
dicting student attrition in digital academia. SSRG
International Journal of Computer Science and En-
gineering, 10(5):1–9.
Ang, K. L.-M., Ge, F. L., and Seng, K. P. (2020). Big ed-
ucational data & analytics: Survey, architecture and
challenges. IEEE access, 8:116392–116414.
Armbrust, M., Das, T., Sun, L., Yavuz, B., Zhu, S., Murthy,
M., Torres, J., van Hovell, H., Ionescu, A., Łuszczak,
A., et al. (2020). Delta lake: high-performance acid
table storage over cloud object stores. Proceedings of
the VLDB Endowment, 13(12):3411–3424.
Armbrust, M., Das, T., Torres, J., Yavuz, B., Zhu, S., Xin,
R., Ghodsi, A., Stoica, I., and Zaharia, M. (2018).
Structured streaming: A declarative api for real-time
applications in apache spark. In Proceedings of the
2018 International Conference on Management of
Data, pages 601–613.
Bahdanau, D. (2014). Neural machine translation by
jointly learning to align and translate. arXiv preprint
arXiv:1409.0473.
Bai, X., Zhang, F., Li, J., Guo, T., Aziz, A., Jin, A., and Xia,
F. (2021). Educational big data: Predictions, applica-
tions and challenges. Big Data Research, 26:100270.
Cao, W., Wang, Q., Sbeih, A., and Shibly, F. (2020). Artifi-
cial intelligence based efficient smart learning frame-
work for education platform. Inteligencia Artificial,
23(66):112–123.
Cuji Chacha, B. R., Gavilanes L
´
opez, W. L., Vicente Guer-
rero, V. X., and Villacis Villacis, W. G. (2020).
Student dropout model based on logistic regression.
In Applied Technologies: First International Con-
ference, ICAT 2019, Quito, Ecuador, December 3–
5, 2019, Proceedings, Part II 1, pages 321–333.
Springer.
Feng, W., Tang, J., and Liu, T. X. (2019). Understand-
ing dropouts in moocs. In Proceedings of the AAAI
conference on artificial intelligence, volume 33, pages
517–524.
Fu, Q., Gao, Z., Zhou, J., and Zheng, Y. (2021). Clsa:
A novel deep learning model for mooc dropout
prediction. Computers & Electrical Engineering,
94:107315.
Itti, L., Koch, C., and Niebur, E. (1998). A model of
saliency-based visual attention for rapid scene anal-
ysis. IEEE Transactions on pattern analysis and ma-
chine intelligence, 20(11):1254–1259.
Jeon, B., Park, N., and Bang, S. (2020). Dropout
prediction over weeks in moocs via interpretable
multi-layer representation learning. arXiv preprint
arXiv:2002.01598.
Kreuzberger, D., K
¨
uhl, N., and Hirschl, S. (2023). Ma-
chine learning operations (mlops): Overview, defini-
tion, and architecture. IEEE access, 11:31866–31879.
Mehrabi, M., Safarpour, A. R., and Keshtkar, A. (2022).
Massive open online courses (moocs) dropout rate in
the world: a protocol for systematic review and meta-
analysis. Interdisciplinary Journal of Virtual Learning
in Medical Sciences, 13(2):85–92.
Pala, S. K. (2021). Databricks analytics: Empowering data
processing, machine learning and real-time analytics.
Machine Learning, 10(1).
Pereira, R. T. and Zambrano, J. C. (2017). Application of
decision trees for detection of student dropout profiles.
In 2017 16th IEEE international conference on ma-
chine learning and applications (ICMLA), pages 528–
531. IEEE.
Rulinawaty, R., Priyanto, A., Kuncoro, S., Rahmawaty, D.,
and Wijaya, A. (2023). Massive open online courses
(moocs) as catalysts of change in education during un-
precedented times: A narrative review. Jurnal Peneli-
tian Pendidikan IPA, 9(SpecialIssue):53–63.
Sakboonyarat, S. and Tantatsanawong, P. (2022). Ap-
plied big data technique and deep learning for massive
open online courses (moocs) recommendation sys-
tem. ECTI Transactions on Computer and Informa-
tion Technology (ECTI-CIT), 16(4):436–447.
Talebi, K., Torabi, Z., and Daneshpour, N. (2024). Ensem-
ble models based on cnn and lstm for dropout pre-
diction in mooc. Expert Systems with Applications,
235:121187.
Wang, W., Zhao, Y., Wu, Y. J., and Goh, M. (2023). Factors
of dropout from moocs: a bibliometric review. Library
Hi Tech, 41(2):432–453.
Younus, A. M., Abumandil, M. S., Gangwar, V. P., and
Gupta, S. K. (2022). Ai-based smart education system
for a smart city using an improved self-adaptive leap-
frogging algorithm. In AI-Centric Smart City Ecosys-
tems, pages 231–245. CRC Press.
Zbakh, M., Essaaidi, M., Manneback, P., and Rong, C.
(2019). Cloud Computing and Big Data: Technolo-
gies, Applications and Security. Springer.
Zheng, L., Wang, C., Chen, X., Song, Y., Meng, Z.,
and Zhang, R. (2023). Evolutionary machine learn-
ing builds smart education big data platform: Data-
driven higher education. Applied Soft Computing,
136:110114.
BiLSTM-Attention-Delta: A Novel Framework for Predicting Dropout in MOOCs Within Big Data Environments
235