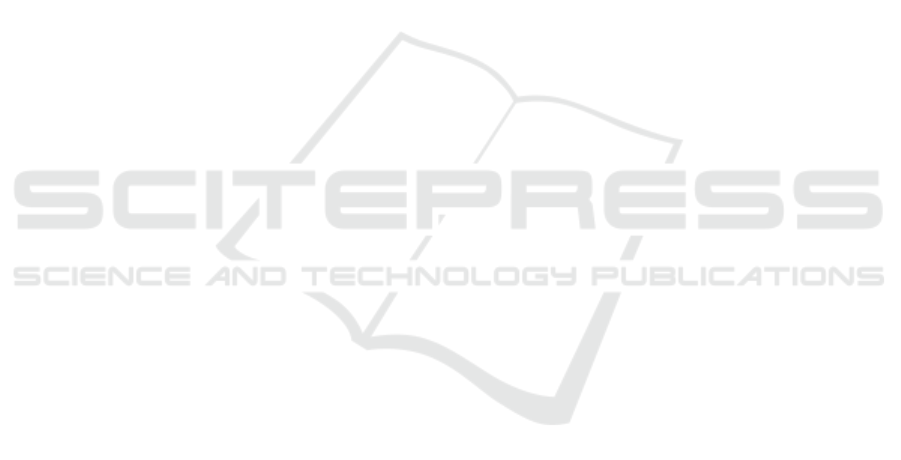
ACKNOWLEDGMENT
This research has been partly funded by the Luxem-
bourg National Research Fund (FNR) under contract
number 16327771 and has been supported by Prox-
imus Luxembourg SA. For the purpose of open ac-
cess, and in fulfillment of the obligations arising from
the grant agreement, the author has applied a Creative
Commons Attribution 4.0 International (CC BY 4.0)
license to any Author Accepted Manuscript version
arising from this submission.
REFERENCES
Bosshard, B. (2020). On the use of web assembly in a
serverless context. In Agile Processes in Software
Engineering and Extreme Programming–Workshops,
page 141.
Chadha, M., Krueger, N., John, J., Jindal, A., Gerndt, M.,
and Benedict, S. (2023). Exploring the use of we-
bassembly in hpc. In Proceedings of the 28th ACM
SIGPLAN Annual Symposium on Principles and Prac-
tice of Parallel Programming, pages 92–106.
Felter, W., Ferreira, A., Rajamony, R., and Rubio, J. (2015).
An updated performance comparison of virtual ma-
chines and linux containers. In 2015 IEEE Interna-
tional Symposium on Performance Analysis of Sys-
tems and Software (ISPASS), pages 171–172. IEEE.
Ferrari, D., Sciascio, E., Gioiosa, R., Krieger, O., Krikellas,
K., and Giuliani, G. (2021). Webassembly for cloud-
native applications: A performance evaluation. Future
Generation Computer Systems, 115:335–348.
Fiedler, M., Alrowaily, H., Menzel, K., and Stiller, B.
(2020). Performance evaluation of webassembly as a
cloud-edge continuum technology. In 2020 IEEE 6th
International Conference on Network Softwarization
(NetSoft), pages 334–340. IEEE.
Fujii, D., Matsubara, K., and Nakata, Y. (2024). State-
ful vm migration among heterogeneous webassem-
bly runtimes for efficient edge-cloud collaborations.
In Proceedings of the 7th International Workshop on
Edge Systems, Analytics and Networking, pages 19–
24.
Gackstatter, P., Frangoudis, P. A., and Dustdar, S. (2022).
Pushing serverless to the edge with webassembly run-
times. In 2022 22nd IEEE International Symposium
on Cluster, Cloud and Internet Computing (CCGrid),
pages 140–149. IEEE.
Gkonis, P., Giannopoulos, A., Trakadas, P., Masip-Bruin,
X., and D’Andria, F. (2023). A survey on iot-edge-
cloud continuum systems: Status, challenges, use
cases, and open issues. Future Internet, 15(12):383.
Hilbig, A., Lehmann, D., and Pradel, M. (2021). An empir-
ical study of real-world webassembly binaries: Secu-
rity, languages, use cases. In Proceedings of the Web
Conference 2021, pages 2696–2708.
Hockley, D. and Williamson, C. (2022). Benchmarking run-
time scripting performance in webassembly.
Kakati, S. and Brorsson, M. (2023). Webassembly be-
yond the web: A review for the edge-cloud contin-
uum. In 2023 3rd International Conference on Intel-
ligent Technologies (CONIT), pages 1–8. IEEE.
Kakati, S. and Brorsson, M. (2024a). A cross-architecture
evaluation of webassembly in the cloud-edge con-
tinuum. 2024 IEEE 24th International Symposium
on Cluster, Cloud and Internet Computing (CCGrid),
pages 337–346.
Kakati, S. and Brorsson, M. (2024b). An investigative study
of webassembly performance in cloud-to-edge. In
2024 International Symposium on Parallel Computing
and Distributed Systems (PCDS), pages 1–5.
Kjorveziroski, V., Filiposka, S., and Mishev, A. (2022).
Evaluating webassembly for orchestrated deployment
of serverless functions. In 2022 30th Telecommunica-
tions Forum (TELFOR), pages 1–4. IEEE.
Mattia, G. P. and Beraldi, R. (2021). Leveraging Reinforce-
ment Learning for online scheduling of real-time tasks
in the Edge/Fog-to-Cloud computing continuum. In
2021 IEEE 20th International Symposium on Net-
work Computing and Applications (NCA), pages 1–9.
ISSN: 2643-7929.
Mendki, P. (2020). Evaluating webassembly enabled server-
less approach for edge computing. In 2020 IEEE
Cloud Summit, pages 161–166.
Nastic, S., Pusztai, T., Morichetta, A., Pujol, V. C., Dustdar,
S., Vii, D., and Xiong, Y. (2021). Polaris Scheduler:
Edge Sensitive and SLO Aware Workload Scheduling
in Cloud-Edge-IoT Clusters. In 2021 IEEE 14th Inter-
national Conference on Cloud Computing (CLOUD),
pages 206–216. ISSN: 2159-6190.
Orive, A., Agirre, A., Truong, H.-L., Sarachaga, I., and
Marcos, M. (2022). Quality of Service Aware Or-
chestration for Cloud–Edge Continuum Applications.
Sensors, 22(5):1755. Number: 5 Publisher: Multidis-
ciplinary Digital Publishing Institute.
Pham, S., Oliveira, K., and Lung, C.-H. (2023). Webassem-
bly modules as alternative to docker containers in iot
application development. In 2023 IEEE 3rd Interna-
tional Conference on Electronic Communications, In-
ternet of Things and Big Data (ICEIB), pages 519–
524. IEEE.
Spies, B. and Mock, M. (2021). An evaluation of we-
bassembly in non-web environments. In 2021 XLVII
Latin American Computing Conference (CLEI), pages
1–10. IEEE.
Wang, W. (2022). How far we’ve come–a characterization
study of standalone webassembly runtimes. In 2022
IEEE International Symposium on Workload Charac-
terization (IISWC), pages 228–241. IEEE.
Wang, Z., Wang, J., Wang, Z., and Hu, Y. (2021).
Characterization and implication of edge webassem-
bly runtimes. In 2021 IEEE 23rd Int Conf on
High Performance Computing & Communications;
7th Int Conf on Data Science & Systems; 19th Int
Conf on Smart City; 7th Int Conf on Dependabil-
ity in Sensor, Cloud & Big Data Systems & Applica-
tion (HPCC/DSS/SmartCity/DependSys), pages 71–
80. IEEE.
Performance and Usability Implications of Multiplatform and WebAssembly Containers
25