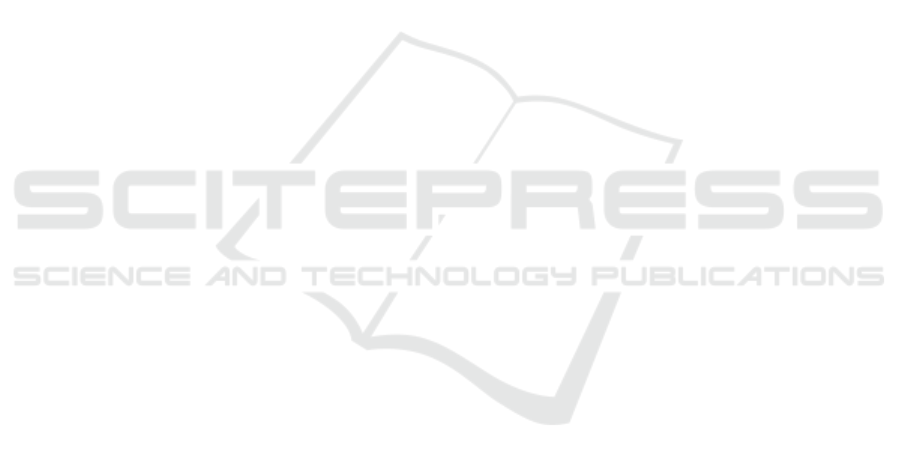
(2015). Beyond Theory and Data in Preference Mod-
eling: Bringing Humans into the Loop, page 3–18.
Springer International Publishing.
Bahcecik, S. O. (2023). I trends security politics and arti-
ficial intelligence: Key trends and debates. Interna-
tional Political Science Abstracts, 73(3):329–338.
Beaty, R. E. and Johnson, D. R. (2020). Automating cre-
ativity assessment with semdis: An open platform
for computing semantic distance. Behavior Research
Methods, 53(2):757–780.
Ca
˜
nete, J., Chaperon, G., Fuentes, R., Ho, J.-H., Kang, H.,
and P
´
erez, J. (2020). Spanish pre-trained bert model
and evaluation data. In PML4DC at ICLR 2020.
Cetinic, E. and She, J. (2021). Understanding and creating
art with ai: Review and outlook.
Chollet, F. (2016). Xception: Deep learning with depth-
wise separable convolutions. 2017 IEEE Conference
on Computer Vision and Pattern Recognition (CVPR),
pages 1800–1807.
Creely, E. and Blannin, J. (2023). The implications of gen-
erative ai for creative composition in higher education
and initial teacher education. ASCILITE Publications,
page 357–361.
Cropley, D. H. and Marrone, R. L. (2022). Automated scor-
ing of figural creativity using a convolutional neural
network. Psychology of Aesthetics, Creativity, and the
Arts.
Davis, J. L., Shank, D. B., Love, T. P., Stefanik, C., and
Wilson, A. (2022). Gender Dynamics in Human-AI
Role-Taking, page 1–22. Emerald Publishing Limited.
Devedzic, V. (2020). Is this artificial intelligence? Facta
universitatis - series: Electronics and Energetics,
33(4):499–529.
Easton, K., Potter, S., Bec, R., Bennion, M., Christensen,
H., Grindell, C., Mirheidari, B., Weich, S., de Witte,
L., Wolstenholme, D., and Hawley, M. S. (2019). A
virtual agent to support individuals living with physi-
cal and mental comorbidities: Co-design and accept-
ability testing. Journal of Medical Internet Research,
21(5):e12996.
Ferrara, S. and Qunbar, S. (2022). Validity arguments
for ai-based automated scores: Essay scoring as an
illustration. Journal of Educational Measurement,
59(3):288–313.
Gado, S., Kempen, R., Lingelbach, K., and Bipp, T. (2021).
Artificial intelligence in psychology: How can we
enable psychology students to accept and use artifi-
cial intelligence? Psychology Learning & Teaching,
21(1):37–56.
Gigi, A. (2015). Human figure drawing (hfd) test is affected
by cognitive style. Clinical and Experimental Psy-
chology, 02.
Harr
´
e, M. S. and El-Tarifi, H. (2023). Testing game the-
ory of mind models for artificial intelligence. Games,
15(1):1.
He, K., Zhang, X., Ren, S., and Sun, J. (2015). Deep resid-
ual learning for image recognition.
Imuta, K., Scarf, D., Pharo, H., and Hayne, H. (2013).
Drawing a close to the use of human figure drawings
as a projective measure of intelligence. PLoS ONE, 8.
Joulin, A., Grave, E., Bojanowski, P., Douze, M., J
´
egou,
H., and Mikolov, T. (2016). Fasttext.zip: Com-
pressing text classification models. arXiv preprint
arXiv:1612.03651.
Kvam, P. D., Sokratous, K., Fitch, A., and Hintze, A.
(2023). Using artificial intelligence to fit, compare,
evaluate, and discover computational models of deci-
sion behavior.
Lee, S. W., Kwak, D. S., Jung, I. S., Kwak, J. H., Park,
J. H., Hong, S. M., Lee, C. B., Park, Y. S., Kim, D. S.,
Choi, W. H., and Ahn, Y. H. (2015). Partial androgen
insensitivity syndrome presenting with gynecomastia.
Endocrinology and Metabolism, 30(2):226.
Liu, J., Xue, Z., Vann, K. R., Shi, X., and Kutateladze, T. G.
(2020). Protocol for biochemical analysis and struc-
ture determination of the zz domain of the e3 ubiquitin
ligase herc2. STAR Protocols, 1.
O’Shea, K. and Nash, R. (2015). An introduction to convo-
lutional neural networks.
Patterson, J. D., Barbot, B., Lloyd-Cox, J., and Beaty, R. E.
(2023). Audra: An automated drawing assessment
platform for evaluating creativity. Behavior Research
Methods, 56(4):3619–3636.
Pezzulo, G., Parr, T., Cisek, P., Clark, A., and Friston, K.
(2023). Generating meaning: Active inference and
the scope and limits of passive ai.
Røed, R. K., Baugerud, G. A., Hassan, S. Z., Sabet, S. S.,
Salehi, P., Powell, M. B., Riegler, M. A., Halvorsen,
P., and Johnson, M. S. (2023). Enhancing questioning
skills through child avatar chatbot training with feed-
back. Frontiers in Psychology, 14.
Searle, J. R. (2018). Minds, Brains and Programs, page
18–40. Routledge.
Shaban-Nejad, A., Michalowski, M., Bianco, S., Brown-
stein, J. S., Buckeridge, D. L., and Davis, R. L.
(2022). Applied artificial intelligence in health-
care: Listening to the winds of change in a post-
covid-19 world. Experimental Biology and Medicine,
247(22):1969–1971.
Sheng, L., Yang, G., Pan, Q., Xia, C., and Zhao, L.
(2019). Synthetic house-tree-person drawing test: A
new method for screening anxiety in cancer patients.
Journal of Oncology, 2019.
Stojnic, G., Gandhi, K., Yasuda, S., Lake, B. M., and Dil-
lon, M. R. (2022). Commonsense psychology in hu-
man infants and machines.
Szegedy, C., Vanhoucke, V., Ioffe, S., Shlens, J., and Wojna,
Z. (2015). Rethinking the inception architecture for
computer vision.
Tan, M. and Le, Q. V. (2020). Efficientnet: Rethinking
model scaling for convolutional neural networks.
Tan, T., Rodriguez-Ruiz, A., Zhang, T., Xu, L., Beets-Tan,
R. G. H., Shen, Y., Karssemeijer, N., Xu, J., Mann,
R. M., and Bao, L. (2023). Multi-modal artificial intel-
ligence for the combination of automated 3d breast ul-
trasound and mammograms in a population of women
with predominantly dense breasts. Insights into Imag-
ing, 14(1).
Wang, W., Kofler, L., Lindgren, C., Lobel, M., Murphy, A.,
Tong, Q., and Pickering, K. (2023). Ai for psycho-
Analysing the Impact of Images and Text for Predicting Human Creativity Through Encoders
23