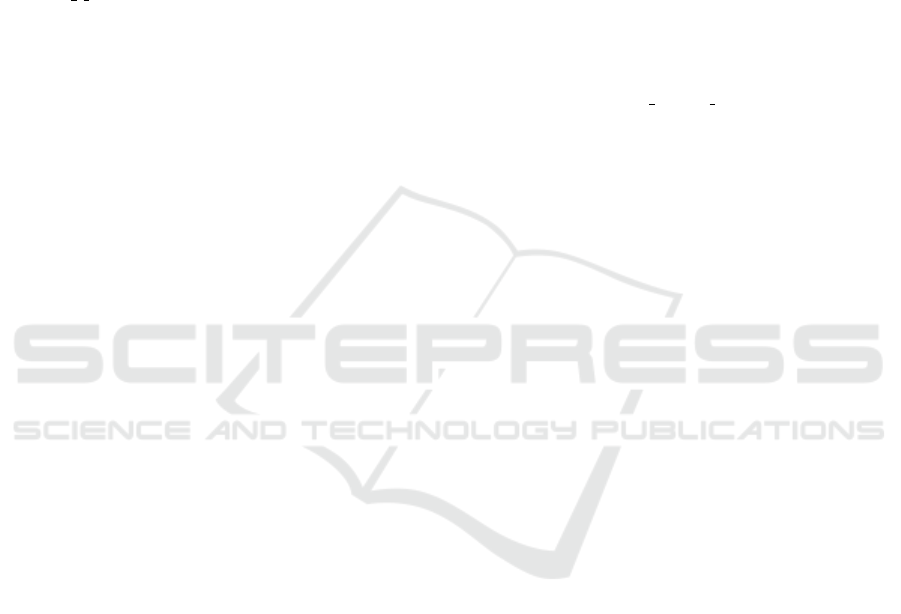
Backhaus, K., Erichson, B., Plinke, W., and Weiber, R.
(2018). Multivariate Analysemethoden. Springer
Berlin Heidelberg, Berlin/Heidelberg, 15 edition.
Eckhoff, Halmos, and German (2013). Potentials and limi-
tations of green light optimal speed advisory systems.
IEEE Vehicular Networking Conference.
European Commission (2016). A european strategy on co-
operative intelligent transport systems, a milestone to-
wards cooperative, connected and automated mobility.
Eurostat (2023). Statistics — eurostat: Stock of ve-
hicles by category and nuts 2 regions. url:
https://ec.europa.eu/eurostat/databrowser/view/
tran r vehst/default/table?lang=en. accessed:
18.10.2024.
HomChaudhuri, B., Vahidi, A., and Pisu, P. (2017). Fast
model predictive control-based fuel efficient control
strategy for a group of connected vehicles in urban
road conditions. IEEE Transactions on Control Sys-
tems Technology, 25(2):760–767.
INRIX (2024). Data and metrics definitions. url:
https://docs.inrix.com/ra/dataandmetrics/. accessed:
15.01.2025.
Kamal, M. A. S., Taguchi, S., and Yoshimura, T. (2015). In-
tersection vehicle cooperative eco-driving in the con-
text of partially connected vehicle environment. In
2015 IEEE 18th Int. Conference on Intelligent Trans-
portation Systems, pages 1261–1266. IEEE.
Katsaros, Kernchen, Dianati, and Rieck (2011). Perfor-
mance study of a green light optimized speed advisory
(glosa) application using an integrated cooperative its
simulation platform. International Wireless Commu-
nications & Mobile Computing Conference.
Khayyat, M., Gabriele, A., Mancini, F., Arrigoni, S., and
Braghin, F. (2024). Enhanced traffic light guidance for
safe and energy-efficient driving: A study on multiple
traffic light advisor (mtla) and 5g integration. Journal
of Intelligent & Robotic Systems, 110(2).
Lebre, M.-A., Le Mou
¨
el, F., M
´
enard, E., Garnault, A.,
Brada
¨
ı, B., and Picron, V. (2015). Real scenario and
simulations on glosa traffic light system for reduced
co2 emissions, waiting time and travel time.
Liu, B. and El Kamel, A. (2016). V2x-based decentralized
cooperative adaptive cruise control in the vicinity of
intersections. IEEE Transactions on Intelligent Trans-
portation Systems, 17(3):644–658.
Milan
´
es, V. and Shladover, S. E. (2014). Modeling coopera-
tive and autonomous adaptive cruise control dynamic
responses using experimental data. Transportation
Research Part C: Emerging Technologies, 48:285–
300.
Presinger, C. (2021). Calibration and validation of meso-
scopic traffic flow simulation. PhD thesis, Technical
University Graz.
Rios-Torres, J. and Malikopoulos, A. A. (2017). Auto-
mated and cooperative vehicle merging at highway
on-ramps. IEEE Transactions on Intelligent Trans-
portation Systems, 18(4):780–789.
Rogers, E. M. (2003). Diffusion of innovations. Free Press,
New York, 5th ed. edition.
Statistik Austria (2024). Kfz-bestand. url:
https://www.statistik.at/statistiken/tourismus-und-
verkehr/fahrzeuge/kfz-bestand. accessed: 18.10.2024.
SUMO (2025a). Glosa - sumo documentation. url:
https://sumo.dlr.de/docs/simulation/glosa.html.
accessed: 15.01.2025.
SUMO (2025b). Tripinfo - sumo documentation.
url: https://sumo.dlr.de/docs/simulation/output/ trip-
info.html. accessed: 15.01.2025.
Umweltbundesamt (2024a). Straßenverkehrsl
¨
arm. url:
https://www.umweltbundesamt.de/themen/laerm/
verkehrslaerm/strassenverkehrslaerm#larmsanierung-
larmschutz-an-bestehenden-strassen. accessed:
18.10.2024.
Umweltbundesamt (2024b). Umwelt - bestand am
1. januar 2024 nach umwelt-merkmalen. url:
https://www.kba.de/de/statistik/fahrzeuge/bestand/
umwelt/2024/2024 umwelt uebersicht.html. ac-
cessed: 18.10.2024.
Walch, M., Neubauer, M., Schildorfer, W., and Schirrer, A.
(2024). Modelling interrelations between c-its impact
categories: a system-dynamics approach using causal
loop diagrams. European Transport Research Review,
16(3):1–27.
Walch, M., Schirrer, A., and Neubauer, M. (2025). Im-
pact assessment of cooperative intelligent transport
systems (c-its) - a structured literature review. Euro-
pean Transport Research Review.
Wang, Z., Chen, X., Ouyang, Y., and Li, M. (2015). Emis-
sion mitigation via longitudinal control of intelligent
vehicles in a congested platoon. Computer-Aided
Civil and Infrastructure Engineering, 30(6):490–506.
Xiao, L., Wang, M., Schakel, W., and van Arem, B.
(2018). Unravelling effects of cooperative adaptive
cruise control deactivation on traffic flow characteris-
tics at merging bottlenecks. Transportation Research
Part C: Emerging Technologies, 96:380–397.
Xiao, L., Wang, M., and van Arem, B. (2017). Realistic
car-following models for microscopic simulation of
adaptive and cooperative adaptive cruise control ve-
hicles. Transportation Research Record: Journal of
the Transportation Research Board, 2623(1):1–9.
Xin, Q., Fu, R., Yuan, W., Liu, Q., and Yu, S. (2018). Pre-
dictive intelligent driver model for eco-driving using
upcoming traffic signal information. Physica A: Sta-
tistical Mechanics and its Applications, 508:806–823.
Towards Synergistic Effects of C-ITS Services: Assessing the Joint Impact of GLOSA and CACC on Traffic Efficiency and Sustainability
349