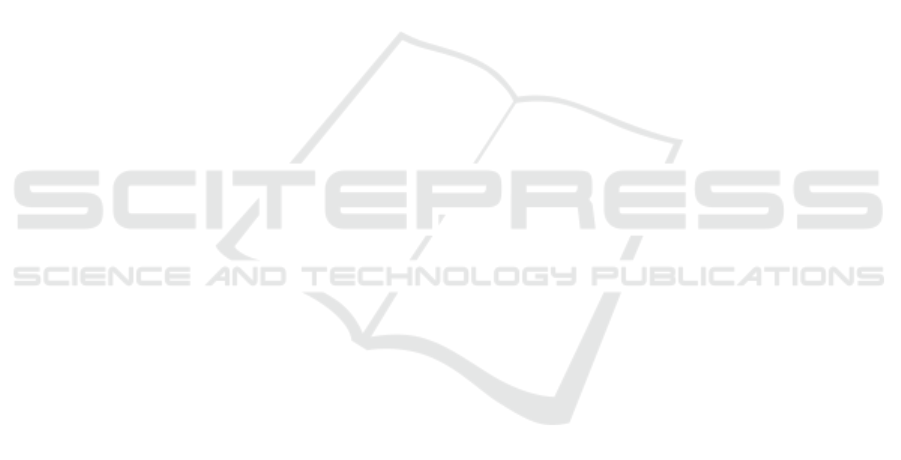
5 CONCLUSION
This work applies the SD modeling technique to as-
sess the impact of the C-ITS service CR on traffic effi-
ciency. By using the developed SFM, multiple scenar-
ios were defined to test different parameter combina-
tions and compare the results. The findings highlight
the influence of the C-ITS EQUIPMENT RATE, DE-
LAY THRESHOLD, and UPDATE INTERVAL on re-
sults, with all scenarios showing improvements over
the Base Scenario to varying degrees.
Key findings indicate that low C-ITS EQUIP-
MENT RATEs yield significant traffic efficiency im-
provements. However, rerouting all C-ITS equipped
vehicles (100% C-ITS EQUIPMENT RATE) to the
route with the lowest Delay can cause overloads, par-
ticularly with long UPDATE INTERVALs, leading to
Delays. Introducing a DELAY THRESHOLD helps
avoid rerouting for minimal Delays, though this re-
duces overall efficiency as it takes some Delay to ini-
tiate rerouting. High UPDATE INTERVALs in com-
bination with a high C-ITS EQUIPMENT RATE lead
to route overloads. A more selective rerouting strat-
egy, where only a portion of equipped vehicles are
rerouted for optimal performance, is recommended.
Future extensions of the model could include pre-
dictive rerouting based on anticipated outcomes, in-
tegration of different rerouting criteria such as Free
Capacity or Travel Time, and the inclusion of stochas-
tic elements to account for compliance probabilities.
Additionally, the model could be expanded to assess
other impact categories such as sustainability, consid-
ering factors like CO
2
emissions and noise levels, al-
lowing for rerouting criteria that balance both traffic
efficiency and sustainability.
ACKNOWLEDGEMENTS
The work leading to the results was funded by the
State of Upper Austria within the project SUBSTAN-
TIATE (grant no. 895978), managed by the Austrian
Research Promotion Agency (FFG).
REFERENCES
Agriesti, S., Studer, L., Marchionni, G., Gandini, P., and
Qu, X. (2020). Roadworks warning - closure of a lane,
the impact of c-its messages. Infrastructures, 5(3):27.
Breitenecker, F., Judex, F., Popper, N., Breitenecker, K.,
Mathe, A., and Mathe, A. (2008). Love emotions be-
tween laura and petrarch an approach by mathematics
and system dynamics. Journal of Computing and In-
formation Technology, 16(4):255.
Drake, Joseph L. Schofer, and A. D. May (1966). A statis-
tical analysis of speed-density hypotheses. Highway
Research Record 154, pages 53–87.
European Commission (2016). A european strategy on co-
operative intelligent transport systems, a milestone to-
wards cooperative, connected and automated mobility.
EVIS.AT (2024). Evis.at - echtzeit verkehrsinformation
straße
¨
osterreich.
Forrester, J. W. (1969). Industrial dynamics: Students’ edi-
tion. MIT Press, Cambridge, Mass, 6th printing edi-
tion.
Katsaros, K., Kernchen, R., Dianati, M., Rieck, D., and Zi-
noviou, C. (2011). Application of vehicular communi-
cations for improving the efficiency of traffic in urban
areas. Wireless Communications and Mobile Comput-
ing, 11(12):1657–1667.
Kim, K., Koo, S., and Choi, J.-W. (2020). Analysis on path
rerouting algorithm based on v2x communication for
traffic flow improvement. In 2020 International Con-
ference on Information and Communication Technol-
ogy Convergence (ICTC), pages 251–254. IEEE.
Lu, N., Cheng, N., Zhang, N., Shen, X., and Mark, J. W.
(2014). Connected vehicles: Solutions and chal-
lenges. IEEE Internet of Things Journal, 1(4):289–
299.
Pribyl, O., Blokpoel, R., and Matowicki, M. (2020). Ad-
dressing eu climate targets: Reducing co2 emissions
using cooperative and automated vehicles. Trans-
portation Research Part D: Transport and Environ-
ment, 86:102437.
Rodrigue, J.-P. (2020). The geography of transport systems.
Routledge/Taylor & Francis Group, Abingdon Oxon
and New York NY, fifth edition edition.
Soon, K. L., Lim, J. M.-Y., and Parthiban, R. (2019). Coor-
dinated traffic light control in cooperative green vehi-
cle routing for pheromone-based multi-agent systems.
Applied Soft Computing, 81:105486.
Statista (2023). Number of connected cars in european
countries in 2023 — statista.
Walch, M., Neubauer, M., Schildorfer, W., and Schirrer, A.
(2024). Modelling interrelations between c-its impact
categories: a system-dynamics approach using causal
loop diagrams. European Transport Research Review,
16(3):1–27.
Walch, M., Schirrer, A., and Neubauer, M. (2025). Im-
pact assessment of cooperative intelligent transport
systems (c-its) - a structured literature review. Euro-
pean Transport Research Review.
Wardrop, J. G. (1952). Some theoretical aspects of road
traffic research. Proceedings of the Institution of Civil
Engineers, 1(3):325–362.
Wedel, J. W., Sch
¨
unemann, B., and Radusch, I. (2009).
V2x-based traffic congestion recognition and avoid-
ance. In I-SPAN 2009, pages 637–641, Los Alamitos,
Calif. IEEE Computer Society.
Wu, X., Zhao, X., Xin, Q., Yang, Q., Yu, S., and Sun, K.
(2019). Dynamic cooperative speed optimization at
signalized arterials with various platoons. Transporta-
tion Research Record: Journal of the Transportation
Research Board, 2673(5):528–537.
VEHITS 2025 - 11th International Conference on Vehicle Technology and Intelligent Transport Systems
356