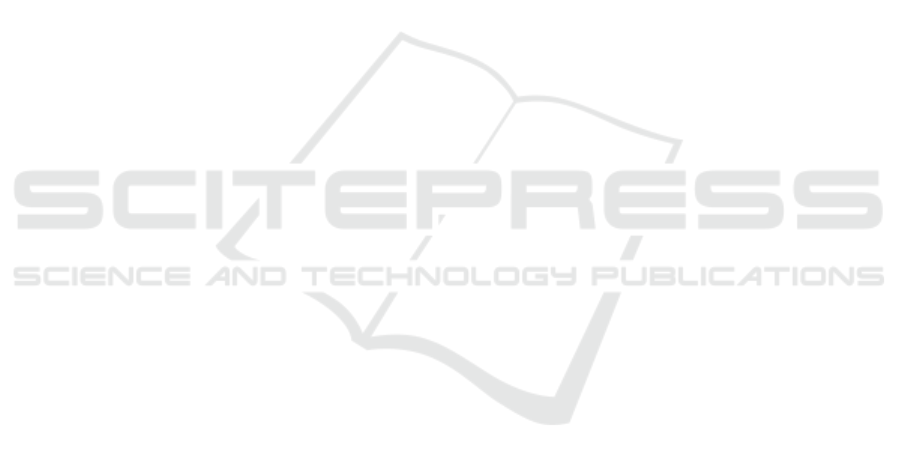
essential for comprehensive traffic management solu-
tions.
ACKNOWLEDGEMENTS
This work is supported by the European Union’s
Horizon Europe research and innovation program un-
der the agreement number Augmented CCAM No
101069717.
REFERENCES
Abboud, K., Omar, H. A., and Zhuang, W. (2016). Inter-
working of DSRC and cellular network technologies
for V2X communications: A survey. IEEE Transac-
tions on Vehicular Technology, 65(12):9457–9470.
Algomaiah, M. and Li, Z. (2021). Exploring work zone late
merge strategies with and without enabling connected
vehicles technologies. Transportation Research Inter-
disciplinary Perspectives, 9:100316.
Algomaiah, M. and Li, Z. (2022). Enhancing work zone
capacity by a cooperative late merge system using de-
centralized and centralized control strategies. Jour-
nal of Transportation Engineering, Part A: Systems,
148(2):04021107.
Cao, D., Wu, J., Wu, J., Kulcs
´
ar, B., and Qu, X.
(2021). A platoon regulation algorithm to im-
prove the traffic performance of highway work zones.
Computer-Aided Civil and Infrastructure Engineer-
ing, 36(7):941–956.
Dixon, K. K., Hummer, J. E., and Lorscheider, A. R.
(1996). Capacity for north carolina freeway work
zones. Transportation Research Record, 1529(1):27–
34.
Ghanadbashi, S. and Golpayegani, F. (2022). Using ontol-
ogy to guide reinforcement learning agents in unseen
situations: A traffic signal control system case study.
Applied Intelligence, 52(2):1808–1824.
Ghanadbashi, S., Malekjafarian, A., and Gholpayegani, F.
(2024a). CAV-enabled ontology-driven evacuation ap-
proach for dynamic emergency. In Proceedings of
the Civil Engineering Research Association of Ireland
(CERAI).
Ghanadbashi, S., Safavifar, Z., Taebi, F., and Golpayegani,
F. (2024b). Handling uncertainty in self-adaptive
systems: an ontology-based reinforcement learning
model. Journal of Reliable Intelligent Environments,
10(1):19–44.
Golpayegani, F., Ghanadbashi, S., and Riad, M. (2021). Ur-
ban emergency management using intelligent traffic
systems: Challenges and future directions. In 2021
IEEE International Smart Cities Conference (ISC2),
pages 1–4. IEEE.
Guo, J., Ghanadbashi, S., Wang, S., and Golpayegani, F.
(2023). Urban traffic signal control at the edge: An
ontology-enhanced deep reinforcement learning ap-
proach. In 2023 IEEE 26th International Conference
on Intelligent Transportation Systems (ITSC), pages
6027–6033. IEEE.
Kang, K.-P., Chang, G.-L., and Paracha, J. (2006). Dynamic
late merge control at highway work zones: Evalua-
tions, observations, and suggestions. Transportation
Research Record, 1948(1):86–95.
Kurker, M., Fournier, C., Zhao, Q., Hakimi, S., Qi, Y., Tang,
S., Liu, R., Juri, N. R., Duthie, J., Machemehl, R.,
et al. (2014). Minimizing user delay and crash poten-
tial through highway work zone planning. Technical
report, Texas. Dept. of Transportation. Research and
Technology Implementation Office.
Liu, H., Wei, H., Zuo, T., Li, Z., and Yang, Y. J. (2017).
Fine-tuning adas algorithm parameters for optimizing
traffic safety and mobility in connected vehicle envi-
ronment. Transportation Research Part C: Emerging
Technologies, 76:132–149.
Meng, Q. and Weng, J. (2013). Uncertainty analysis of acci-
dent notification time and emergency medical service
response time in work zone traffic accidents. Traffic
Injury Prevention, 14(2):150–158.
Mikolasek, I., Ghanadbashi, S., Afraz, N., and Gol-
payegani, F. (2024). Data sharing at the edge of
the network: A disturbance resilient multi-modal ITS.
arXiv preprint arXiv:2405.12431.
Ramadan, O. E. and Sisiopiku, V. P. (2016). Evaluation of
merge control strategies at interstate work zones un-
der peak and off-peak traffic conditions. Journal of
Transportation Technologies, 6(3):118–130.
Ramadan, O. E. and Sisiopiku, V. P. (2018). Modeling high-
way performance under various short-term work zone
configurations. Journal of Transportation Engineer-
ing, Part A: Systems, 144(9):04018050.
Ren, T., Xie, Y., and Jiang, L. (2020a). Cooperative high-
way work zone merge control based on reinforcement
learning in a connected and automated environment.
Transportation Research Record, 2674(10):363–374.
Ren, T., Xie, Y., and Jiang, L. (2020b). New Eng-
land Merge: A novel cooperative merge control
method for improving highway work zone mobility
and safety. Journal of Intelligent Transportation Sys-
tems, 25(1):107–121.
Riad, M., Ghanadbashi, S., and Golpayegani, F. (2022).
Run-time norms synthesis in dynamic environments
with changing objectives. In Irish Conference on Ar-
tificial Intelligence and Cognitive Science, pages 462–
474. Springer.
Stewart, R. (1990). Pre-hospital care of trauma. McMurtry
RY and McLellan BA, Management of Blunt Trauma,
Baltimore, MD: Williams and Wilkins, pages 23–29.
Wu, J., Kulcs
´
ar, B., Ahn, S., and Qu, X. (2020). Emergency
vehicle lane pre-clearing: From microscopic cooper-
ation to routing decision making. Transportation Re-
search Part B: Methodological, 141:223–239.
Adaptive Traffic Management for Emergency Vehicles in Work Zones
373