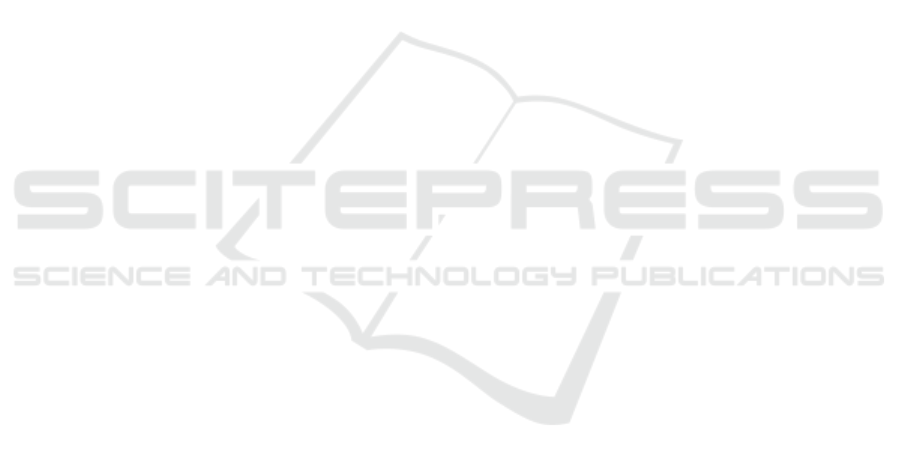
dation facilitates transparency in risk communication
and fosters informed decision-making among cloud
service providers (CSPs) and consumers.
Moving forward, one key area of focus will be in-
tegrating this ontology into an expert system capable
of actively tracking the occurrence of preconditions
linked to potential hazards. This system could mon-
itor real-time data to detect risk factors or precondi-
tions as they emerge, triggering preventive mecha-
nisms proactively to avoid the manifestation of haz-
ardous events. By embedding such predictive func-
tionality, we aim to shift risk management from a
reactive to a more proactive stance, significantly en-
hancing the resilience of federated cloud services.
In addition, we plan to develop a complementary
system for post-event management. Should a haz-
ardous event occur despite preventive measures, this
system would activate remediation strategies, mitigat-
ing the impact on associated postconditions. This
dual-component approach would provide a compre-
hensive risk management framework, covering both
preventive actions and responsive remedies within
SLAs.
Beyond these technical advancements, we envi-
sion continuous collaboration with industry stake-
holders and researchers to enrich the ontology’s ex-
pressiveness, ensuring it remains a robust, adaptable
resource that reflects emerging needs and complexi-
ties within the cloud federation domain.
ACKNOWLEDGEMENTS
This research activity was conducted from the Dutch
ECOFED project which is part of the integrated IP-
CEI CIS project. The early foundation of this research
was established during the Gaia-X Tech-X hackathon
in 2024, in which our participation was facilitated by
the Dutch Gaia-X hub part of the CoE DSC.
REFERENCES
Alkhamees, S. (2022). Sla negotiation and renegotiation
in cloud sla management: Issue and challenges. In
Hussain, W. and Jan, M. A., editors, IoT as a Ser-
vice, pages 159–170, Cham. Springer International
Publishing.
Allemang, D., Hendler, J., and Gandon, F. (2020). Semantic
Web for the Working Ontologist: Effective Modeling
for Linked Data, RDFS, and OWL, volume 33. As-
sociation for Computing Machinery, New York, NY,
USA, 3 edition.
Aust, J. and Pons, D. J. (2020). A systematic methodology
for developing bowtie in risk assessment: Application
to borescope inspection. Aerospace.
Bermbach, D., Kurze, T., and Tai, S. (2013). Cloud federa-
tion: Effects of federated compute resources on qual-
ity of service and cost. In 2013 IEEE International
Conference on Cloud Engineering (IC2E), pages 31–
37.
Blumauer, A., Nagy, H., and edition mono/monochrom
(2020). The Knowledge Graph Cookbook: Recipes
that Work. edition mono/monochrom.
Bonfiglio, F. (2021). Gaia-x, vision & strategy. https://gaia-
x.eu/sites/default/files/2021-12/Vision & Strategy.pdf.
CEO Gaia-X, December 16, 2021.
De Filippi, P. and McCarthy, S. (2012). Cloud computing:
Centralization and data sovereignty. European Jour-
nal of Law and Technology, 3(2).
Hogan, A. (2020). Web of data. The Web of Data.
Holfelder, W., Mayer, A., and Baumgart, T. (2022).
Sovereign cloud technologies for scalable data spaces.
In Designing Data Spaces, pages 419–436. Springer.
Iorio, M., Risso, F., Palesandro, A., Camiciotti, L., and
Manzalini, A. (2022). Computing without borders:
The way towards liquid computing. IEEE Transac-
tions on Cloud Computing.
Karamanlioglu, A. and Alpaslan, F. N. (2018). An
ontology-based expert system to detect service level
agreement violations. In Shishkov, B., editor, Busi-
ness Modeling and Software Design, pages 362–371,
Cham. Springer International Publishing.
Kbah, Z., Erdil, N. O., and Aqlan, F. (2020). Risk assess-
ment in oil and gas industry using simulation and bow-
tie analysis. International Journal of Industrial Engi-
neering: Theory, Applications and Practice, 27(1).
Kluwer, W. (2017). The history of bowtie.
https://www.wolterskluwer.com/en/solutions/
enablon/bowtie/expert-insights/barrier-based-risk-
management-knowledge-base/the-historie-of-bowtie.
Kluwer, W. (2019). The bowtie method. https:
//www.wolterskluwer.com/en/solutions/enablon/
bowtie/expert-insights/barrier-based-risk-
management-knowledge-base/the-bowtie-method.
Kurze, T., Klems, M., Bermbach, D., Lenk, A., Tai, S., and
Kunze, M. (2011). Cloud federation. The Second In-
ternational Conference on Cloud Computing, GRIDs,
and Virtualization, pages 32–38.
Labidi, T., Mtibaa, A., and Brabra, H. (2016). Cslaonto:
A comprehensive ontological sla model in cloud com-
puting. Journal on Data Semantics, 5.
L
ˆ
e, L.-S. and Nguyen, T.-V. (2020). Digitizing service level
agreements in service-oriented enterprise architecture.
SN Computer Science, 1(5):257.
McLeod, R. W. and Bowie, P. (2020). Guidance on cus-
tomising bowtie analysis for use in healthcare. In
Charles, R. and Golightly, D., editors, Contempo-
rary Ergonomics and Human Factors 2020, CIEHF.
CIEHF, CIEHF. Based on the CIEHF white paper
‘Human factors in barrier management’.
Mustafa, E. M., Saad, M. M., and Rizkallah, L. W. (2023).
Building an enhanced case-based reasoning and rule-
OTRA: A Risk Management Ontology for Transparent Service Level Agreements in Federated Cloud Environment
125