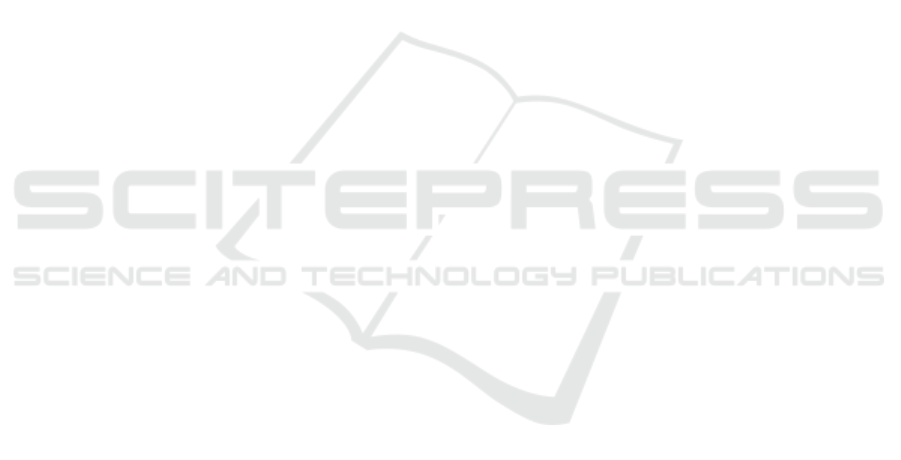
strategies in smart agriculture. IEEE Access, 9:32517–
32548.
Ibraiwish, H., Eltokhey, M. W., and Alouini, M.-S. (2024).
Uav-assisted vlc using led-based grow lights in pre-
cision agriculture systems. IEEE Internet of Things
Magazine, 7(3):100–105.
Jani, K. A. and Chaubey, N. K. (2022). A novel model
for optimization of resource utilization in smart agri-
culture system using iot (smaiot). IEEE Internet of
Things Journal, 9(13):11275–11282.
Jasim, A. N., Fourati, L. C., and Albahri, O. S. (2023). Eval-
uation of unmanned aerial vehicles for precision agri-
culture based on integrated fuzzy decision-making ap-
proach. IEEE Access, 11:75037–75062.
Liakos, K. G., Busato, P., Moshou, D., Pearson, S., and
Bochtis, D. (2018). Machine learning in agriculture:
A review. Sensors, 18(8).
Lin, Y.-B., Chen, W.-E., and Chang, T. C.-Y. (2023).
Moving from cloud to fog/edge: The smart agricul-
ture experience. IEEE Communications Magazine,
61(12):86–92.
Marwa, C., Othman, S. B., and Sakli, H. (2020). Iot based
low-cost weather station and monitoring system for
smart agriculture. In 2020 20th International Confer-
ence on Sciences and Techniques of Automatic Control
and Computer Engineering (STA), pages 349–354.
Mohyuddin, G., Khan, M. A., Haseeb, A., Mahpara, S.,
Waseem, M., and Saleh, A. M. (2024). Evaluation
of machine learning approaches for precision farming
in smart agriculture system: A comprehensive review.
IEEE Access, 12:60155–60184.
Mukhamediev, R. I., Yakunin, K., Aubakirov, M., As-
sanov, I., Kuchin, Y., Symagulov, A., Levashenko,
V., Zaitseva, E., Sokolov, D., and Amirgaliyev, Y.
(2023). Coverage path planning optimization of het-
erogeneous uavs group for precision agriculture. IEEE
Access, 11:5789–5803.
Naseer, A., Shmoon, M., Shakeel, T., Ur Rehman, S., Ah-
mad, A., and Gruhn, V. (2024). A systematic litera-
ture review of the iot in agriculture—global adoption,
innovations, security, and privacy challenges. IEEE
Access, 12:60986–61021.
N
´
obrega, L., Gonc¸alves, P., Pedreiras, P., and Pereira, J.
(2019). An iot-based solution for intelligent farming.
Sensors, 19(3).
Pallagani, V., Khandelwal, V., Chandra, B., Udutalapally,
V., Das, D., and P. Mohanty, S. (2019). dcrop: A deep-
learning based framework for accurate prediction of
diseases of crops in smart agriculture. In 2019 IEEE
International Symposium on Smart Electronic Systems
(iSES) (Formerly iNiS), pages 29–33.
Panjaitan, S. D., Dewi, Y. S. K., Hendri, M. I., Wicaksono,
R. A., and Priyatman, H. (2022). A drone technology
implementation approach to conventional paddy fields
application. IEEE Access, 10:120650–120658.
Patil, P., Kestur, R., Rao, M., and C†, A. (2023). Iot based
data sensing system for autogrow, an autonomous
greenhouse system for precision agriculture. In 2023
IEEE Applied Sensing Conference (APSCON), pages
1–3.
Reddy Maddikunta, P. K., Hakak, S., Alazab, M., Bhat-
tacharya, S., Gadekallu, T. R., Khan, W. Z., and Pham,
Q.-V. (2021). Unmanned aerial vehicles in smart agri-
culture: Applications, requirements, and challenges.
IEEE Sensors Journal, 21(16):17608–17619.
Roux, J., Escriba, C., Fourniols, J.-Y., and Soto-Romero, G.
(2018). A new bi-frequency soil smart sensing mois-
ture and salinity for connected sustainable agriculture.
Journal of Sensor Technology, 09.
Shaikh, F. K., Karim, S., Zeadally, S., and Nebhen, J.
(2022a). Recent trends in internet-of-things-enabled
sensor technologies for smart agriculture. IEEE Inter-
net of Things Journal, 9(23):23583–23598.
Shaikh, F. K., Karim, S., Zeadally, S., and Nebhen, J.
(2022b). Recent trends in internet-of-things-enabled
sensor technologies for smart agriculture. IEEE Inter-
net of Things Journal, 9(23):23583–23598.
Sharma, A., Jain, A., Gupta, P., and Chowdary, V. (2021).
Machine learning applications for precision agricul-
ture: A comprehensive review. IEEE Access, 9:4843–
4873.
Taghizadeh-Mehrjardi, R., Nabiollahi, K., Rasoli, L., Kerry,
R., and Scholten, T. (2020). Land suitability assess-
ment and agricultural production sustainability using
machine learning models. Agronomy, 10(4).
Talavera, J. M., Tob
´
on, L. E., G
´
omez, J. A., Culman, M. A.,
Aranda, J. M., Parra, D. T., Quiroz, L. A., Hoyos, A.,
and Garreta, L. E. (2017). Review of iot applications
in agro-industrial and environmental fields. Comput-
ers and Electronics in Agriculture, 142:283–297.
Toscano, F., Fiorentino, C., Capece, N., Erra, U., Travascia,
D., Scopa, A., Drosos, M., and D’Antonio, P. (2024).
Unmanned aerial vehicle for precision agriculture: A
review. IEEE Access, 12:69188–69205.
Verma, M., Lafarga, V., Dehaeze, T., and Collette, C.
(2020). Multi-degree of freedom isolation system with
high frequency roll-off for drone camera stabilization.
IEEE Access, 8:176188–176201.
Villa-Henriksen, A., Edwards, G. T., Pesonen, L. A., Green,
O., and Sørensen, C. A. G. (2020). Internet of
things in arable farming: Implementation, applica-
tions, challenges and potential. Biosystems Engineer-
ing, 191:60–84.
Vitali, G., Francia, M., Golfarelli, M., and Canavari, M.
(2021). Crop management with the iot: An interdisci-
plinary survey. Agronomy, 11(1).
Wilson, A. N., Gupta, K. A., Koduru, B. H., Kumar, A.,
Jha, A., and Cenkeramaddi, L. R. (2023). Recent ad-
vances in thermal imaging and its applications using
machine learning: A review. IEEE Sensors Journal,
23(4):3395–3407.
Wu, J., Ping, L., Ge, X., Wang, Y., and Fu, J. (2010). Cloud
storage as the infrastructure of cloud computing. In
2010 International Conference on Intelligent Comput-
ing and Cognitive Informatics, pages 380–383.
Zhu, D., Zhang, Y., Gao, Q., Lu, Y., and Sun, D. (2023).
Infrared and visible image fusion using threshold seg-
mentation and weight optimization. IEEE Sensors
Journal, 23(20):24970–24982.
IoTBDS 2025 - 10th International Conference on Internet of Things, Big Data and Security
230