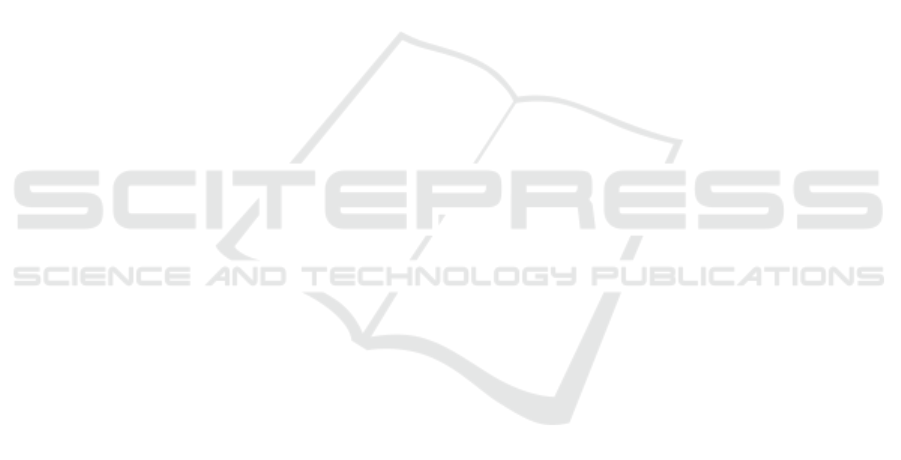
cated that the most impactful variables for prediction
were those derived from the ECG, accelerometer, and
EDA sensors.
8 CONCLUSIONS
This study confirms the feasibility of developing an
effective stress prediction model using information
collected from wearable devices. The findings under-
score the importance of leveraging diverse datasets
to enhance predictive accuracy, as demonstrated by
the Convolutional Neural Network achieving an accu-
racy of 99.8% for a binary classification that identifies
relaxed-stressed situations using two subject depen-
dent models. By building AI models upon WESAD
dataset we learned that ECG and EDA signals provide
the most valuable information to predict stress. The
results obtained in this research work will be used in
an observational study that will build a new dataset to
predict the stress suffered by Vicomtech profession-
als.
The potential applications of these models extend
to real-world settings, where early stress detection
can lead to timely interventions and improved mental
health outcomes. Future research could focus on op-
timizing personalized models for individual subjects
and exploring the integration of additional physiolog-
ical data from commercial wearables to advance early
stress detection.
ACKNOWLEDGMENT
We would like to acknowledge QOLIFE - Multimodal
real world capture and processing for quality of life
assessment project, funded as internal project of Fun-
daci
´
on Vicomtech.
REFERENCES
Affanni, A. (2020). Wireless sensors system for stress de-
tection by means of ecg and eda acquisition. Sensors,
20(7):2026.
American Psychological Association (2017). Stress in
america: The state of our nation. Technical report,
American Psychological Association.
Baig, M. M., Afifi, S., GholamHosseini, H., and Mirza, F.
(2019). A systematic review of wearable sensors and
iot-based monitoring applications for older adults–a
focus on ageing population and independent living.
Journal of medical systems, 43:1–11.
Can, Y. S., Chalabianloo, N., Ekiz, D., Fernandez-Alvarez,
J., Riva, G., and Ersoy, C. (2020). Personal stress-
level clustering and decision-level smoothing to en-
hance the performance of ambulatory stress detection
with smartwatches. IEEE Access, 8:38146–38163.
Dalmeida, K. M. and Masala, G. L. (2021). Hrv features as
viable physiological markers for stress detection using
wearable devices. Sensors, 21(8):2873.
Depression, W. (2017). Other common mental disorders:
global health estimates. Geneva: World Health Orga-
nization, 24(1).
Dinh, T., Nguyen, T., Phan, H.-P., Nguyen, N.-T., Dao,
D. V., and Bell, J. (2020). Stretchable respiration
sensors: Advanced designs and multifunctional plat-
forms for wearable physiological monitoring. Biosen-
sors and Bioelectronics, 166:112460.
Eren, E. and Navruz, T. S. (2022). Stress detection with
deep learning using bvp and eda signals. In 2022
International Congress on Human-Computer Interac-
tion, Optimization and Robotic Applications (HORA),
pages 1–7. IEEE.
Espeleta, H. C., Brett, E. I., Ridings, L. E., Leavens, E. L.,
and Mullins, L. L. (2018). Childhood adversity and
adult health-risk behaviors: Examining the roles of
emotion dysregulation and urgency. Child Abuse &
Neglect, 82:92–101.
Gallup (2017). State of the american workplace: Employee
engagement insights for u.s. business leaders. Techni-
cal report, Gallup.
Giorgi, G., Lecca, L. I., Alessio, F., Finstad, G. L., Bon-
danini, G., Lulli, L. G., Arcangeli, G., and Mucci,
N. (2020). Covid-19-related mental health effects
in the workplace: a narrative review. International
journal of environmental research and public health,
17(21):7857.
Kivim
¨
aki, M. and Steptoe, A. (2018). Effects of stress
on the development and progression of cardiovascular
disease. Nature Reviews Cardiology, 15(4):215–229.
Lupton, D. (2020). Wearable devices: Sociotechnical imag-
inaries and agential capacities.
Moise, N., Wainberg, M., and Shah, R. N. (2021). Primary
care and mental health: Where do we go from here?
World Journal of Psychiatry, 11(7):271–276.
Patel, V., Saxena, S., Lund, C., Thornicroft, G., Baingana,
F., Bolton, P., Chisholm, D., Collins, P. Y., Cooper,
J. L., Eaton, J., et al. (2018). The lancet commission
on global mental health and sustainable development.
The lancet, 392(10157):1553–1598.
Slavich, G. M. (2020). Social safety theory: a biologically
based evolutionary perspective on life stress, health,
and behavior. Annual review of clinical psychology,
16(1):265–295.
World Health Organization (2018). Mental health: strength-
ening our response. Technical report, World Health
Organization.
ICT4AWE 2025 - 11th International Conference on Information and Communication Technologies for Ageing Well and e-Health
196