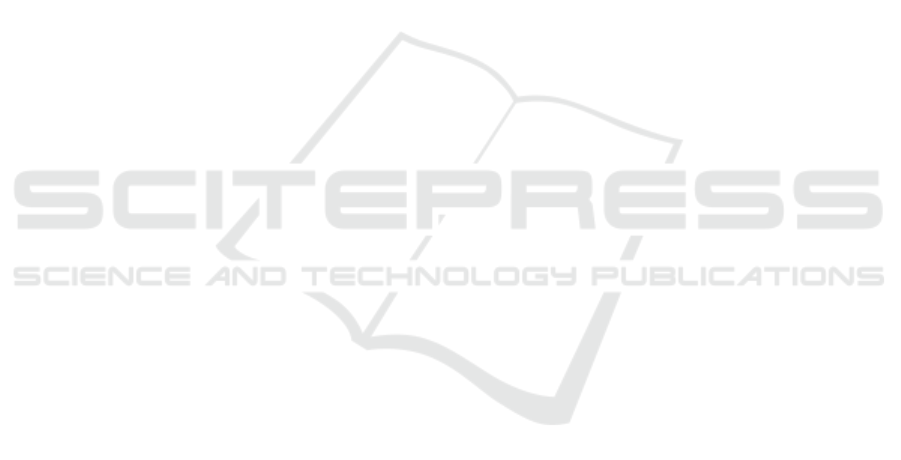
for distributed model selection and training. arXiv
preprint arXiv:1807.05118.
Liessner., R., Schmitt., J., Dietermann., A., and B
¨
aker., B.
(2019). Hyperparameter optimization for deep re-
inforcement learning in vehicle energy management.
In Proceedings of the 11th International Conference
on Agents and Artificial Intelligence - Volume 2:
ICAART, pages 134–144. INSTICC, SciTePress.
Marquard, U. and G
¨
otz, C. (2008). SAP Standard Appli-
cation Benchmarks - IT Benchmarks with a Business
Focus. In Kounev, S., Gorton, I., and Sachs, K., ed-
itors, Performance Evaluation: Metrics, Models and
Benchmarks, pages 4–8, Berlin, Heidelberg. Springer.
Mazyavkina, N., Sviridov, S., Ivanov, S., and Burnaev, E.
(2021). Reinforcement learning for combinatorial op-
timization: A survey. Computers & Operations Re-
search, 134:105400.
Mell, P. and Grance, T. (2011). The NIST Definition of
Cloud Computing. Technical Report NIST Special
Publication (SP) 800-145, National Institute of Stan-
dards and Technology.
Mennes, R., Spinnewyn, B., Latre, S., and Botero, J. F.
(2016). GRECO: A Distributed Genetic Algorithm
for Reliable Application Placement in Hybrid Clouds.
Proceedings - 2016 5th IEEE International Confer-
ence on Cloud Networking, CloudNet 2016.
Mnih, V., Badia, A. P., Mirza, M., Graves, A., Lillicrap,
T. P., Harley, T., Silver, D., and Kavukcuoglu, K.
(2016). Asynchronous Methods for Deep Reinforce-
ment Learning.
Mnih, V., Kavukcuoglu, K., Silver, D., Rusu, A. A., Ve-
ness, J., Bellemare, M. G., Graves, A., Riedmiller,
M., Fidjeland, A. K., Ostrovski, G., Petersen, S.,
Beattie, C., Sadik, A., Antonoglou, I., King, H., Ku-
maran, D., Wierstra, D., Legg, S., and Hassabis, D.
(2015). Human-level control through deep reinforce-
ment learning. Nature, 518(7540):529–533.
M
¨
uller, H., Kharitonov, A., Nahhas, A., Bosse, S., and Tur-
owski, K. (2021). Addressing IT Capacity Manage-
ment Concerns Using Machine Learning Techniques.
SN Computer Science, 3(1):26.
Papadimitriou, C. H. and Tsitsiklis, J. N. (1987). The Com-
plexity of Markov Decision Processes. Mathematics
of Operations Research, 12(3):441–450.
Raffin, A., Hill, A., Gleave, A., Kanervisto, A., Ernestus,
M., and Dormann, N. (2021). Stable-Baselines3: Reli-
able Reinforcement Learning Implementations. Jour-
nal of Machine Learning Research, 22(268):1–8.
Sahu, P., Roy, S., and Gharote, M. (2024). CloudAdvisor
for Sustainable and Data Residency Compliant Data
Placement in Multi-Cloud. In 2024 16th International
Conference on COMmunication Systems & NETworkS
(COMSNETS), pages 285–287.
Sahu, P., Roy, S., Gharote, M., Mondal, S., and Lodha, S.
(2022). Cloud Storage and Processing Service Selec-
tion considering Tiered Pricing and Data Regulations.
In 2022 IEEE/ACM 15th International Conference on
Utility and Cloud Computing (UCC), pages 92–101.
Schulman, J., Wolski, F., Dhariwal, P., Radford, A., and
Klimov, O. (2017). Proximal Policy Optimization Al-
gorithms.
Sfondrini, N., Motta, G., and Longo, A. (2018). Public
Cloud Adoption in Multinational Companies: A Sur-
vey. In 2018 IEEE International Conference on Ser-
vices Computing (SCC), pages 177–184.
Shi, T., Ma, H., Chen, G., and Hartmann, S. (2020).
Location-Aware and Budget-Constrained Service De-
ployment for Composite Applications in Multi-Cloud
Environment. IEEE Transactions on Parallel and Dis-
tributed Systems, 31(8):1954–1969.
Sutton, R. S., McAllester, D., Singh, S., and Mansour,
Y. (1999). Policy Gradient Methods for Reinforce-
ment Learning with Function Approximation. In
Advances in Neural Information Processing Systems,
volume 12. MIT Press.
Tavakoli, A., Pardo, F., and Kormushev, P. (2018). Action
branching architectures for deep reinforcement learn-
ing. Proceedings of the AAAI Conference on Artificial
Intelligence, 32(1).
Towers, M., Kwiatkowski, A., Terry, J., Balis, J. U.,
De Cola, G., Deleu, T., Goul
˜
ao, M., Kallinteris, A.,
Krimmel, M., KG, A., Perez-Vicente, R., Pierr
´
e, A.,
Schulhoff, S., Tai, J. J., Tan, H., and Younis, O. G.
(2024). Gymnasium: A Standard Interface for Rein-
forcement Learning Environments.
Triantaphyllou, E. (2000). Multi-Criteria Decision Mak-
ing Methods. In Triantaphyllou, E., editor, Multi-
Criteria Decision Making Methods: A Comparative
Study, pages 5–21. Springer US, Boston, MA.
Venkatraman, A. and Arend, C. (2022). A Resilient, Ef-
ficient, and Adaptive Hybrid Cloud Fit for a Dy-
namic Digital Business. Technical Report IDC
#EUR149741222, International Data Corporation.
Wang, X., Wang, S., Liang, X., Zhao, D., Huang, J., Xu, X.,
Dai, B., and Miao, Q. (2024). Deep Reinforcement
Learning: A Survey. IEEE Transactions on Neural
Networks and Learning Systems, 35(4):5064–5078.
Watkins, C. J. C. H. and Dayan, P. (1992). Q-learning. Ma-
chine Learning, 8(3):279–292.
Weinman, J. (2016). Hybrid cloud economics. IEEE Cloud
Computing, 3(1):18–22.
Ying, H., Song, M., Tang, Y., Xiao, S., and Xiao, Z.
(2024). Enhancing deep neural network training ef-
ficiency and performance through linear prediction.
Scientific Reports, 14(1):15197.
Zhang, B., Rajan, R., Pineda, L., Lambert, N., Biedenkapp,
A., Chua, K., Hutter, F., and Calandra, R. (2021).
On the importance of hyperparameter optimization
for model-based reinforcement learning. In Baner-
jee, A. and Fukumizu, K., editors, Proceedings of
The 24th International Conference on Artificial Intel-
ligence and Statistics, volume 130 of Proceedings of
Machine Learning Research. PMLR.
Zhu, J., Wu, F., and Zhao, J. (2022). An Overview of
the Action Space for Deep Reinforcement Learning.
In Proceedings of the 2021 4th International Confer-
ence on Algorithms, Computing and Artificial Intelli-
gence, ACAI ’21, New York, NY, USA. Association
for Computing Machinery.
Deep Reinforcement Learning for Selecting the Optimal Hybrid Cloud Placement Combination of Standard Enterprise IT Applications
443