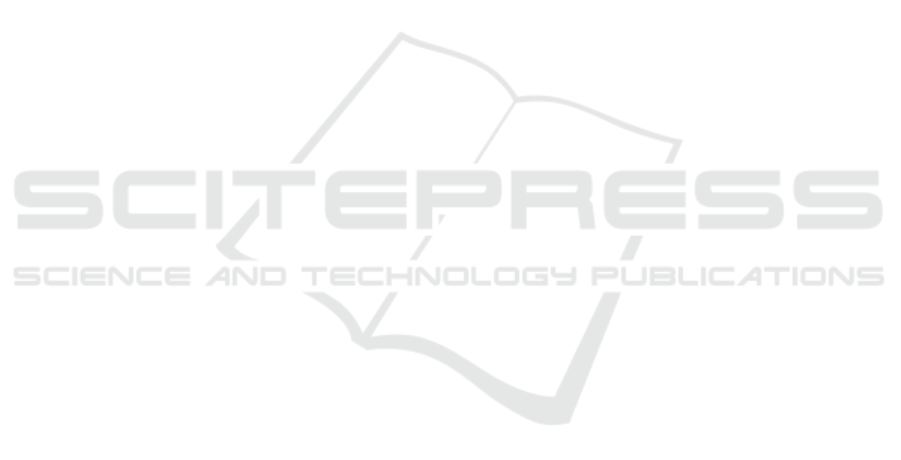
Conference on Advances in Computing, Communica-
tions and Informatics (ICACCI), pages 2659–2664.
Moser, R., Pedrycz, W., and Succi, G. (2008). A compar-
ative analysis of the efficiency of change metrics and
static code attributes for defect prediction. In Proceed-
ings of the 30th International Conference on Software
Engineering, ICSE ’08, page 181–190, New York,
NY, USA. Association for Computing Machinery.
Najmani, K., Benlahmar, E., Sael, N., and Zellou, A.
(2022). Collaborative filtering approach: A review
of recent research. In Kacprzyk, J., Balas, V., and
Ezziyyani, M., editors, Advanced Intelligent Systems
for Sustainable Development (AI2SD’2020), pages
151–163, Cham. Springer International Publishing.
Nassif, A., Talib, M. A., Azzeh, M., Alzaabi, S., Khanfar,
R., Kharsa, R., and Angelis, L. (2023). Software de-
fect prediction using learning to rank approach. Sci-
entific Reports, 13.
Ouedrhiri, O., Banouar, O., Hadaj, S. E., and Raghay, S.
(2022). Intelligent recommender system based on
quantum clustering and matrix completion. Concur-
rency and Computation: Practice and Experience,
34(15):e6943.
Pushpendu, K., Monideepa, R., and Sujoy, D. (2024).
Collaborative Filtering and Content-Based Systems,
pages 19–30. Springer Nature Singapore, Singapore.
Rathore, S. and Kumar, S. (2017). A decision tree logic
based recommendation system to select software fault
prediction techniques. Computing, 99.
Rokach, L. (2005). Ensemble Methods for Classifiers,
pages 957–980. Springer US, Boston, MA.
Salih, H., Basna, M., and Abdulazeez, A. (2021). A review
of principal component analysis algorithm for dimen-
sionality reduction. Journal of Soft Computing and
Data Mining, 2(1):20–30.
Salman, M., Nag, A., Pathan, M., and Dev, S. (2022). An-
alyzing the impact of feature selection on the accu-
racy of heart disease prediction. Healthcare Analytics,
2:100060.
Shakeela, S., Shankar, N. S., Reddy, P. M., Tulasi, T. K.,
and Koneru, M. M. (2021). Optimal ensemble learn-
ing based on distinctive feature selection by univari-
ate anova-f statistics for ids. International Journal
of Electronics and Telecommunications, vol. 67(No
2):267–275.
Sheugh, L. and Alizadeh, S. (2015). A note on pearson
correlation coefficient as a metric of similarity in rec-
ommender system. In 2015 AI and Robotics (IRA-
NOPEN), pages 1–6.
Singh, R. H., Maurya, S., Tripathi, T., Narula, T., and Sri-
vastav, G. (2020). Movie recommendation system us-
ing cosine similarity and knn. International Journal
of Engineering and Advanced Technology, 9(5):556–
559.
Tahir, H., Khan, S., S.Ali, and Syed, S. (2021). Lcbpa: An
enhanced deep neural network-oriented bug prioriti-
zation and assignment technique using content-based
filtering. IEEE Access, 9:92798–92814.
Tapucu, D., Kasap, S., and Tekbacak, F. (2012). Perfor-
mance comparison of combined collaborative filter-
ing algorithms for recommender systems. In 2012
IEEE 36th Annual Computer Software and Applica-
tions Conference Workshops, pages 284–289.
Toure, F., Mourad, B., and Lamontagne, L. (2017). Inves-
tigating the prioritization of unit testing effort using
software metrics. In Proceedings of the 12th Interna-
tional Conference on Evaluation of Novel Approaches
to Software Engineering - ENASE, pages 69–80. IN-
STICC, SciTePress.
Vujovic, Z. D. (2021). Classification model evaluation met-
rics. International Journal of Advanced Computer
Science and Applications, 12(6).
Wu, D., Shang, M., Luo, X., and Wang, Z. (2022). An l1-
and-l2-norm-oriented latent factor model for recom-
mender systems. IEEE Transactions on Neural Net-
works and Learning Systems, 33(10):5775–5788.
Wu, X. (2024). Review of collaborative filtering recom-
mendation systems. Applied and Computational En-
gineering, 43:76–82.
Zaid, M. (2015). Correlation and Regression Analysis.
SESRI Publications. Comprehensive overview of
Pearson and Spearman correlation types, assumptions,
and applications.
Zamani, M., Beigy, H., and Shaban, A. (2014). Cascading
randomized weighted majority: A new online ensem-
ble learning algorithm. ArXiv, abs/1403.0388.
Zegzouti, S. E. F., Banouar, O., and Benslimane, M. (2023).
Collaborative filtering approach: A review of recent
research. In Cerchiello, P., Osmetti, A. A. S., and
Spelta, A., editors, Proceedings of the Statistics and
Data Science Conference (SDS’2023), pages 164–
169, Cham. Springer International Publishing.
Zhongbin, S., Junqi, L., Heli, S., and Liang, H. (2021).
Cfps: Collaborative filtering based source projects se-
lection for cross-project defect prediction. Applied
Soft Computing, 99:106940.
ENASE 2025 - 20th International Conference on Evaluation of Novel Approaches to Software Engineering
424