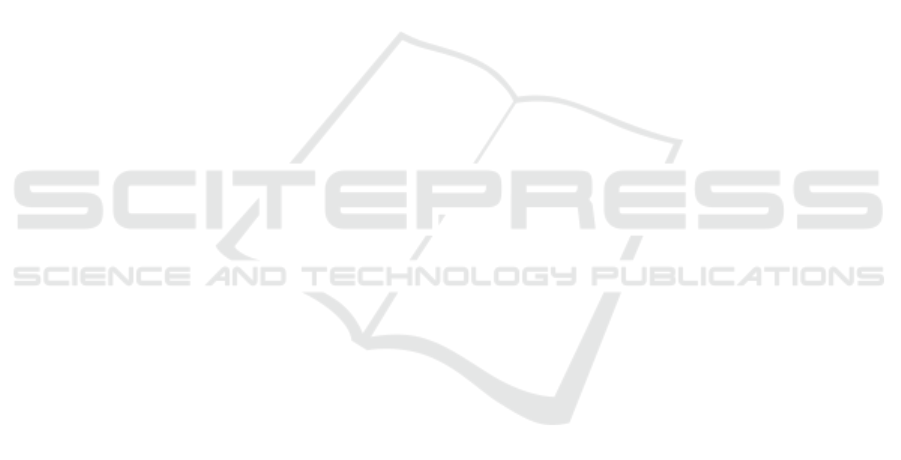
Gehrmann, S., et al. (2023). Palm: Scaling language
modeling with pathways. Journal of Machine Learn-
ing Research, 24(240):1–113.
Es, S., James, J., Espinosa-Anke, L., and Schockaert, S.
(2023). Ragas: Automated evaluation of retrieval aug-
mented generation. arXiv preprint arXiv:2309.15217.
Freire, M., Serrano-Laguna,
´
A., Manero Iglesias, B.,
Mart
´
ınez-Ortiz, I., Moreno-Ger, P., and Fern
´
andez-
Manj
´
on, B. (2023). Game learning analytics: Learn-
ing analytics for serious games. In Learning, design,
and technology: An international compendium of the-
ory, research, practice, and policy, pages 3475–3502.
Springer.
Gallotta, R., Todd, G., Zammit, M., Earle, S., Liapis, A.,
Togelius, J., and Yannakakis, G. N. (2024). Large
language models and games: A survey and roadmap.
arXiv preprint arXiv:2402.18659.
Gao, Y., Xiong, Y., Gao, X., Jia, K., Pan, J., Bi, Y., Dai, Y.,
Sun, J., and Wang, H. (2023). Retrieval-augmented
generation for large language models: A survey. arXiv
preprint arXiv:2312.10997.
Gee, J. P. (2003). What video games have to teach us
about learning and literacy. Computers in entertain-
ment (CIE), 1(1):20–20.
Geertz, C. (2008). Thick description: Toward an inter-
pretive theory of culture. In The cultural geography
reader, pages 41–51. Routledge.
Hann, C. and James, D. (2024). One Hundred Years of Arg-
onauts: Malinowski, Ethnography and Economic An-
thropology, volume 13. Berghahn Books.
Hoffmann, M. P., Fillies, J., and Paschke, A. (2024). Ma-
linowski in the age of ai: Can large language models
create a text game based on an anthropological clas-
sic? arXiv preprint arXiv:2410.20536.
Hua, M. and Raley, R. (2020). Playing with unicorns: Ai
dungeon and citizen nlp. DHQ: Digital Humanities
Quarterly, 14(4).
Huesmann, L. R. (2010). Nailing the coffin shut on doubts
that violent video games stimulate aggression: com-
ment on anderson et al.(2010).
J
¨
arvelin, K. and Kek
¨
al
¨
ainen, J. (2002). Cumulated gain-
based evaluation of ir techniques. ACM Transactions
on Information Systems (TOIS), 20(4):422–446.
Leach, J. W. and Leach, E. (1983). The Kula: new perspec-
tives on Massim exchange. CUP Archive.
Lewis, P., Perez, E., Piktus, A., Petroni, F., Karpukhin,
V., Goyal, N., K
¨
uttler, H., Lewis, M., Yih, W.-t.,
Rockt
¨
aschel, T., et al. (2020). Retrieval-augmented
generation for knowledge-intensive nlp tasks. Ad-
vances in Neural Information Processing Systems,
33:9459–9474.
Lin, X. V., Chen, X., Chen, M., Shi, W., Lomeli, M., James,
R., Rodriguez, P., Kahn, J., Szilvasy, G., Lewis, M.,
et al. (2023). Ra-dit: Retrieval-augmented dual in-
struction tuning. arXiv preprint arXiv:2310.01352.
Liu, J. (2023). Building production-ready rag applications.
Malinowski, B. (2013). Argonauts of the western Pa-
cific: An account of native enterprise and adven-
ture in the archipelagoes of Melanesian New Guinea
[1922/1994]. Routledge.
Manathunga, S. S. and Illangasekara, Y. (2023). Retrieval
augmented generation and representative vector sum-
marization for large unstructured textual data in med-
ical education. arXiv preprint arXiv:2308.00479.
Manuaba, I. B. K. (2017). Text-based games as potential
media for improving reading behaviour in indonesia.
Procedia computer science, 116:214–221.
Marcus, G. (2020). The next decade in ai: four steps
towards robust artificial intelligence. arXiv preprint
arXiv:2002.06177.
Pell, B. (1992). Metagame in symmetric chess-like games.
Petroni, F., Rockt
¨
aschel, T., Lewis, P., Bakhtin, A., Wu,
Y., Miller, A. H., and Riedel, S. (2019). Lan-
guage models as knowledge bases? arXiv preprint
arXiv:1909.01066.
Pia, A. (2019). On digital ethnographies. anthropology, pol-
itics and pedagogy (part i).
Reed, A. (2023). 50 Years of Text Games: From Oregon
Trail to AI Dungeon. Changeful Tales.
Rizki, I. A., Suprapto, N., Saphira, H. V., Alfarizy, Y., Ra-
madani, R., Saputri, A. D., and Suryani, D. (2024).
Cooperative model, digital game, and augmented
reality-based learning to enhance students’ critical
thinking skills and learning motivation. Journal of
Pedagogical Research, 8(1):339–355.
Saad-Falcon, J., Khattab, O., Potts, C., and Zaharia, M.
(2023). Ares: An automated evaluation framework
for retrieval-augmented generation systems. arXiv
preprint arXiv:2311.09476.
Todd, G., Padula, A., Stephenson, M., Piette,
´
E., Soe-
mers, D. J., and Togelius, J. (2024). Gavel: Generat-
ing games via evolution and language models. arXiv
preprint arXiv:2407.09388.
Touvron, H., Martin, L., Stone, K., Albert, P., Almahairi,
A., Babaei, Y., Bashlykov, N., Batra, S., Bhargava,
P., Bhosale, S., et al. (2023). Llama 2: Open foun-
dation and fine-tuned chat models. arXiv preprint
arXiv:2307.09288.
van Horssen, J., Moreton, Z., and Pelurson, G. (2023). From
pixels to pedagogy: using video games for higher ed-
ucation in the humanities. Journal of Learning Devel-
opment in Higher Education, (28).
Vaswani, A. (2017). Attention is all you need. Advances in
Neural Information Processing Systems.
Wang, A. I. and Tahir, R. (2020). The effect of using ka-
hoot! for learning–a literature review. Computers &
Education, 149:103818.
Yasunaga, M., Aghajanyan, A., Shi, W., James, R.,
Leskovec, J., Liang, P., Lewis, M., Zettlemoyer,
L., and Yih, W.-t. (2022). Retrieval-augmented
multimodal language modeling. arXiv preprint
arXiv:2211.12561.
Zhang, T., Kishore, V., Wu, F., Weinberger, K. Q., and
Artzi, Y. (2019). Bertscore: Evaluating text genera-
tion with bert. arXiv preprint arXiv:1904.09675.
From Text to Text Game: A Novel RAG Approach to Gamifying Anthropological Literature and Build Thick Games
255