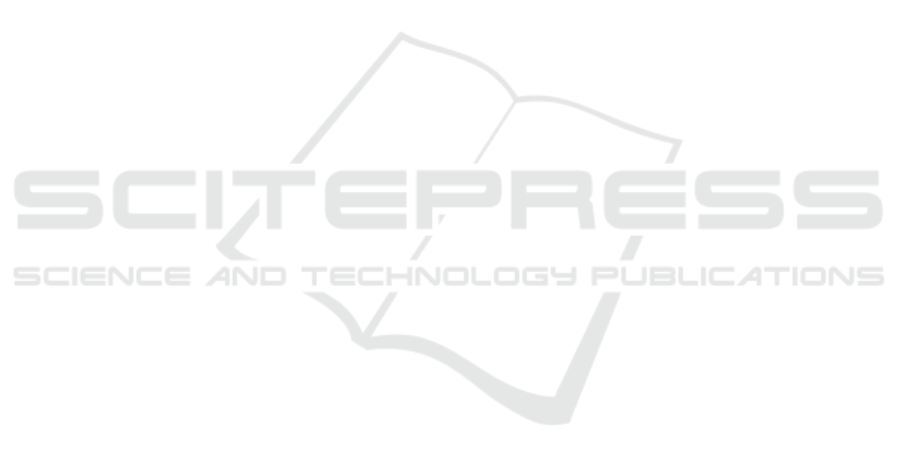
model. The Annals of Applied Statistics, 9(3):1350–
1371.
Martin, F., Chen, Y., Moore, R. L., and Westine, C. D.
(2020). Systematic review of adaptive learning re-
search designs, context, strategies, and technologies
from 2009 to 2018. Educational Technology Research
and Development, 68(4):1903–1929.
Menze, D., Seidel, N., and Kasakowskij, R. (2022). Interac-
tion of reading and assessment behavior. In Henning,
P. A., Striewe, M., and W
¨
olfel, M., editors, DELFI
2022 – Die 21. Fachtagung Bildungstechnologien der
Gesellschaft f
¨
ur Informatik e.V., pages 27–38, Bonn.
Gesellschaft f
¨
ur Informatik.
Molenaar, I., de Mooij, S., Azevedo, R., Bannert, M.,
J
¨
arvel
¨
a, S., and Ga
ˇ
sevi
´
c, D. (2023). Measuring self-
regulated learning and the role of AI: Five years of
research using multimodal multichannel data. Com-
puters in Human Behavior, 139:107540.
Nakic, J., Granic, A., and Glavinic, V. (2015). Anatomy
of Student Models in Adaptive Learning Systems:
A Systematic Literature Review of Individual Differ-
ences from 2001 to 2013. Journal of Educational
Computing Research, 51(4):459–489.
Nielsen, L. (2014). Personas. In Soegaard, M. and Dam,
R. F., editors, The Encyclopedia of Human-Computer
Interaction. Interaction Design Foundation.
Normadhi, N. B., Shuib, L., Md Nasir, H. N., Bimba, A.,
Idris, N., and Balakrishnan, V. (2019). Identification
of personal traits in adaptive learning environment:
Systematic literature review. Computers & Education,
130:168–190.
Nussbaumer, A., Dahn, I., Kroop, S., Mikroyannidis, A.,
and Albert, D. (2015). Supporting Self-Regulated
Learning, pages 17–48. Springer International Pub-
lishing, Cham.
Nussbaumer, A., Kravcik, M., Renzel, D., Klamma, R.,
Berthold, M., and Albert, D. (2014). A Framework
for Facilitating Self-Regulation in Responsive Open
Learning Environments.
Perez Alvarez, R., Jivet, I., Perez-sanagustin, M., Scheffel,
M., and Verbert, K. (2022). Tools Designed to Support
Self-Regulated Learning in Online Learning Environ-
ments: A Systematic Review. IEEE Transactions on
Learning Technologies, 15(4):508–522.
Prinsloo, P. and Slade, S. (2017). Ethics and Learning
Analytics: Charting the (Un)Charted. In Lang, C.,
Siemens, G., Wise, A., and Ga
ˇ
sevi
´
c, D., editors,
Handbook of Learning Analytics, pages 49–57. SO-
LAR.
Radovi
´
c, S., Seidel, N., Menze, D., and Kasakowskij, R.
(2024). Investigating the effects of different levels of
students’ regulation support on learning process and
outcome: In search of the optimal level of support
for self-regulated learning. Computers & Education,
215(105041).
Renzel, D., Klamma, R., Kravcik, M., and Nussbaumer, A.
(2015). Tracing self-regulated learning in responsive
open learning environments. In Li, F. W., Klamma,
R., Laanpere, M., Zhang, J., Manj
´
on, B. F., and Lau,
R. W., editors, Advances in Web-Based Learning –
ICWL 2015, pages 155–164, Cham. Springer Interna-
tional Publishing.
Rose, S., Engel, D., Cramer, N., and Cowley, W. (2010).
Automatic Keyword Extraction from Individual Docu-
ments, pages 1–20.
Seidel, N., Karolyi, H., Burchart, M., and de Witt, C.
(2021). Approaching Adaptive Support for Self-
regulated Learning. In Guralnick, D., Auer, M. E., and
Poce, A., editors, Innovations in Learning and Tech-
nology for the Workplace and Higher Education. TLIC
2021. Lecture Notes in Networks and Systems, pages
409–424, Cham. Springer International Publishing.
Sghir, N., Adadi, A., and Lahmer, M. (2023). Recent ad-
vances in Predictive Learning Analytics: A decade
systematic review (2012–2022). Education and In-
formation Technologies, 28(7):8299–8333.
Shi, S., Xie, Y., Wang, Z., Ding, B., Li, Y., and Zhang, M.
(2022). Explainable Neural Rule Learning. In Pro-
ceedings of the ACM Web Conference 2022, WWW
’22, pages 3031–3041, New York, NY, USA. Associ-
ation for Computing Machinery.
Tabebordbar, A. and Beheshti, A. (2018). Adaptive Rule
Monitoring System. In 2018 IEEE/ACM 1st Interna-
tional Workshop on Software Engineering for Cogni-
tive Services (SE4COG). Proceedings, pages 45–51.
Tsai, Y.-S., Moreno-Marcos, P. M., Jivet, I., Scheffel, M.,
Tammets, K., Kollom, K., and Ga
ˇ
sevi
´
c, D. (2018).
The sheila framework: Informing institutional strate-
gies and policy processes of learning analytics. Jour-
nal of Learning Analytics, 5(3):5–20.
Vandewaetere, M., Desmet, P., and Clarebout, G. (2011).
The contribution of learner characteristics in devel-
opment of computer-based adaptive learning environ-
ments. Computers in Human Behaviors, 27:118–130.
White, J., Fu, Q., Hays, S., Sandborn, M., Olea, C., Gilbert,
H., Elnashar, A., Spencer-Smith, J., and Schmidt,
D. C. (2023). A prompt pattern catalog to enhance
prompt engineering with chatgpt. arXiv:2302.11382.
Wisniewski, B., Zierer, K., and Hattie, J. (2020). The Power
of Feedback Revisited: A Meta-Analysis of Educa-
tional Feedback Research. Frontiers in Psychology,
10.
Wu, T. T., Lee, H. Y., Li, P. H., Huang, C. N., and Huang,
Y. M. (2024). Promoting Self-Regulation Progress
and Knowledge Construction in Blended Learning via
ChatGPT-Based Learning Aid. Journal of Educa-
tional Computing Research, 61(8):3–31.
Xie, H., Chu, H.-C., Hwang, G.-J., and Wang, C.-C.
(2019). Trends and development in technology-
enhanced adaptive/personalized learning: A system-
atic review of journal publications from 2007 to 2017.
Computers & Education, 140:103599.
Yau, J. (2009). A MOBILE CONTEXT-AWARE FRAME-
WORK FOR SUPPORTING SELF-REGULATED
LEARNERS. In International Conference on Cog-
nition and Exploratory Learning in Digital Age
(CELDA, pages 415–419. IADIS.
Yau, J. Y.-K. and Joy, M. (2008). A Self-Regulated Learn-
ing Approach: A Mobile Context-aware and Adap-
tive Learning Schedule (mCALS) Tool. International
Journal of Interactive Mobile Technologies (iJIM), 2.
CSEDU 2025 - 17th International Conference on Computer Supported Education
254