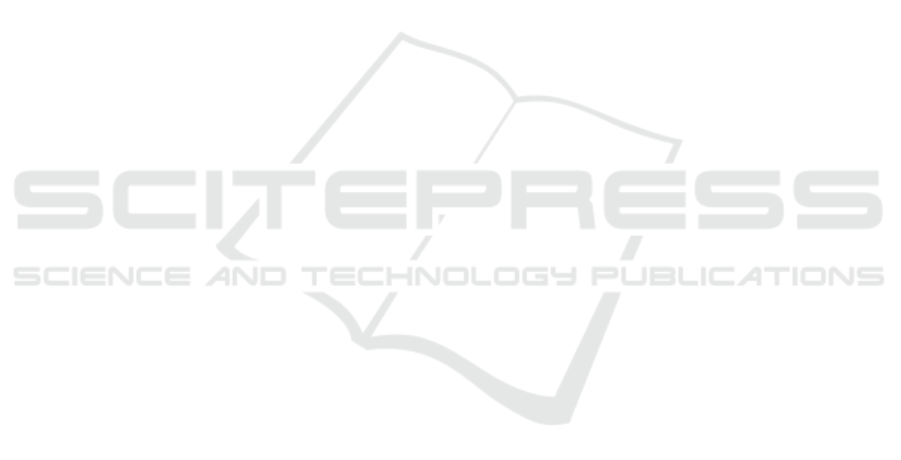
de Santana, V. F. and Silva, F. E. F. (2019). An Easy to Use
Data Logger for Local User Studies. In Proceedings of
the 16th International Web for All Conference, W4A
’19, New York, NY, USA. Association for Computing
Machinery.
Fullerton, T. (2008). Game design workshop: a playcentric
approach to creating innovative games. CRC press.
Gajos, K. Z. and Chauncey, K. (2017). The influence of per-
sonality traits and cognitive load on the use of adaptive
user interfaces. In Proceedings of the 22Nd Interna-
tional Conference on Intelligent User Interfaces, IUI
’17, pages 301–306, New York, NY, USA. ACM.
Gajos, K. Z., Weld, D. S., and Wobbrock, J. O. (2010).
Automatically generating personalized user interfaces
with Supple. Artificial Intelligence.
Galindo, J. A., Dupuy-Chessa, S., and C
´
eret, E. (2018). To-
ward a generic architecture for ui adaptation to emo-
tions. In Proceedings of the 29th Conference on
L’Interaction Homme-Machine, IHM ’17, pages 263–
272, New York, NY, USA. ACM.
Henze, N., Rukzio, E., and Boll, S. (2011). 100,000,000
taps: analysis and improvement of touch performance
in the large. In Proceedings of the 13th Interna-
tional Conference on Human Computer Interaction
with Mobile Devices and Services, MobileHCI ’11,
page 133–142, New York, NY, USA. Association for
Computing Machinery.
Inkpen, K., Dearman, D., Argue, R., Comeau, M., Fu, C.-
L., Kolli, S., Moses, J., Pilon, N., and Wallace, J.
(2006). Left-handed scrolling for pen-based devices.
International Journal of Human–Computer Interac-
tion, 21(1):91–108.
Kane, S. K., Wobbrock, J. O., Harniss, M., and Johnson,
K. L. (2008). TrueKeys: Identifying and Correcting
Typing Errors for People with Motor Impairments. In
Proceedings of the 13th International Conference on
Intelligent User Interfaces, IUI ’08, pages 349–352,
New York, NY, USA. ACM.
Kurschl, W., Augstein, M., and Stitz, H. (2012). Adap-
tive User Interfaces on Tablets to Support People with
Disabilities. In Mensch & Computer 2012 – Work-
shopband - 12. fach
¨
ubergreifende Konferenz f
¨
ur inter-
aktive und kooperative Medien.
Lee, H. and Choi, Y. S. (2011). Fit your hand: Personal-
ized user interface considering physical attributes of
mobile device users. In Proceedings of the 24th An-
nual ACM Symposium Adjunct on User Interface Soft-
ware and Technology, UIST ’11 Adjunct, pages 59–
60, New York, NY, USA. ACM.
Martin, F., Chen, Y., Moore, R. L., and Westine, C. D.
(2020). Systematic review of adaptive learning re-
search designs, context, strategies, and technologies
from 2009 to 2018. Educational Technology Research
and Development, 68(4):1903–1929.
Moon, J., Kum, S., and Lee, S. (2017). Introduction to
the design of personalized user interface platform with
recommended contents. In Proceedings of the 3rd In-
ternational Conference on Communication and Infor-
mation Processing, ICCIP ’17, pages 104–107, New
York, NY, USA. ACM.
Moured, O., Baumgarten-Egemole, M., M
¨
uller, K., Roit-
berg, A., Schwarz, T., and Stiefelhagen, R. (2024).
Chart4blind: An intelligent interface for chart accessi-
bility conversion. In Proceedings of the 29th Interna-
tional Conference on Intelligent User Interfaces, IUI
’24, page 504–514, New York, NY, USA. Association
for Computing Machinery.
Naughton, J. E. (2017). Nothing About Us Without Us.
Technical report, EDF European Disability Forum.
Parhi, P., Karlson, A. K., and Bederson, B. B. (2006). Target
size study for one-handed thumb use on small touch-
screen devices.
Parker, R. I., Vannest, K. J., and Davis, J. L. (2011). Effect
size in single-case research: a review of nine nonover-
lap techniques. Behavior modification, 35(4):303–
322.
Paymans, T. F., Lindenberg, J., and Neerincx, M. (2004).
Usability trade-offs for adaptive user interfaces: Ease
of use and learnability. In Proceedings of the 9th In-
ternational Conference on Intelligent User Interfaces,
IUI ’04, pages 301–303, New York, NY, USA. ACM.
Perry, K. B. and Hourcade, J. P. (2008). Evaluating one
handed thumb tapping on mobile touchscreen devices.
In Proceedings of Graphics Interface 2008.
Riddell, S. and Weedon, E. (2014). Disabled students in
higher education: Discourses of disability and the ne-
gotiation of identity. International Journal of Educa-
tional Research, 63:38–46. Special Issues: Represent-
ing Diversity in Education: Student identities in con-
texts of learning and instruction & Classroom-Based
Talk.
Schmidtmann, H. and Preusse, J. (2015). Soziode-
mografie, studienmotive und studienerfolg beruflich
qualifizierter studierender: Befunde an der fern-
universit
¨
at in hagen. Beruflich Qualifizierte im
Studium, 101.
Silfverberg, M., MacKenzie, I. S., and Korhonen, P. (2000).
Predicting Text Entry Speed on Mobile Phones. In
Proceedings of the SIGCHI Conference on Human
Factors in Computing Systems, CHI ’00, pages 9–16,
New York, NY, USA. ACM.
The National Eye Institute (NEI) (2015). Facts About Color
Blindness.
W3C (2011). Accessible Rich Internet Applications (WAI-
ARIA) 1.0 W3C Candidate Recommendation 18 Jan-
uary 2011.
Wang, W., Khalajzadeh, H., Grundy, J., Madugalla, A., and
Obie, H. O. (2024). Adaptive user interfaces for soft-
ware supporting chronic disease. In Proceedings of
the 46th International Conference on Software En-
gineering: Software Engineering in Society, ICSE-
SEIS’24, page 118–129, New York, NY, USA. Asso-
ciation for Computing Machinery.
Wobbrock, J. O., Gajos, K. Z., Kane, S. K., and Vanderhei-
den, G. C. (2018). Ability-based design. Commun.
ACM, 61(6):62–71.
Wobbrock, J. O., Kane, S. K., Gajos, K. Z., Harada, S.,
and Froehlich, J. (2011). Ability-Based Design. ACM
Transactions on Accessible Computing.
Game-Based Ergonomic Adaptation of User Interfaces for Online Learning Environments
265