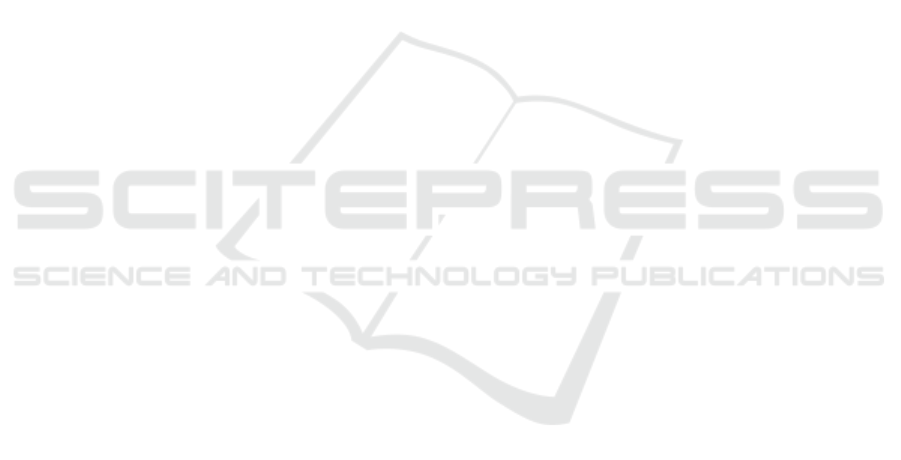
models predict multiple depths of comprehension dur-
ing and one week following reading. Intl. EDM Soci-
ety.
Christhilf, K., Newton, N., Butterfuss, R., McCarthy, K. S.,
Allen, L. K., Magliano, J. P., and McNamara, D. S.
(2022). Using markov models and random walks to
examine strategy use of more or less successful com-
prehenders. International Educational Data Mining
Society.
Crossley, S. A., Skalicky, S., Dascalu, M., McNamara,
D. S., and Kyle, K. (2017). Predicting text compre-
hension, processing, and familiarity in adult readers:
New approaches to readability formulas. Discourse
Processes, 54:340–359.
Cutting, L. E. and Scarborough, H. S. (2006). Predic-
tion of reading comprehension: Relative contributions
of word recognition, language proficiency, and other
cognitive skills can depend on how comprehension is
measured. Scientific Studies of Reading, 10:277–299.
Daniel, D. B. and Woody, W. D. (2013). E-textbooks at
what cost? Performance and use of electronic v. print
texts. Computers and Education, 62:18–23.
Davis, F. B. (1944). Fundamental factors of comprehension
in reading. Psychometrika, 9:185–197.
Fletcher, J. M. (2006). Measuring reading comprehension.
Scientific Studies of Reading, 10:323–330.
Foroughi, C. K., Werner, N. E., Barrag
´
an, D., and Boehm-
Davis, D. A. (2015). Interruptions disrupt reading
comprehension. Journal of Experimental Psychology,
144.
Gooding, S., Berzak, Y., Mak, T., and Sharifi, M. (2021).
Predicting text readability from scrolling interactions.
Association for Computational Linguistics.
Gough, P. B. and Tunmer, W. E. (1986). Decoding, reading,
and reading disability. Remedial and Special Educa-
tion, 7:6–10.
Guerra, J., Parra, D., and Brusilovsky, P. (2013). Encour-
aging online student reading with social visualization
support. Proceedings of the Workshops at the 16th
International Conference on Artificial Intelligence in
Education AIED, pages 47–50.
Hellings, J. and Haelermans, C. (2022). The effect of pro-
viding learning analytics on student behaviour and
performance in programming: a randomised con-
trolled experiment. Higher Education, 83:1–18.
Hoppe, H. U., Doberstein, D., and Hecking, T. (2021).
Using sequence analysis to determine the well-
functioning of small groups in large online courses.
International Journal of Artificial Intelligence in Edu-
cation, 31:680–699.
Kim, M. K., Gaul, C. J., Bundrage, C. N., and Madathany,
R. J. (2020). Technology supported reading com-
prehension: a design research of the student mental
model analyzer for research and teaching (smart) tech-
nology. Interact. Learn. Environ.
Landrum, R. E., Gurung, R. A., and Spann, N. (2012).
Assessments of textbook usage and the relationship
to student course performance. College Teaching,
60:17–24.
Milliner, B. and Cote, T. (2015). One year of extensive read-
ing on mobile devices: engagement and impressions.
pages 404–409. Research-publishing.net.
Panel, N. R. (2000). Teaching children to read: An
evidence-based assessment of the scientific research
literature on reading and its implications for reading
instruction. NIH Publication No. 00-4769, 7.
Peckham, T. and McCalla, G. (2012). Mining student be-
havior patterns in reading comprehension tasks.
Perfetti, C. A., Landi, N., and Oakhill, J. (2005). The acqui-
sition of reading comprehension skill. pages 227–247.
Peverly, S. T. and Wood, R. (2001). The effects of ad-
junct questions and feedback on improving the read-
ing comprehension skills of learning-disabled adoles-
cents. Contemporary Educational Psychology, 26:25–
43.
Seidel, N., Stritzinger, A., Menze, D., and Friedrich, K.
(2024). Longpage - A Moodle activity plugin de-
signed to assist learners with reading extended texts.
Slade, S. and Prinsloo, P. (2013). Learning analytics: Ethi-
cal issues and dilemmas. American Behavioral Scien-
tist, 57:1510–1529.
Sun, J. C. Y., Lin, C. T., and Chou, C. (2018). Applying
learning analytics to explore the effects of motivation
on online students’ reading behavioral patterns. In-
ternational Review of Research in Open and Distance
Learning, 19:209–227.
Sun, Y., Harper, D. J., and Watt, S. N. K. (2004). De-
sign of an e-book user interface and visualizations to
support reading for comprehension. pages 510–511.
ACM Press.
Suresh, K. (2011). An overview of randomization tech-
niques: An unbiased assessment of outcome in clin-
ical research. Journal of Human Reproductive Sci-
ences, 4:8.
Syed, R., Collins-Thompson, K., Bennett, P. N., Teng, M.,
Williams, S., Tay, D. W. W., and Iqbal, S. (2020). Im-
proving learning outcomes with gaze tracking and au-
tomatic question generation. pages 1693–1703. ACM.
Thaker, K. M., Zhang, L., He, D., and Brusilovsky, P.
(2020). Recommending remedial readings using stu-
dent knowledge state. International Conference on
Education Data Mining.
Wang, S. and Walker, E. (2021). Providing adaptive feed-
back in concept mapping to improve reading compre-
hension. Conference on Human Factors in Computing
Systems - Proceedings.
Yang, A. C., Chen, I. Y., Flanagan, B., and Ogata, H. (2021).
Automatic generation of cloze items for repeated test-
ing to improve reading comprehension. Educational
Technology and Society, 24:147–158.
Dynamic Reading Comprehension Visualization in Digital Course Texts
273