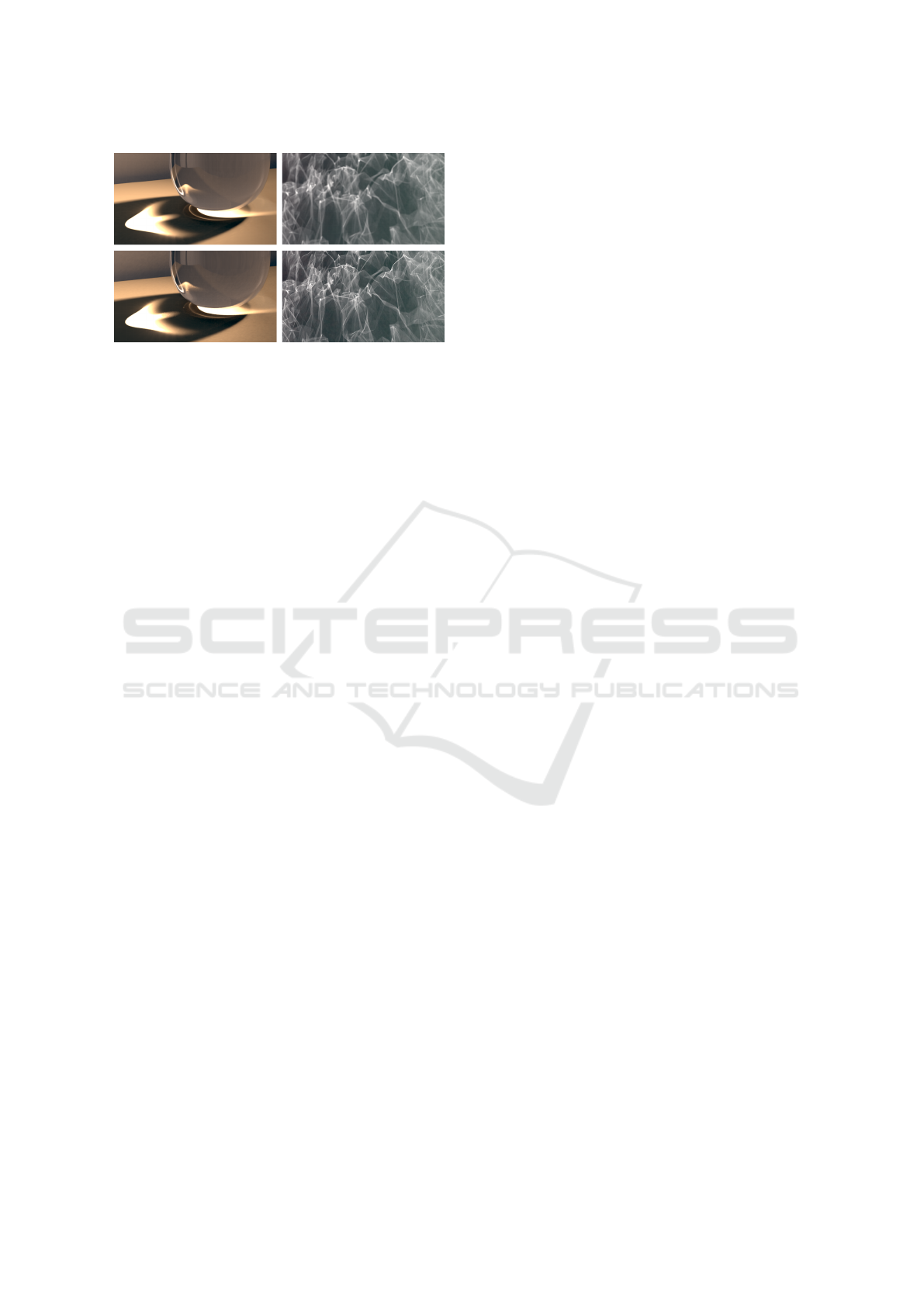
Figure 6: Qualitative comparison of caustics under E-
1000K (top-left: RTPM, 100,000 iterations; bottom-left:
ARP∆, 10,000 iterations; right: RTPM and ARP∆, 500 iter-
ations).
of shadows, as the smoothing parameters remained
unchanged.
Photon differentials, in contrast to progressive
techniques, enable the rendering of sharp and well-
defined caustics, as demonstrated in Figure 6 and Ta-
ble 2. Progressive methods often result in blurry caus-
tics before reaching convergence, noticeable in the
Water-Caustics and Caustic-Glass scenes (see Figure
6, right). While reducing the initial radius can mit-
igate this blurriness, it also increases variance. By
integrating progressive photon mapping with photon
differentials, we effectively address the challenge of
extremely anisotropic photons by defaulting to pro-
gressive photons when such cases are detected. We
posit that large photon footprints and their corre-
sponding large bounding volumes hinder balanced
BVH construction. Preliminary tests indicate that us-
ing the culling heuristic boosts computational effi-
ciency.
7 CONCLUSION & FUTURE
WORK
This paper presents a new approach to global illu-
mination using photon differentials optimised for ray
tracing hardware. When facing problematic ellipsoid
footprints, we switch to progressive photon mapping
to ensure the accuracy of our solution. The results
demonstrate high-quality physically based rendering
at real-time rates. Future research should focus on
automating the smoothing parameters based on pho-
ton density, illumination type, photon path and scene
geometry.
REFERENCES
Bitterli, B. (2016). Rendering resources. https://benedikt-
bitterli.me/resources/.
Evangelou, I., Papaioannou, G., Vardis, K., and Vasilakis,
A. A. (2021). Fast radius search exploiting ray trac-
ing frameworks. Journal of Computer Graphics Tech-
niques (JCGT), 10(1):25–48.
Fabianowski, B. (2011). Interactive Manycore Photon
Mapping. PhD thesis, Trinity College (Dublin, Ire-
land). School of Computer Science & Statistics.
Fabianowski, B. and Dingliana, J. (2009). Interactive
global photon mapping. Computer Graphics Forum,
28(4):1151–1159.
Frisvad, J. R., Schjøth, L., Erleben, K., and Sporring, J.
(2014). Photon differential splatting for rendering
caustics. Computer Graphics Forum, 33(6):252–263.
Hachisuka, T., Ogaki, S., and Jensen, H. W. (2008). Pro-
gressive photon mapping. ACM Trans. Graph., 27(5).
Igehy, H. (1999). Tracing ray differentials. In Proceedings
of the 26th Annual Conference on Computer Graph-
ics and Interactive Techniques, SIGGRAPH ’99, page
179–186, USA. ACM Press/Addison-Wesley Publish-
ing Co.
Jensen, H. W. (1996). Global illumination using photon
maps. In Pueyo, X. and Schr
¨
oder, P., editors, Ren-
dering Techniques ’96, pages 21–30, Vienna. Springer
Vienna.
Jensen, H. W. (2004). A practical guide to global illu-
mination using ray tracing and photon mapping. In
ACM SIGGRAPH 2004 Course Notes, SIGGRAPH
’04, page 20–es, New York, NY, USA. Association
for Computing Machinery.
Kallweit, S., Clarberg, P., Kolb, C., Davidovi
ˇ
c, T., Yao,
K.-H., Foley, T., He, Y., Wu, L., Chen, L., Akenine-
M
¨
oller, T., Wyman, C., Crassin, C., and Benty, N.
(2022). The Falcor rendering framework. https:
//github.com/NVIDIAGameWorks/Falcor.
Kaplanyan, A. S. and Dachsbacher, C. (2013). Adaptive
progressive photon mapping. ACM Trans. Graph.,
32(2).
Kern, R., Br
¨
ull, F., and Grosch, T. (2023). Accelerated pho-
ton mapping for hardware-based ray tracing. Journal
of Computer Graphics Techniques (JCGT), 12(1):1–
28.
Kim, H. (2019). Caustics Using Screen-Space Photon Map-
ping, pages 543–555. Apress, Berkeley, CA.
Knaus, C. and Zwicker, M. (2011). Progressive photon
mapping: A probabilistic approach. ACM Trans.
Graph., 30(3).
Mara, M., Luebke, D., and McGuire, M. (2013). Toward
practical real-time : Efficient gpu density estimation.
In Proceedings of the ACM SIGGRAPH Symposium
on Interactive 3D Graphics and Games, I3D ’13, page
71–78, New York, NY, USA. Association for Comput-
ing Machinery.
McGuire, M. (2017). Computer graphics archive. https:
//casual-effects.com/data.
McGuire, M. and Luebke, D. (2009). Hardware-accelerated
global illumination by image space photon mapping.
GRAPP 2025 - 20th International Conference on Computer Graphics Theory and Applications
306