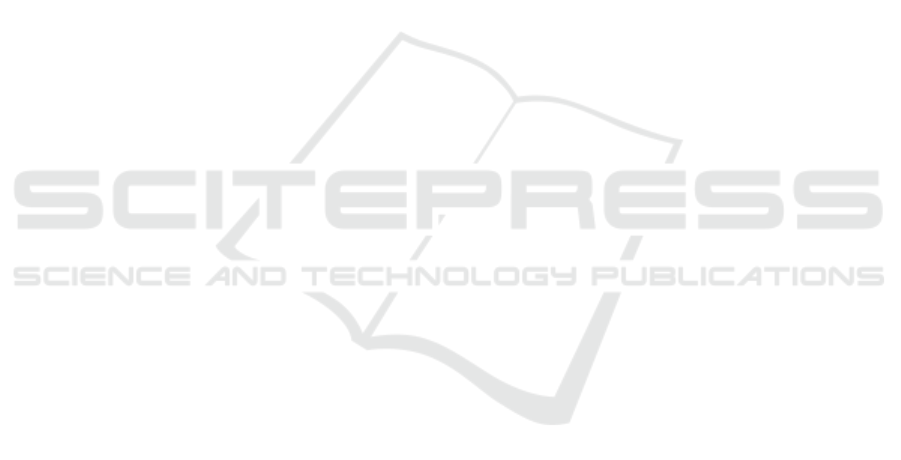
strates limited scalability). ArangoDB performed the
worst, likely due to its multi-model approach. Cas-
sandra only counts friends, while MongoDB uses an
array with exact friend IDs, affecting performance.
Neighborhood Search (C5). Unexpectedly, no
system performed a query on more than 4k dataset.
On smaller datasets, the best performers were SQLite
and Neo4j. ArangoDB and MySQL performed worse.
MongoDB was not fully comparable due to incom-
plete result sets. Surprisingly, SQLite handled the
graph traversal well using recursive common table ex-
pressions but may struggle with larger joins. Neo4j
outperformed ArangoDB, making it the better graph
database for this query, but more research on its per-
formance is needed.
Shortest Path (C6). ArangoDB and Neo4j ex-
celled with optimized methods for finding the short-
est path. SQLite and MySQL performed poorly, espe-
cially at higher depths, struggling with graph traver-
sals. MongoDB’s breadth-first search approach and
Cassandra’s lack of support for graph traversals made
them unsuitable for this comparison. Neo4j and
ArangoDB are the most effective for querying inter-
connected data, particularly in complex graph queries
like the shortest path.
Optional Traversal (C7). Cassandra and Mon-
goDB excelled, handling higher data volumes effec-
tively, while MySQL and Neo4j performed the worst.
ArangoDB outperformed Neo4j by a noticeable mar-
gin, suggesting it’s better suited for querying poten-
tially non-existent relationships. SQLite maintained
consistent performance across all data volumes. This
query highlights how systems differ in modelling and
processing data; Cassandra optimizes performance
by directly storing a friendCount property, reduc-
ing the need for additional tables, while SQLite and
ArangoDB may be more suitable for handling com-
plex or aggregate operations.
Union (D1). Cassandra and SQLite performed
best, with SQLite handling the normalized schema
surprisingly well. Neo4j struggled, experiencing
timeouts during testing. MySQL’s performance was
unexpectedly poor compared to SQLite, possibly
due to SQLite’s superior query optimizer for union
queries. ArangoDB’s performance was similar to
MySQL. MongoDB handled the query well, even
with an inter-collection join, though more research
is needed to assess the scalability of its $unionWith
operation. Depending on the frequency, SQLite is
ideal for infrequent unions, Cassandra for frequent,
and MongoDB for flexibility.
Intersection (D2). Cassandra and MongoDB per-
formed best, particularly with lower data volumes.
Neo4j struggled with datasets of 4k or more, likely
due to the apoc.coll.intersection bottleneck.
SQLite again outperformed MySQL in this set opera-
tion. ArangoDB’s performance was better than Neo4j
but lagged behind relational databases. Cassandra and
MongoDB excel at real-time data processing for inter-
section queries, while SQLite offers an alternative for
minimizing server strain in such operations.
Difference (D3). Cassandra, MongoDB, and
SQLite performed best, while ArangoDB and
MySQL lagged. MySQL’s performance decreased
notably with the 1024k dataset, though the difference
was not drastic. For finding differences, Cassandra,
MongoDB, and SQLite are strong choices, with Cas-
sandra and MongoDB leveraging arrays or attributes
to reduce join operations, though this requires careful
handling of synchronization and denormalization.
Non-Indexed Columns Sorting (E1). All sys-
tems, except Cassandra, performed well in sorting by
non-indexed columns. Cassandra does not support
sorting by non-clustering columns.
Indexed Columns Sorting (E2). All systems per-
formed well in sorting by indexed columns, with Cas-
sandra showing a slight but almost unnoticeable per-
formance advantage.
Distinct (E3). Cassandra performed well in find-
ing unique combinations of product brands and ven-
dor countries. ArangoDB was the worst performer.
Higher data volumes made it challenging to iden-
tify the slowest system, as reducing Vendor-Products
relationships caused execution time drops across all
systems. Cassandra is particularly efficient, auto-
matically upserting duplicates using PRIMARY KEY.
SQLite may be a good choice for this query pattern
based on its strong performance in the experiments.
MapReduce (F1). Cassandra and MongoDB are
the top choices for very large datasets, thanks to their
scalability and support for horizontal operations.
While Cassandra performed well with smaller data
volumes, the results were inconclusive overall. This
query on small volumes resembles aggregation, with
similar execution times, but Cassandra’s lack of a
join operation and reliance on denormalized data
could limit its performance at larger scales. MySQL’s
performance notably degraded in larger datasets.
To sum up, our analysis shows that MySQL, SQLite,
and ArangoDB have the most expressive query lan-
guages, but Cassandra and MongoDB excel in per-
formance and scalability with large datasets. Neo4j
and ArangoDB are ideal for traversing interconnected
data, with ArangoDB offering more versatility in
data models and outperforming Neo4j in many cases,
though further research is needed to explore this fully.
Cassandra and MongoDB are the top performers
SQL vs NoSQL: Six Systems Compared
51