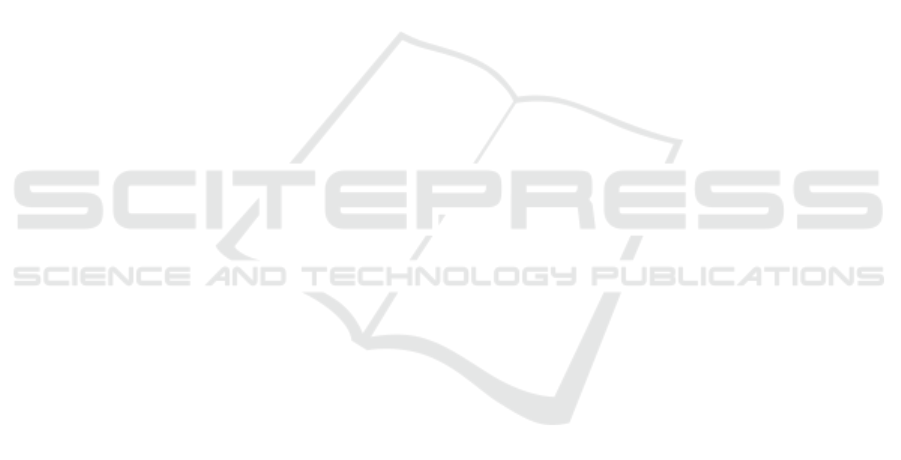
REFERENCES
Abreu, J. M., Pereira, F. C., and Ferr
˜
ao, P. (2012). Using
pattern recognition to identify habitual behavior in resi-
dential electricity consumption. Energy and Buildings,
49:479–487.
Alahakoon, D. and Yu, X. (2016). Smart electricity meter
data intelligence for future energy systems: A survey.
IEEE Transactions on Industrial Informatics, 12:425–
436.
Armel, K. C., Gupta, A., Shrimali, G., and Albert, A. (2013).
Is disaggregation the holy grail of energy efficiency?
the case of electricity. Energy Policy, 52:213–234.
Asghar, M. R., D
´
an, G., Miorandi, D., and Chlamtac, I.
(2017). Smart meter data privacy: A survey. IEEE
Communications Surveys Tutorials, 19:2820–2835.
Beckel, C., Kleiminger, W., Cicchetti, R., Staake, T., and
Santini, S. (2014). The eco data set and the perfor-
mance of non-intrusive load monitoring algorithms. In
Proceedings of the 1st ACM International Conference
on Embedded Systems for Energy-Efficient Buildings,
BuildSys ’14, page 80–89, New York, NY, USA. Asso-
ciation for Computing Machinery.
Beckel, C., Sadamori, L., and Santini, S. (2012). Towards
automatic classification of private households using
electricity consumption data. In Proceedings of the
Fourth ACM Workshop on Embedded Sensing Systems
for Energy-Efficiency in Buildings, BuildSys ’12, page
169–176, New York, NY, USA. Association for Com-
puting Machinery.
Beckel, C., Sadamori, L., and Santini, S. (2013). Automatic
socio-economic classification of households using elec-
tricity consumption data. In Proceedings of the 4th
ACM International Conference on Future Energy Sys-
tems (e-Energy 2013), pages 75–86, New York, NY,
USA. ACM.
Birt, B. J., Newsham, G. R., Beausoleil-Morrison, I., Arm-
strong, M. M., Saldanha, N., and Rowlands, I. H.
(2012). Disaggregating categories of electrical energy
end-use from whole-house hourly data. Energy and
Buildings, 50:93–102.
Bishop, C. M. (2006). Pattern Recognition and Ma-
chine Learning (Information Science and Statistics).
Springer-Verlag, Berlin, Heidelberg.
Burkhart, S., Unterweger, A., Eibl, G., and Engel, D. (2018).
Detecting swimming pools in 15-minute load data. In
17th IEEE International Conference On Trust, Secu-
rity And Privacy In Computing And Communications
(TrustCom 2018), pages 1651–1655, New York, NY,
USA. IEEE.
Chen, D., Barker, S., Subbaswamy, A., Irwin, D., and
Shenoy, P. (2013). Non-intrusive occupancy monitor-
ing using smart meters. In Proceedings of the 5th ACM
Workshop on Embedded Systems For Energy-Efficient
Buildings - BuildSys’13, pages 1–8, New York, NY,
USA. Association for Computing Machinery.
Chen, T. and Guestrin, C. (2016). Xgboost: A scalable tree
boosting system. In Proceedings of the 22nd ACM
SIGKDD International Conference on Knowledge Dis-
covery and Data Mining, KDD ’16, page 785–794,
New York, NY, USA. Association for Computing Ma-
chinery.
Chicco, D. and Jurman, G. (2020). The advantages of the
matthews correlation coefficient (mcc) over f1 score
and accuracy in binary classification evaluation. BMC
genomics, 21:1–13.
Chicco, D., Starovoitov, V. V., and Jurman, G. (2021). The
benefits of the matthews correlation coefficient (mcc)
over the diagnostic odds ratio (dor) in binary classifica-
tion assessment. IEEE Access, 9:47112–47124.
Chicco, G. (2016). Customer behaviour and data analytics.
In 2016 International Conference and Exposition on
Electrical and Power Engineering (EPE), pages 771–
779, Iasi, Romania. IEEE.
Commission, E. (2012). 2012/148/eu: Commission recom-
mendation of 9 march 2012 on preparations for the
roll-out of smart metering systems.
Darby, S. (2010). Smart metering: What potential for house-
holder engagement? Building Research and Informa-
tion, 38:442–457.
Efthymiou, C. and Kalogridis, G. (2010). Smart grid pri-
vacy via anonymization of smart metering data. In
2010 First IEEE International Conference on Smart
Grid Communications, pages 238–243, Gaithersburg,
Maryland, USA. IEEE.
Eibl, G. and Engel, D. (2015). Influence of data granularity
on smart meter privacy. IEEE Transactions on Smart
Grid, 6:930–939.
Engel, D. and Eibl, G. (2017). Wavelet-based multiresolution
smart meter privacy. IEEE Transactions on Smart Grid,
8:1710–1721.
Erkin, Z., Troncoso-Pastoriza, J. R., Lagendijk, R. L., and
Perez-Gonzalez, F. (2013). Privacy-preserving data
aggregation in smart metering systems: An overview.
IEEE Signal Processing Magazine, 30:75–86.
Fan, Z., Kulkarni, P., Gormus, S., Efthymiou, C., Kalogridis,
G., Sooriyabandara, M., Zhu, Z., Lambotharan, S.,
and Chin, W. H. (2013). Smart grid communications:
Overview of research challenges, solutions, and stan-
dardization activities. IEEE Communications Surveys
and Tutorials, 15:21–38.
Ferner, C., Eibl, G., Unterweger, A., Burkhart, S., and We-
genkittl, S. (2019). Pool detection from smart metering
data with convolutional neural networks. Energy Infor-
matics, 2:1–9.
Finster, S. and Baumgart, I. (2014). Privacy-aware smart
metering: A survey. IEEE Communications Surveys &
Tutorials, 16:1732–1745.
Granell, R., Axon, C. J., and Wallom, D. C. (2015). Impacts
of raw data temporal resolution using selected clus-
tering methods on residential electricity load profiles.
IEEE Transactions on Power Systems, 30(6):3217–
3224.
Greveler, U., Justus, B., and L
¨
ohr, D. (2012). Multime-
dia content identification through smart meter power
usage profiles. In Proceedings of the International Con-
ference on Information and Knowledge Engineering
(IKE), pages 1–8, Athens, GA, USA. CSREA Press.
Hart, G. (1992). Nonintrusive appliance load monitoring.
Proceedings of the IEEE, 80(12):1870–1891.
Predicting Socio-Demographic Characteristics from Load Profiles with Varying Time Granularities
97