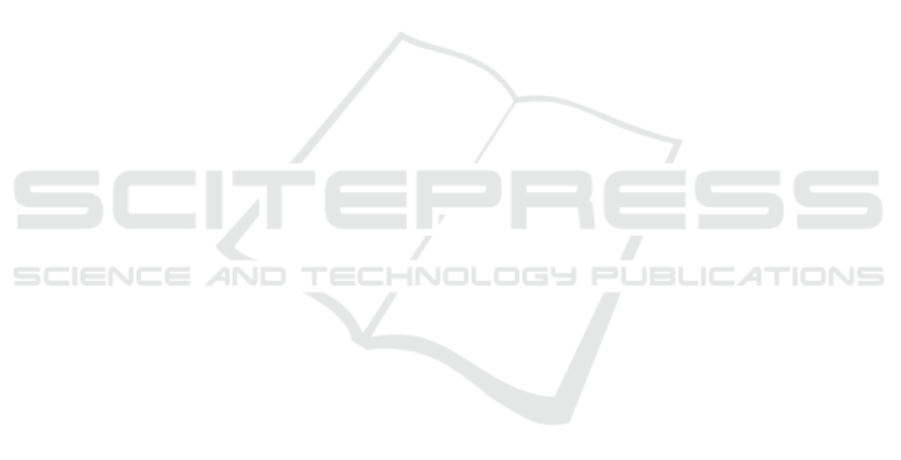
Almeida, H., and Perkusich, A. (2019). An effort es-
timation support tool for agile software development:
An empirical evaluation. In Proceedings of the In-
ternational Conference on Software Engineering and
Knowledge Engineering, SEKE, volume 2019-July,
pages 82–87. Knowledge Systems Institute Graduate
School.
Dantas, E., Perkusich, M., Dilorenzo, E., Santos, D. F.,
Almeida, H., and Perkusich, A. (2018). Effort esti-
mation in agile software development: An updated re-
view. In International Journal of Software Engineer-
ing and Knowledge Engineering, volume 28, pages
1811–1831. World Scientific Publishing Co. Pte Ltd.
de A. Cabral, J. T. H., de A. Araujo, R., Nobrega, J. P.,
and de Oliveira, A. L. (2017). Heterogeneous ensem-
ble dynamic selection for software development effort
estimation. In 2017 IEEE 29th International Con-
ference on Tools with Artificial Intelligence (ICTAI),
pages 210–217.
Dehghan, A., Blincoe, K., and Damian, D. (2016). A hy-
brid model for task completion effort estimation. In
SWAN 2016 - Proceedings of the 2nd International
Workshop on Software Analytics, co-located with FSE
2016, pages 22–28. Association for Computing Ma-
chinery, Inc.
Dragicevic, S., Celar, S., and Turic, M. (2017). Bayesian
network model for task effort estimation in agile soft-
ware development. Journal of Systems and Software,
127:109–119.
Goto, T. (2016). ACIT-CSII-BCD 2016 : 4th International
Conference on Applied Computing and Information
Technology (ACIT 2016) ; 3rd International Confer-
ence on Computational Science/Intelligence and Ap-
plied Informatics (CSII 2016), 1st International Con-
ference on Big Data, Cloud Computing, Data Science
& Engineering (BCD 2016) : proceedings : 12-14 De-
cember 2016, Las Vegas, Nevada, USA. Conference
Publishing Services, IEEE Computer Society.
Gultekin, M. and Kalipsiz, O. (2020). Story point-based
effort estimation model with machine learning tech-
niques. International Journal of Software Engineering
and Knowledge Engineering, 30:43–66.
Hoda, M. N. (2023). Proceedings of the 17th INDIACom;
2023 10th International Conference on Computing for
Sustainable Global Development (INDIACom) : (15-
17 March 2023). India-Com.
Hosni, M. (2023). On the value of combiners in hetero-
geneous ensemble effort estimation. In International
Joint Conference on Knowledge Discovery, Knowl-
edge Engineering and Knowledge Management, IC3K
- Proceedings, volume 1, pages 153–163. Science and
Technology Publications, Lda.
Idri, A., Hosni, M., and Abran, A. (2016). Improved esti-
mation of software development effort using classical
and fuzzy analogy ensembles. Applied Soft Comput-
ing Journal, 49:990–1019.
Iordan, A. E. (2024). An optimized lstm neural network
for accurate estimation of software development ef-
fort. Mathematics, 12.
Iqbal, M., Ijaz, M., Mazhar, T., Shahzad, T., Abbas, Q.,
Ghadi, Y. Y., Ahmad, W., and Hamam, H. (2024). Ex-
ploring issues of story-based effort estimation in ag-
ile software development (asd). Science of Computer
Programming, 236.
Iwata, K., Nakashima, T., Anan, Y., and Ishii, N. (2017).
Machine learning classification to effort estimation for
embedded software development projects. Interna-
tional Journal of Software Innovation, 5:19–32.
Karner, G. (1993). Metrics for object-oriented system de-
velopment. Technical report, Objectory Systems De-
veloper Report.
Kassaymeh, S., Alweshah, M., Al-Betar, M. A., Hammouri,
A. I., and Al-Ma’aitah, M. A. (2024). Software ef-
fort estimation modeling and fully connected artifi-
cial neural network optimization using soft computing
techniques. Cluster Computing, 27:737–760.
Kitchenham, B., Pearl Brereton, O., Budgen, D., Turner,
M., Bailey, J., and Linkman, S. (2009). Systematic lit-
erature reviews in software engineering – a systematic
literature review. Information and Software Technol-
ogy, 51(1):7–15. Special Section - Most Cited Articles
in 2002 and Regular Research Papers.
Kitchenham, B. A. (2004). Procedures for performing sys-
tematic reviews.
Kumar, A. (2024). Recommendation of machine learning
techniques for software effort estimation using multi-
criteria decision making. Journal of Universal Com-
puter Science, 30:221–241.
Kumar, K. H. and Srinivas, K. (2023). An accurate anal-
ogy based software effort estimation using hybrid op-
timization and machine learning techniques. Multime-
dia Tools and Applications, 82:30463–30490.
Kumar, S., Arora, M., Sakshi, and Chopra, S. (2022). A
review of effort estimation in agile software devel-
opment using machine learning techniques. In 4th
International Conference on Inventive Research in
Computing Applications, ICIRCA 2022 - Proceedings,
pages 416–422. Institute of Electrical and Electronics
Engineers Inc.
Lavingia, K., Patel, R., Patel, V., and Lavingia, A. (2024).
Software effort estimation using machine learning al-
gorithms. Scalable Computing, 25:1276–1285.
Marapelli, B., Carie, A., and Islam, S. M. (2021). Software
effort estimation with use case points using ensemble
machine learning models. In International Confer-
ence on Electrical, Computer, and Energy Technolo-
gies, ICECET 2021. Institute of Electrical and Elec-
tronics Engineers Inc.
Meiliana, Daniella, G., Wijaya, N., Putra, N. G. E., and
Efata, R. (2023). Agile software development effort
estimation based on product backlog items. In Proce-
dia Computer Science, volume 227, pages 186–193.
Elsevier B.V.
Najm, A. and Zakrani, A. (2023). On the interpretability of
a tree-based ensemble for predicting software effort.
In Colloquium in Information Science and Technol-
ogy, CIST, pages 129–134. Institute of Electrical and
Electronics Engineers Inc.
Nakamura, M. (2019). Proceedings, 18th IEEE/ACIS Inter-
national Conference on Software Engineering, Artifi-
cial Intelligence, Networking and Parallel/Distributed
ICEIS 2025 - 27th International Conference on Enterprise Information Systems
150