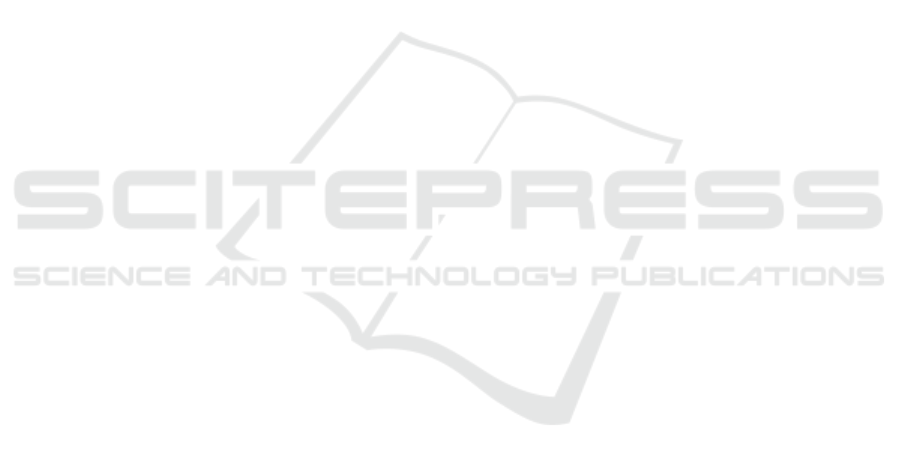
Iqbal, M., Ijaz, M., Mazhar, T., Shahzad, T., Abbas,
Q., Ghadi, Y., et al. (2024). Exploring issues of
story-based effort estimation in agile software devel-
opment (asd). Science of Computer Programming,
236:103114.
Javed, M. and Lin, Y. (2021). imer: Iterative process of
entity relationship and business process model extrac-
tion from the requirements. Information and Software
Technology, 135:106558.
Kitchenham, B. and Charters, S. (2007). Guidelines for per-
forming systematic literature reviews in software en-
gineering. Technical Report EBSE 2007-001, Keele
University and Durham University Joint Report.
Kolhatkar, G., Madan, A., Kowtal, N., Roy, S., and Son-
awane, S. (2023). Converting epics/stories into pseu-
docode using transformers. In 2023 IEEE 20th India
Council International Conference (INDICON), pages
37–42. IEEE.
Kustiawan, Y. A. and Lim, T. Y. (2023). User stories in re-
quirements elicitation: A systematic literature review.
In 2023 IEEE 8th International Conference On Soft-
ware Engineering and Computer Systems (ICSECS),
pages 211–216. IEEE.
Nistala, P. V., Rajbhoj, A., Kulkarni, V., Soni, S., Nori,
K. V., and Reddy, R. (2022). Towards digitalization
of requirements: generating context-sensitive user sto-
ries from diverse specifications. Automated Software
Engineering, 29(1):26.
Oran, A. C., Santos, G., Gadelha, B., and Conte, T.
(2021). A framework for evaluating and improving
requirements specifications based on the developers
and testers perspective. Requirements Engineering,
26(4):481–508.
¨
Ozcelikkan, N., Tuzkaya, G., Alabas-Uslu, C., and Sen-
naroglu, B. (2022). A multi-objective agile project
planning model and a comparative meta-heuristic
approach. Information and Software Technology,
151:107023.
Putri, R. A., Yuhana, U. L., and Amri, T. C. (2023). K-
means and feature selection mechanism to improve
performance of clustering user stories in agile devel-
opment. In 2023 International Conference on Mod-
eling & E-Information Research, Artificial Learning
and Digital Applications (ICMERALDA), pages 39–
43. IEEE.
Raharjana, I. K., Siahaan, D., and Fatichah, C. (2021). User
stories and natural language processing: A systematic
literature review. IEEE Access, 9:53811–53826.
Siahaan, D., Raharjana, I. K., and Fatichah, C. (2023).
User story extraction from natural language for re-
quirements elicitation: Identify software-related infor-
mation from online news. Information and Software
Technology, 158:107195.
Thanomwong, P. and Senivongse, T. (2022). User story risk
prioritization model for agile software development.
In 2022 International Conference on Data and Soft-
ware Engineering (ICoDSE), pages 161–166. IEEE.
Tsilionis, K., Maene, J., Heng, S., Wautelet, Y., and Poel-
mans, S. (2021a). Conceptual modeling versus user
story mapping: Which is the best approach to agile
requirements engineering? In International Confer-
ence on Research Challenges in Information Science,
pages 356–373. Springer International Publishing.
Tsilionis, K., Maene, J., Heng, S., Wautelet, Y., and Poel-
mans, S. (2021b). Evaluating the software problem
representation on the basis of rationale trees and user
story maps: premises of an experiment. In Soft-
ware Business: 11th International Conference, IC-
SOB 2020, Karlskrona, Sweden, November 16–18,
2020, Proceedings 11, pages 219–227. Springer In-
ternational Publishing.
Urbieta, M., Antonelli, L., Rossi, G., and do Prado Leite, J.
C. S. (2020). The impact of using a domain language
for an agile requirements management. Information
and Software Technology, 127:106375.
Vera-Rivera, F. H., Barbosa Mora, J. L., and Gaona, C.
(2020). Generaci
´
on autom
´
atica de la planificaci
´
on de
la entrega en desarrollo de software agil, asignaci
´
on
de historias de usuario a los desarrolladores usando
algoritmos gen
´
eticos. AiBi Revista de Investigaci
´
on,
Administraci
´
on e Ingenier
´
ıa, 8(2):29–38.
Villamizar, H., Kalinowski, M., Garcia, A., and Mendez,
D. (2020). An efficient approach for reviewing
security-related aspects in agile requirements specifi-
cations of web applications. Requirements Engineer-
ing, 25(4):439–468.
Wang, T., Wang, C., Li, T., Liu, Z., and Zhai, Y.
(2022). User story quality assessment based on multi-
dimensional perspective: A preliminary framework.
In iStar, pages 7–13.
Wu, C., Wang, C., Li, T., and Zhai, Y. (2022). A node-
merging based approach for generating istar mod-
els from user stories. In Software Engineering and
Knowledge Engineering, pages 257–262.
Xu, X., Dou, Y., Qian, L., Jiang, J., Yang, K., and
Tan, Y. (2023a). Quality improvement method for
high-end equipment’s functional requirements based
on user stories. Advanced Engineering Informatics,
56:102017.
Xu, X., Dou, Y., Qian, L., Zhang, Z., Ma, Y., and Tan, Y.
(2023b). A requirement quality assessment method
based on user stories. Electronics, 12(10):2155.
Yang, B., Guo, H., and Liu, H. (2023). Evaluation and as-
sessment of machine learning based user story group-
ing: A framework and empirical studies. Science of
Computer Programming, 227:102943.
Analyzing User Story Quality: A Systematic Review of Common Issues and Solutions
159