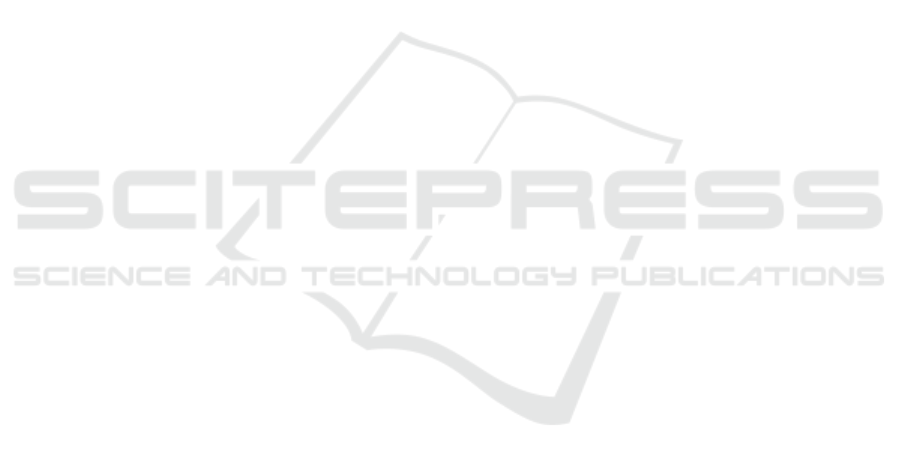
ness, creativity, social intelligence, and the capacity
to drive innovation, contributing to collective well-
being. The union between individuals and exponen-
tial technologies enables the development of expo-
nential schools and universities, forming a generation
of "5.0 Citizens," equipped with hard skills and soft
skills, ready to drive innovations with a positive im-
pact on society. In this sense, the culture of innova-
tion is nurtured from preschool education and extends
to Elementary School, High School, Technical Educa-
tion, and Higher Education, fostering lifelong innova-
tion skills and adaptation, which continuously foster
new businesses and solutions for environmental, so-
cial, and governance challenges.
However, Education 5.0 faces significant chal-
lenges, particularly in bridging the gap between
universities, notably in engineering and comput-
ing courses—responsible for hard skills develop-
ment—and public schools, which are run with dedica-
tion by pedagogues and educators. The Manna_Team
addresses this challenge directly by integrating prac-
tical kits of Artificial Intelligence, IoD, IoT, Robotics,
and Games into public schools, promoting soft
skills development through an innovative method-
ology known as MannaDrigar. This methodology
combines learning styles such as Accommodating,
Converging, Assimilating, and Diverging with socio-
emotional competencies (emotional awareness, emo-
tional regulation, relationship skills), fostering a per-
sonalized approach adapted to students’ demands
(Luckin et al., 2016) (Kolb and Kolb, 2005).
This paper aims to present the MannaDrigar
Methodology, developed as a strategy that combines
learning styles and socio-emotional competencies to
maximize student engagement and performance in in-
novation activities conducted in Manna_Team Boot-
camps. The methodology proposes analyzing partici-
pants’ learning styles and socio-emotional competen-
cies to organize teams with complementary profiles,
seeking greater engagement and synergy to foster bet-
ter performance in practical innovation activities.
As a case study, we applied the methodology in
Manna_Team Bootcamps, immersive experiences in
Exponential Technologies, with a special focus on
the Internet of Drones (IoD). During the bootcamps,
participants were divided into two groups: control
(without applying the methodology) and experimental
(with applying the methodology). These groups were
evaluated in three stages: diagnostic assessment at the
beginning of the bootcamp to measure learning styles
and socio-emotional skills; formative assessment dur-
ing the bootcamp to monitor progress in each session;
and summative assessment at the end, which mea-
sured the experimental groups’ performance through
innovation competitions. This study aims to demon-
strate the impact of personalization, based on learning
styles and socio-emotional skills, on developing tech-
nical and interpersonal skills.
Building the future involves broad access to Expo-
nential Technologies and knowledge dissemination,
making it accessible to everyone. When these tech-
nologies are used to develop soft skills and integrate
curricular content, they offer a unique opportunity for
educational innovation and disruption. This paper,
therefore, adopts the Internet of Drones as a central
technology in the bootcamp experiments, seeking to
demonstrate the potential of personalized methodolo-
gies for comprehensive student development.
2 LITERATURE REVIEW
This study builds upon two systematic literature
reviews offering a comprehensive perspective on
methodologies and technologies within the Education
5.0 framework. The first review, "Systematic Litera-
ture Review on Instruments and Strategies for Learn-
ing Assessment in the Context of Education 5.0,"
examined methodologies and tools designed to per-
sonalize learning and integrate technical and socio-
emotional skills. Using the Parsifal tool, the initial
review retrieved 241 articles, from which 30 were
selected based on rigorous inclusion and exclusion
criteria. Among these, 12 articles underwent an in-
depth evaluation, highlighting approaches such as so-
cial interactions and collaborative platforms (25% of
the studies), survey techniques and online question-
naires (33%), as well as probing and rubric-based as-
sessments (17%). Emerging technologies, such as the
metaverse and blockchain, were explored in 8% of the
articles, while 17% recommended combining forma-
tive and summative assessments as an effective eval-
uation practice.
The second review, "Systematic Literature Review
on Artificial Intelligence in the Context of Education
5.0," explored the application of technologies such as
Artificial Intelligence (AI) and the Internet of Drones
(IoD) in educational contexts. Initially, 224 articles
were identified, with 32 pre-selected and 14 analyzed
in detail. Results revealed the growing adoption of
AI to personalize learning and provide individual-
ized feedback. Approximately 21% of the analyzed
studies examined gamification as a motivation and
engagement strategy, while 29% highlighted recom-
mendation systems that tailor content to individual
needs, creating a dynamic and collaborative learning
environment.
Manna Methodology: A Novel Approach to Education 5.0 Through Learning and Socio-Emotional Assessment in IoD Bootcamps
505