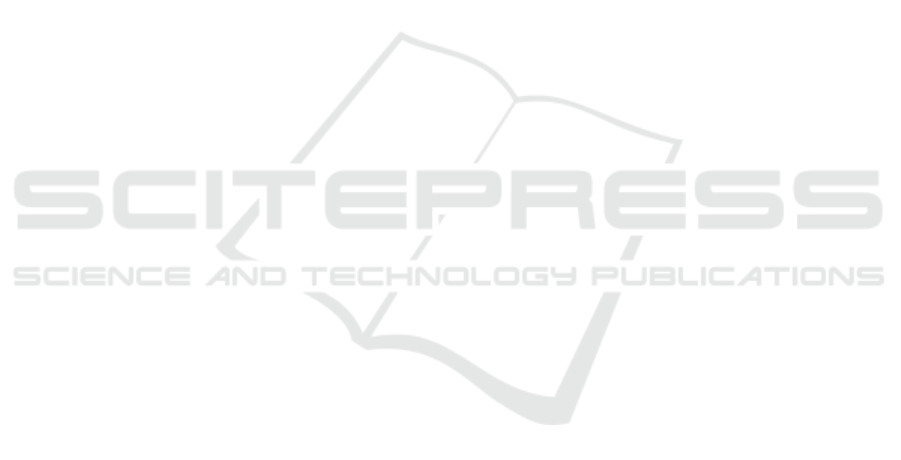
Adaptive Interaction Field Framework for Risk-Aware Navigation of
Driverless Minibus in Pedestrian Zones
Qazi Hamza Jan and Karsten Berns
RPTU Kaiserslautern-Landau, Erwin-Schr
¨
odinger-Straße 52, 67663, Kaiserslautern, Germany
Keywords:
Mapping, Autonomous Driving, Pedestrian Zone, Risk Assessment.
Abstract:
In complex pedestrian zones, the navigation of driverless minibuses faces significant challenges due to vary-
ing environmental structures and pedestrian behavior. These zones range from organized pathways to open,
unstructured spaces with minimal navigational cues. To address this, dynamic interaction fields are developed
around the driverless minibus, adapting in size and shape to real-time movements. To achieve a similar rep-
resentation as vehicle, interaction fields are developed that incorporate pedestrian unawareness. These virtual
fields facilitate safer and more intuitive interactions between vehicles and pedestrians by incorporating real-
time pedestrian awareness and activity data. The proposed model assesses risk by aggregating grid values
from overlapping zones between pedestrians and driverless minibus, computing potential encounters based on
spatial positions and awareness levels. A gradient-based heat map visualizes risk, highlighting areas where
interaction with pedestrians is needed. This adaptive approach enables the decision-making module to initiate
appropriate responses, such as escape maneuvers or interaction mode activation, based on risk thresholds. The
interaction field further classifies risk into ambient, direct, or critical levels, guiding the system’s reactions.
This framework enhances safety protocols and situational awareness in diverse urban environments. The vehi-
cle was able to drive and interaction in a better way with enabled interaction fields. Based on these risk values,
various interaction modules were activated, facilitating meaningful and context-aware interactions with pedes-
trians.
1 INTRODUCTION
The inclusion of driverless minibuses in pedestrian
zones is becoming an exciting area for the au-
tomotive industry and researchers (Jan and Berns,
2023),(Nesheli et al., 2021),(Milan
´
es et al., 2020).
With the expansion of pedestrian zones and narrow
spaces, driverless minibuses offer quick and flexible
transport between buildings in shared spaces. Safety
becomes a primary concern when navigating through
crowds. In one of our previous works (Jan and Berns,
2021), a hardwired safety system was configured to
prevent collisions. This fail-safe system directly en-
abled emergency braking in case of routine malfunc-
tions. To ensure smooth navigation and avoid abrupt
safety braking due to pedestrians’ presence within the
field, risky pedestrians need to be informed about the
vehicle and give way in advance.
Risky pedestrians are defined by their unaware-
ness of the driverless minibus. This unawareness
could stem from several reasons, such as being en-
gaged in conversation, talking/texting on the phone,
or walking in the same direction ahead of the vehicle.
In such cases, it is necessary to interact with pedes-
trians to alert them using various interaction mod-
ules (Jan et al., 2023), (Rasouli and Tsotsos, 2019).
There exists a spectrum of structural variation in
pedestrian zones based on the intended use and level
of pedestrian and vehicle integration. This enforces
different walking and driving behaviors in pedestri-
ans and vehicle, respectively. To understand the riski-
ness of pedestrians around the vehicle, we introduce a
novel method of understanding riskiness of pedestri-
ans in maps by creating virtual dynamic fields around
the vehicle. Such dynamic fields, also known as ve-
hicle interaction fields serve dual purpose, to improve
safety and enable useful interaction between vehicle
and pedestrians. This helped in better driving and
efficient interaction. To understand the riskiness of
pedestrians in the map, their riskiness is presented as
dynamic fields. Next section presents the inspiration
and concept of interaction field for driverless mini.
Section 3 provides the implementation of the concept.
Integrated results from vehicle-pedestrian interaction
382
Jan, Q. H. and Berns, K.
Adaptive Interaction Field Framework for Risk-Aware Navigation of Driverless Minibus in Pedestrian Zones.
DOI: 10.5220/0013221400003941
Paper published under CC license (CC BY-NC-ND 4.0)
In Proceedings of the 11th International Conference on Vehicle Technology and Intelligent Transport Systems (VEHITS 2025), pages 382-389
ISBN: 978-989-758-745-0; ISSN: 2184-495X
Proceedings Copyright © 2025 by SCITEPRESS – Science and Technology Publications, Lda.