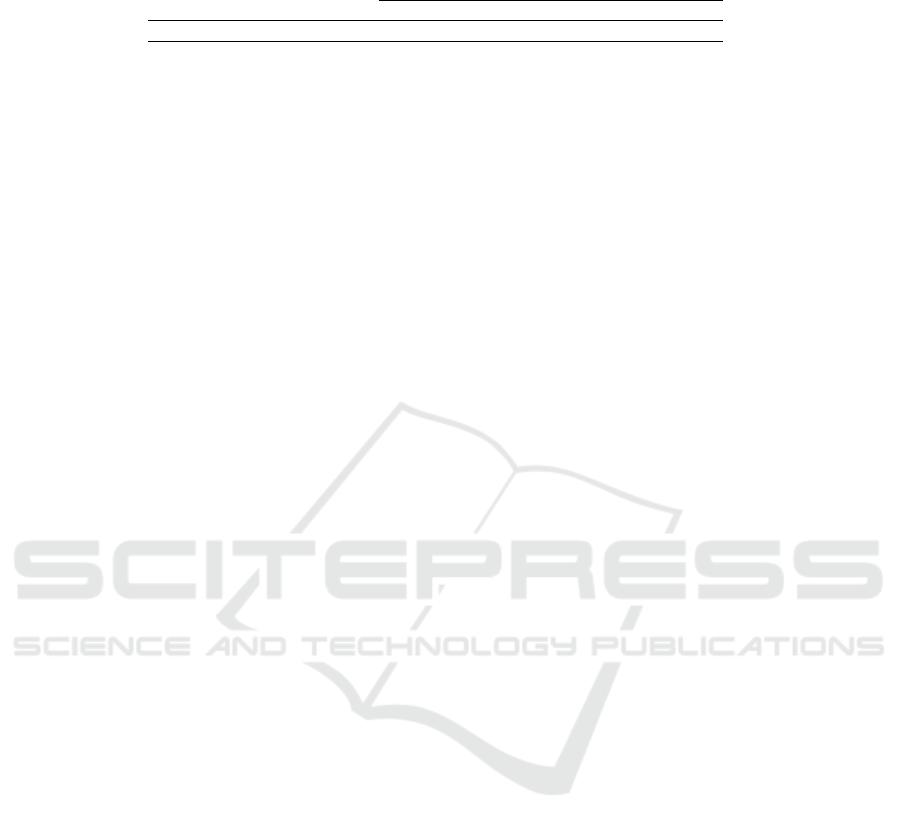
Table 2: Accurary of methods (* is our method), KM is K-means.
Method KM-LSTM
KM-GRU
1 layers 2 layers 3 layers(*) 4 layers
Accuracy (%) 82.45 77.12 86.08 87.11 87.23
Execute time
1.520 1.346
(ms/sample)
5 CONCLUSION
In this paper, we present a novel approach for predict-
ing user movement behavior to facilitate the seamless
handover of User Plane Function (UPF) in network
environments. Initial results validate the effective-
ness of the proposed solution in addressing the key re-
quirements of the problem. However, significant chal-
lenges persist, particularly regarding the deployment
of the solution across the large-scale data generated
by the network.
As the number of base stations increases, the data
quality may degrade, especially in scenarios involv-
ing User Equipment (UE) with low mobility or base
stations with a limited number of active subscribers.
These factors contribute to increased variability and
complexity in the data, potentially impacting the ac-
curacy and efficiency of the solution. Therefore, fur-
ther experimentation and refinements are essential to
optimize the approach and ensure its robustness under
diverse real-world conditions.
REFERENCES
Ariffin, S. H., Abd, N., Ghazali, N. E., et al. (2013). Mobil-
ity prediction via markov model in lte femtocell. Inter-
national Journal of Computer Applications, 65(18).
Cho, K., van Merri
¨
enboer, B., Gulcehre, C., Bahdanau, D.,
Bougares, F., Schwenk, H., and Bengio, Y. (2014).
Learning phrase representations using RNN encoder–
decoder for statistical machine translation. In Pro-
ceedings of the 2014 Conference on Empirical Meth-
ods in Natural Language Processing (EMNLP), pages
1724–1734, Doha, Qatar. Association for Computa-
tional Linguistics.
Cho, K., Van Merrienboer, B., Gulcehre, C., Bahdanau, D.,
Bougares, F., Schwenk, H., and Bengio, Y. (2020).
Learning phrase representations using rnn encoder-
decoder for statistical machine translation. arxiv 2014.
arXiv preprint arXiv:1406.1078.
Faizan, M., Zuhairi, M. F., Ismail, S., and Sultan, S. (2020).
Applications of clustering techniques in data mining:
A comparative study. International Journal of Ad-
vanced Computer Science and Applications, 11(12).
Gou, F. and Wu, J. (2022). Message transmission strategy
based on recurrent neural network and attention mech-
anism in iot system. Journal of Circuits, Systems and
Computers, 31(07):2250126.
Hochreiter, S., Bengio, Y., Frasconi, P., Schmidhuber, J.,
et al. (2001). Gradient flow in recurrent nets: the dif-
ficulty of learning long-term dependencies.
Kakbra, J. (2014). Image classification using k-mean al-
gorithm. International Journal of Emerging Trends &
Technology in Computer Science (IJETTCS), 3:38–41.
Kim, B., Raza, S. M., Yang, H., Challa, R., Le, D.-T.,
Choi, H., Lee, D., Kim, M., and Choo, H. (2022).
ipaas: Intelligent paging as a service. IEEE Network,
37(2):238–245.
Liu, C.-L., Jou, E., and Lee, C.-H. (2010). Analysis and
prediction of trajectories using bayesian network. In
2010 Sixth International Conference on Natural Com-
putation, volume 7, pages 3808–3812. IEEE.
MacQueen, J. (1967). Some methods for classification and
analysis of multivariate observations. In Le Cam,
L. M. and Neyman, J., editors, Proceedings of the fifth
berkeley symposium on mathematical statistics and
probability, pages 281–297. University of California
Press.
Rahamathunnisa, U., Nallakaruppan, M., Anith, A., and
Kumar K.S., S. (2020). Vegetable disease detec-
tion using k-means clustering and svm. In 2020
6th International Conference on Advanced Computing
and Communication Systems (ICACCS), pages 1308–
1311.
Robusto, C. C. (1957). The cosine-haversine formula. The
American Mathematical Monthly, 64(1):38–40.
Schmidhuber, J., Hochreiter, S., et al. (1997). Long short-
term memory. Neural Comput, 9(8):1735–1780.
Subbiah, B. and Christopher, S. C. (2012). Image classi-
fication through integrated k-means algorithm. Inter-
national Journal of Computer Science Issues (IJCSI),
9(2):518.
Xiong, Z., Zhang, Y., Niyato, D., Deng, R., Wang, P., and
Wang, L.-C. (2019). Deep reinforcement learning for
mobile 5g and beyond: Fundamentals, applications,
and challenges. IEEE Vehicular Technology Maga-
zine, 14(2):44–52.
Yan, M., Li, S., Chan, C. A., Shen, Y., and Yu, Y. (2021).
Mobility prediction using a weighted markov model
based on mobile user classification. Sensors, 21(5).
Yin, H., Aryani, A., Petrie, S., Nambissan, A., Astudillo,
A., and Cao, S. (2024). A rapid review of clustering
algorithms. ArXiv, abs/2401.07389.
Zhang, Y., Hu, J., Dong, J., Yuan, Y., Zhou, J., and Shi, J.
(2009). Location prediction model based on bayesian
network theory. In GLOBECOM 2009-2009 IEEE
Global Telecommunications Conference, pages 1–6.
IEEE.
A Learning Approach for User Localization and Movement Prediction with Limited Information
723