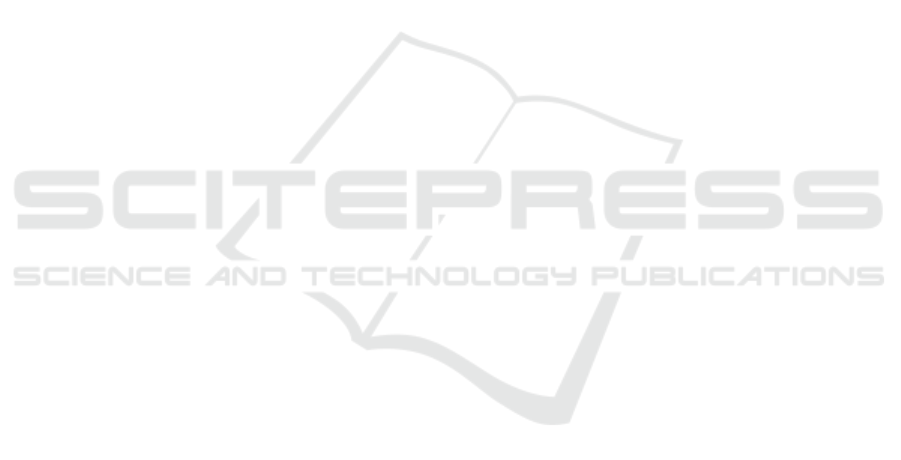
Fushiki, T. (2011). Estimation of prediction error by us-
ing k-fold cross-validation. Statistics and Computing,
21:137–146.
Gao, J., Xiao, C., Wang, Y., Tang, W., Glass, L. M., and
Sun, J. (2020). Stagenet: Stage-aware neural networks
for health risk prediction. In Proceedings of The Web
Conference 2020, pages 530–540.
Giannoula, A., Centeno, E., Mayer, M.-A., Sanz, F., and
Furlong, L. I. (2020). A system-level analysis of
patient disease trajectories based on clinical, phe-
notypic and molecular similarities. Bioinformatics,
37(10):1435–1443.
Goyal, A., Mehta, S. R., Gerstein, H. C., D
´
ıaz, R., Afzal,
R., Xavier, D., Zhu, J., Pais, P., Lisheng, L., Kazmi,
K. A., Zubaid, M., Piegas, L. S., Widimsky, P., Budaj,
A., Avezum, A., and Yusuf, S. (2009). Glucose levels
compared with diabetes history in the risk assessment
of patients with acute myocardial infarction. Ameri-
can Heart Journal, 157(4):763–770.
Ma, L., Gao, J., Wang, Y., Zhang, C., Wang, J., Ruan, W.,
Tang, W., Gao, X., and Ma, X. (2020a). Adacare: Ex-
plainable clinical health status representation learning
via scale-adaptive feature extraction and recalibration.
In Proceedings of the AAAI Conference on Artificial
Intelligence, volume 34, pages 825–832.
Ma, L., Zhang, C., Wang, Y., Ruan, W., Wang, J., Tang, W.,
Ma, X., Gao, X., and Gao, J. (2020b). Concare: Per-
sonalized clinical feature embedding via capturing the
healthcare context. In Proceedings of the AAAI Con-
ference on Artificial Intelligence, volume 34, pages
833–840.
Markov, N., Gao, C. A., Stoeger, T., Pawlowski, A.,
Kang, M., Nannapaneni, P., Grant, R., Rasmussen, L.,
Schneider, D., Starren, J., Wunderink, R., Budinger,
G. S., Misharin, A., Singer, B., and Investigators, N.
S. S. (2023). Script carpediem dataset: demographics,
outcomes, and per-day clinical parameters for criti-
cally ill patients with suspected pneumonia (version
1.1.0).
M
¨
uller, M. (2007). Dynamic time warping. Information
retrieval for music and motion, pages 69–84.
Nakamura, T., Shoji, M., Harigaya, Y., Watanabe, M.,
Hosoda, K., Cheung, T. T., Shaffer, L. M., Golde,
T. E., Younkin, L. H., Younkin, S. G., et al. (1994).
Amyloid β protein levels in cerebrospinal fluid are el-
evated in early-onset alzheimer’s disease. Annals of
Neurology: Official Journal of the American Neuro-
logical Association and the Child Neurology Society,
36(6):903–911.
Reddi, S. J., Kale, S., and Kumar, S. (2019). On the
convergence of adam and beyond. arXiv preprint
arXiv:1904.09237.
Shickel, B., Tighe, P. J., Bihorac, A., and Rashidi, P. (2018).
Deep ehr: A survey of recent advances in deep learn-
ing techniques for electronic health record (ehr) anal-
ysis. IEEE Journal of Biomedical and Health Infor-
matics, 22(5):1589–1604.
Song, H., Rajan, D., Thiagarajan, J., and Spanias, A.
(2018). Attend and diagnose: Clinical time series
analysis using attention models. In Proceedings of the
AAAI conference on artificial intelligence, volume 32.
Strack, B., DeShazo, J. P., Gennings, C., Olmo, J. L., Ven-
tura, S., Cios, K. J., and Clore, J. N. (2014). Impact
of hba1c measurement on hospital readmission rates:
analysis of 70,000 clinical database patient records.
BioMed research international, 2014(1):781670.
Yu, F., Cui, L., Cao, Y., Liu, N., Huang, W., and Xu, Y.
(2022). Similarity-aware collaborative learning for
patient outcome prediction. In International Confer-
ence on Database Systems for Advanced Applications,
pages 407–422. Springer.
Yu, Z., Wang, J., Luo, W., Tse, R., and Pau, G. (2023).
Mpre: Multi-perspective patient representation ex-
tractor for disease prediction. In 2023 IEEE Inter-
national Conference on Data Mining (ICDM), pages
758–767. IEEE.
Yu, Z., Zhang, C., Wang, Y., Tang, W., Wang, J., and Ma,
L. (2024). Predict and interpret health risk using ehr
through typical patients. In ICASSP 2024-2024 IEEE
International Conference on Acoustics, Speech and
Signal Processing (ICASSP), pages 1506–1510. IEEE.
Zhang, C., Gao, X., Ma, L., Wang, Y., Wang, J., and Tang,
W. (2021). Grasp: generic framework for health status
representation learning based on incorporating knowl-
edge from similar patients. In Proceedings of the AAAI
conference on artificial intelligence, volume 35, pages
715–723.
PatSimBoosting: Enhancing Patient Representations for Disease Prediction Through Similarity Analysis
55