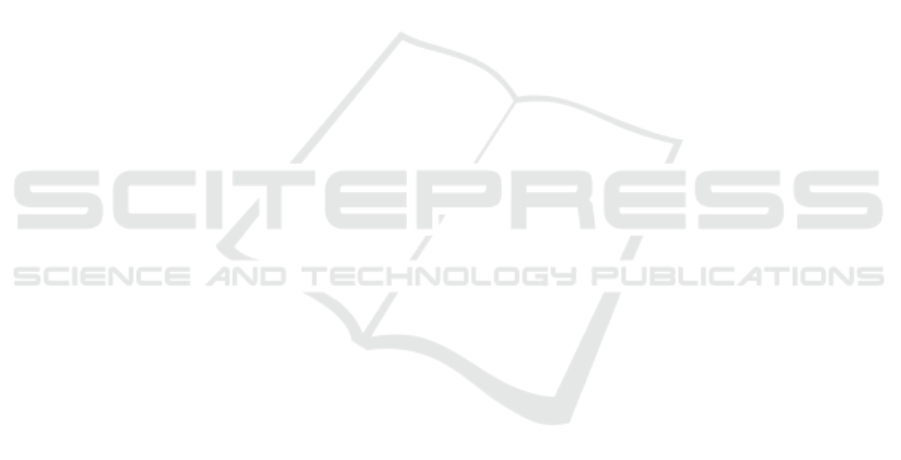
ACKNOWLEDGMENT
Various GenAI Tools were used throughout the writ-
ing process of this paper. Every paragraph was drafted
by the authors and revised with ChatGPT, Claude,
Grammarly, etc.
REFERENCES
Alarc
˜
ao, I. (2017). “dilemmas” of early career researchers
from “dilemmas” to problems.
Ameen, K., Batool, S. H., and Naveed, M. A. (2019). Dif-
ficulties novice lis researchers face while formulating
a research topic. Information Development, 35:592 –
600.
Bhatia, P. (2023). ChatGPT for Academic Writing: A
Game Changer or a Disruptive Tool? . Journal of
Anaesthesiology Clinical Pharmacology.
Bocar, A. C. (2009). Difficulties encountered by the student
– researchers and the effects on their research output.
In Emerging Trends for Sustainability in Global Edu-
cation: Opportuinities and Challenges.
Chatzea, V.-E., Mechili, E. A., Melidoniotis, E., Petrougaki,
E., Nikiforidis, G., Argyriadis, A., and Sifaki-
Pistolla, D. (2022). Recommendations for young re-
searchers on how to better advance their scientific
career: A systematic review. Population Medicine,
4(September):1–17.
Chong, H. N. (2012). Perception and Use of Instructional
Technology: Teacher Candidates as Adopters of Inno-
vation.
Class, B. and De la Higuera, C. (2024). From Disposable
Education to Acting in the World as a Human in the
Time of AI. Journal of Ethics in Higher Education,
(4):231–244.
Cluxton-Corley, V. (2017). The Role of Autoethnography
within Anthropology(How Self Narrative is a Useful
Research Tool in Social Science).
Cohen, L., Manion, L., and Morrison, K. (2018). Research
Methods in Education, 8th ed. Routledge/Taylor &
Francis Group, New York, NY, US.
Cooper, G. (2023). Examining science education in chat-
gpt: An exploratory study of generative artificial in-
telligence. Journal of Science Education and Tech-
nology, 32:444–452.
Eksail, F. A. A. and Afari, E. (2019). Factors affecting
trainee teachers’ intention to use technology: A struc-
tural equation modeling approach. Education and In-
formation Technologies, 25:2681 – 2697.
Elliot, D. L., Baumfield, V., and Reid, K. (2016). Searching
for ‘a third space’: a creative pathway towards interna-
tional PhD students’ academic acculturation. Higher
Education Research & Development, 35:1180 – 1195.
Gloria, A. and Oluwadara, A. (2015). Pre-Service Teachers’
Ease of Use and Intention to Use Selected E-learning
Technologies in Designing Instruction. American
Journal of Educational Research, 3:1320–1323.
Goodfellow, I. J., Pouget-Abadie, J., Mirza, M., Xu, B.,
Warde-Farley, D., Ozair, S., Courville, A. C., and Ben-
gio, Y. (2014). Generative adversarial networks. Com-
munications of the ACM, 63:139 – 144.
Gowda, R. M. (2023). Education 5.0: Evolution of Promis-
ing Digital Technologies – A Comprehensive Review.
International Journal of Advanced Science and Engi-
neering.
Homer, S. R. (2018). Should PhD Students Teach. Psychol-
ogy Teaching Review, 24:77–81.
H
¨
ormann, C., Kuka, L., Fraser, A., and Sabitzer, B. (2024).
Innovation or Imitation? A Critical Analysis of AI-
Authored vs. Human-Authored Scientific Papers. In
CSEDU – 16th International Conference on Com-
puter Supported Education.
Kantcheva, R. and Sum, K. (2023). Getting connected: es-
tablishing direct communication between learning de-
velopers and phd students with teaching responsibili-
ties. Journal of Learning Development in Higher Ed-
ucation.
Kuka, L. and Sabitzer, B. (2024). Navigating Educational
Frontiers in the AI Era: A Teacher’s Autoethnography
on AI-Infused Education. In 16th International Con-
ference on Computer Supported Education.
Kumwenda, S., Niang, E. H. A., Orondo, P. W., William,
P., Oyinlola, L., Bongo, G. N., and Chiwona, B.
(2017). Challenges facing young african scientists in
their research careers: A qualitative exploratory study.
Malawi medical journal : the journal of Medical As-
sociation of Malawi, 29 1:1–4.
Kurian, S., Ramanathan, H. N., and Andrli
´
c, B. (2019). The
Role Internal Marketing in The Promotion of Technol-
ogy Usage Among Teachers: An Empirical Investiga-
tion of the Mediating Role of Extrinsic Factors Lead-
ing to Improved Technology Use. DAAAM Proceed-
ings.
Lalitha, R. V. S. (2021). Ai vs. machine learning vs. deep
learning. In Artificial Intelligence - Recent Trends and
Applications.
Liu, R. (2023). ChatGPT and Other Artificial Intelligence
Applications Speed Up Scientific Writing. Journal of
The Chinese Medical Association .
Tomaszewska, R. (2023). Andragogy Meets ChatGPT
in Lifelong Learning: Exploring Opportunities and
Challenges. 2023 IEEE International Conference on
Data Mining Workshops (ICDMW), pages 868–874.
Wolstenholme, C. (2008). Experience of completing a PhD:
a student’s perspective. Support for Learning, 23:208–
211.
CSEDU 2025 - 17th International Conference on Computer Supported Education
306